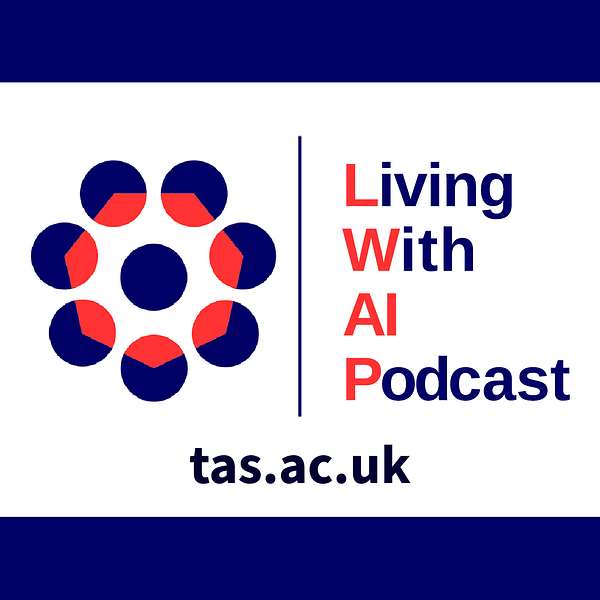
Living With AI Podcast: Challenges of Living with Artificial Intelligence
Living With AI Podcast: Challenges of Living with Artificial Intelligence
Living with AI Security
00:26 Alan Chamberlain
00:38 Paurav Shukla
00:51 Sean Riley
01:45 AI helps this Koda social robot dog sense human emotions (Cnet)
05:08 Classic Moments: Dalek climbs stairs! (Digital Spy)
06:10 WhatsApp to delay new privacy policy amid mass confusion about Facebook data sharing (The Verge)
07:20 Whatsapp Competitor Signal Stops Working Properly as Users Rush to Leave over Privacy Update
10:10 Yes, You Can Stop Using WhatsApp—But Don’t Make This Mistake (Forbes)
13:25 Facebook surveys strip data of friends of friends (wikipedia)
13:50 Professor Neeraj Suri
Podcast production by boardie.com
Podcast Host: Sean Riley
Producer: Louise Male
If you want to get in touch with us here at the Living with AI Podcast, you can visit the TAS Hub website at www.tas.ac.uk where you can also find out more about the Trustworthy Autonomous Systems Hub Living With AI Podcast.
Podcast Host: Sean Riley
The UKRI Trustworthy Autonomous Systems (TAS) Hub Website
Living With AI Podcast: Challenges of Living with Artificial Intelligence
This podcast digs into key issues that arise when building, operating, and using machines and apps that are powered by artificial intelligence. We look at industry, homes and cities. AI is increasingly being used to help optimise our lives, making software and machines faster, more precise, and generally easier to use. However, they also raise concerns when they fail, misuse our data, or are too complex for the users to understand their implications. Set up by the UKRI Trustworthy Autonomous Systems Hub this podcast brings in experts in the field from Industry & Academia to discuss Robots in Space, Driverless Cars, Autonomous Ships, Drones, Covid-19 Track & Trace and much more.
Season: 1, Episode: 10
Living with AI Security
00:26 Alan Chamberlain
00:38 Paurav Shukla
00:51 Sean Riley
01:45 AI helps this Koda social robot dog sense human emotions (Cnet)
05:08 Classic Moments: Dalek climbs stairs! (Digital Spy)
06:10 WhatsApp to delay new privacy policy amid mass confusion about Facebook data sharing (The Verge)
07:20 WhatsApp
10:10 Yes, You Can Stop Using WhatsApp—But Don’t Make This Mistake (Forbes)
13:25 Facebook surveys strip data of friends of friends (wikipedia)
13:50 Professor Neeraj Suri
Podcast production by boardie.com
Podcast Host: Sean Riley
Producer: Louise Male
If you want to get in touch with us here at the Living with AI Podcast, you can visit the TAS Hub website at www.tas.ac.uk where you can also find out more about the Trustworthy Autonomous Systems Hub Living With AI Podcast.
Episode Transcript:
Sean: Hi, and welcome to another episode of Living With AI, the podcast all about artificial intelligence and trust. Today, we're featuring AI security. Very soon, you'll hear from Neeraj Suri, he's chair in cybersecurity at Lancaster University, and he's got a great way of bringing AI trust issues to life, so you're going to enjoy that, I think, today. Before that, let's meet this week's panel. This week, we're joined by Alan Chamberlain and Paurav Shukla. Alan is currently a senior research fellow in the University of Nottingham's Mixed Reality Lab, also known as the MRL, where he mainly works in human-centred design. So welcome to Living With AI, Alan.
Alan: Hi there.
Sean: And Paurav, we see fairly often on this podcast. He's Head of the Department of Digital and Data-Driven Marketing at the Business School in the University of Southampton. Hello, Paurav.
Paurav: Hello, Sean. Wonderful to meet you again.
Sean: Nice to see you again. My name's Sean, Sean Riley, and I'm a video maker or videographer, but try and find that on an AI dropdown of careers. We are recording this on the 4th of February, 2021. Plenty seems to be happening in the world of AI at the moment. I've seen a story about Walmart taking on Amazon at their own game with robot-powered fulfilment centres. I've seen a story about Edinburgh's high-performance computing centre choosing a new AI supercomputer to rapidly accelerate AI research. So AI researching AI, the circle finally complete. hall we start with Alan? Anything caught your eye?
Alan: Yeah, I kind of, some of the work that I've seen coming out of certainly research projects recently has been about how do you make things intelligent that are physical? I've come across this Coda, some kind of, it's an intelligent robot dog. So the idea is that you'd, it's not only a robot, but it's intelligent enough to interact with you. So it's an empathetic dog. So it can recognise when you're sad and it can be sad and it shares experiences. So it's not only sentient, it's aware, but it's sapient.
Sean: I saw this and I, those alarm bells started ringing straight away when I saw it knows when it's sad, happy or excited. In the words of another famous robot, “Danger, Will Robinson.”
Paurav: So very true. But at the same time, isn't that posing a question also, Alan? Because when I'm thinking about these technologies and we have seen ample of them recently, a thing that has come to my mind when I saw this was, is this robot going to understand a Chinese expression the same way as an English or Anglo-Saxon person's impressions and expressions and empathetic views and how far reliable or how reliable this is going to be. So quite an interesting domain in itself when you think about it.
Alan: Yeah, culturally defined trust or, I mean, what do you want your dog to be? I mean, I've got a greyhound. It's very lazy. I wouldn't say it's the most intelligent of creatures. It's not a guard dog. It can run after fluffy things quite quickly. There are certain things I know it can't do, certain things I know it can do, but you could end up with quite a boring animal if it just kind of interacted with it with the same ways over and over again.
Sean: The thing is about this though, we're very lucky because I read a bit further down the article and apparently it is capable of blockchain enabled decentralised AI infrastructure. So come on, the bingo is strong in this one, isn't it?
Alan: But it's not furry though, is it?
Paurav: No, and at the same time, one of the other things I find it quite fascinating is that the dog itself can do so many tasks, but it is very predictable. Your animal is never predictable. There are certain parts of it predictable that you come to a home and your animal jumps at you. That part is predictable. But beyond that, then after what it is going to do? And what you are going to do is so much of that interaction and every day it is like a chess play. It's different every day.
Alan: Yeah, yeah. There's something about ad hoc relationships with people and animals that adds to your life, isn't there, as a human. But I mean, we're able to deal with those kinds of inconsistencies, whereas AI kind of almost wants to go the other way and make everything a uniform, totally efficient process. Whereas what you want is a bit of that rubbing up against, you want your dog to jump up against you, don't you? You want to tell it what to do and you want to try and understand it and engage, I guess.
Sean: I think that there is something telling in the article when it has a real big thing that it makes of the fact that the dog can climb stairs, right? I mean, a Dalek, it is not.
Alan: I thought it looked a bit like a Ferrari.
Sean: It is a nice looking thing. It's like, you can imagine that being one of the kind of things on a Paurav's luxury kind of list of things that perhaps people in a certain kind of tax bracket might choose to try and acquire, shall we say?
Alan: Yeah, you wouldn't call it scruff, would you?
Paurav: No, I don't know. Anyway, somebody may, you know, somebody named, oh, I must not stereotype there. So let's leave it at that. I was just going to say.
Alan: Let's step away from that one right now.
Paurav: I was going to say somebody named Penelope could call it scruff, so no, we don't want to get into that.
Alan: Once they alight from their pink Rolls Royce, yes. Anyway, anything else? Paurav, has anything caught your eye this week?
Paurav: Well, one of the things which has become quite a thing across the world is this new announcement from WhatsApp that has gone around in last month or so. And it has caused a lot of anxiety among its users. And these are, again, AI-driven platforms run by Facebook, wherein, if you remember a few years ago, Facebook paid $19.2 billion to buy WhatsApp and now it has a major problem of how to monetise WhatsApp. This is a key problem. Instagram was bought for about 1.6bn or something and it is monetising so very well. In case of Facebook, that is great. But when it comes to WhatsApp, it is not working out. And suddenly it has come up with this new privacy policy wherein the data will now be shared across the platforms. And this also causes remarkable trust issues.
We have already started seeing the range of that trust issue in leading to the competitors gaining tremendous grounds, including those other companies like Signal and Telegram. Funnily enough, Signal could not even handle the amount of traffic that was being directed towards it. And so that is another very interesting, globally defining trust issue in the technology domain, I think.
Sean: Alan do you use WhatsApp?
Alan: I don’t know, I do use Facebook, though. But what I thought was ironic about it is I just wondered how all these people were sharing their woes about privacy and if they were using WhatsApp to share it in order to move on to different platforms. Because the platform is fantastic for advertising and engendering trust. But if something can engender trust, it can also engender distrust. So if you want to create myths and legends or that kind of thing, you need to broadcast and tell other people. So it sort of becomes a perfect storm, really. It's an advertising platform which peer-to-peers, yeah, so it was just something that I looked at and thought, wow, I mean, there might be something that Paurav can add to this because when something is such a big brand and it is totally honest, does that honesty always work to support your brand or does it mean that when you are honest, people move to another platform that might be doing the same thing but doesn't give you all the details?
Paurav: Yeah, you're absolutely right, Alan. In a way, there are just, but there are so many parameters at play. So one is what we want the companies that we deal with to be honest. For example, I'll take a different example, domain altogether. Think about sustainability, the green consumption, as we call it. And when you think about it, we want those companies to be absolutely honest about their green consumption and want them to be buying sustainability, producing sustainability, retailing sustainability. But when it comes to sustainable consumption and suddenly you see this organic bread available at £2.67 and the non-organic bread available at £1.67, you know where the choices are lying. And so we won't, we are this dodgy characters in marketplace, as I call it.
We show something and we do something different. And so similarly, if a big company like Facebook tries to be honest, we want it to be honest, but when it becomes honest, we also say, oh, you are a dodgy company now, so I'm going to go away. And it's such a funny thing. So we want best of both worlds without compromising on either. And there is not a best of both worlds situation ever.
[00:10:06]
Sean: No. There's one funny thing about the whole WhatsApp thing though, which is that it built a lot of its usership based on this idea of end-to-end encryption. So the idea is not even they can look inside your messages while they're being transmitted, but what that sort of skirts nicely and neatly round is that they've got hold of you at the device end, right? At both ends of that conversation and that's where they're kind of able to have a look at what you're typing, et cetera.
Alan: I suppose what's fascinating about that, it does show you that people are becoming very aware about what the technical infrastructure can or can't do and where the pinch points are, where privacy is happening on the system. Because it's not just your phone, this is like global. And it's just fascinating, isn't it? You think even five years ago, people wouldn't be discussing this, 10 years ago, no way, 20 years ago, I mean, it's kind of, we wouldn't be carrying around a small packet of glass and plastic that let us see anywhere in the world real time and talk with billions of people.
Sean: Yeah. It's rather nice, isn't it? I mean, how quickly this has happened and how slowly we evolve actually and the mismatch there, there is a big mismatch. It's funny because in terms of WhatsApp and people kind of migrating away from it, because I have some contacts that don't use WhatsApp, I use various devices and various messaging systems and I use Signal. And for years, Signal has had this thing where when one of your contacts starts using it, it sends you a message to say, “Hey, Bob is now using Signal.” And honestly, the amount of messages I got in the last few weeks, I mean, this is just an anecdotal look at it, but it just keeps popping up with people that I perhaps once, I don't know, bought a car off five years ago who happens to still be on my contacts list. It might come up as a car man is now using Signal. You know, it's quite interesting. It's quite interesting.
Paurav: It certainly is and imagine in about 10 years' time or even in five years' time, you know that robot dog you were talking about, as you come home will tell you the WhatsApp message. This is the latest message. Now that would really freak me out.
Alan: Yeah, well, I thought with this sort of stuff, it's not only, I mean, if you think about it, I mean, what is it of value that we're sharing with each other that people might want? It's like, you know, I'm going to be back late tonight because, or not nowadays, it's like, I'll be in the front room later on, from upstairs after having this discussion. But the way things are going, it might be that that dog we talked about, it might be that Paurav puts his hand on top of it to touch it, it takes his heart rate. It scans the room. I was looking at some of the latest phones and they've got like, they can sort of, you could do a 3D scan of objects and it will store, that's on a small, you know, that's on your iPhone or the latest like Samsung phone. So all that data that we're swapping becomes amazingly personal and, or it becomes amazing- you might have other people's data about their heart rates or.
Sean: Well, yeah, I mean, it's a bit like the whole friends of friends thing on Facebook. You know, it's not so long ago, and it probably still happens in one way or another, but that we see things like the surveys that are able to strip data not only from you, but from also friends of yours. And therefore that's exactly it, isn't it? You know, we are entrusting a lot of our health, our personal information to these devices, be it from passwords through to, as you say, heart rate monitors and various kind of like health data.
This week's feature is AI security and we're lucky to be able to speak to Neeraj Suri. He holds distinguished Professorship and Chair in Cybersecurity at Lancaster University, where he co-directs the University-wide Security Institute. He's previously worked at Allied Signal, Honeywell Research, Boston University, as well as holding positions at global corporations across the world. Well, welcome to Living with AI.
Neeraj: Thank you.
Sean: Well, your research interests include cybersecurity, trustworthy cloud systems and software. The cloud is a blanket term for kind of multiple technologies. How do we quantify trust when it comes to things like cloud technologies?
Neeraj: So first of all, cloud is that amorphous blob of computing things that you have no idea what they are, where they are, how they work or the other. So let's leave the cloud alone. Let's simply talk about trust. It's the same thing as trusting a human being. What's your notion of trusting someone? Most of the time, the notion is based on, can you depend on person to do something that you expect the person to be doing, right? It's exactly the same thing with your car. It's exactly the same thing with your computer. If something does what you expect it to be doing, you trust it. Now, if all the mechanics of your car are perfectly in order, you will get exactly what you expect out of it.
But let's say the engine is stuttering or someone tries to, let's say, sneak in some water in your gas or something or the other. So something is not exactly as per specification. Now, if your system is designed in a manner that despite any kind of perturbations it is encountering, whether it was, let's say, a design defect, whether it was stress on the system, whether it was load on the system, and switching from a car to the computing world, if you notice, or someone has tried to attack your system or the other. Again, you don't know the mechanics of the thing that is providing you the service. All you care is it continues to give you the service that you're expecting it to do.
So now the question becomes, how do we go about quantifying what trust is? Do we measure? So, like for a car, you have horsepower or something, and there's a frame of measurement. For a computer chip, there is something called millions of instructions per second. Now, trust is one of those very fuzzy kind of entity where you say, so what exactly am I trusting it? Am I trusting it for the duration of an activity? So let me again switch context. Supposing you're flying in a plane, all right? It's an eight-hour flight, 10-hour flight, or some flights which are an hour feel like a 10-hour flight. But basically for the duration of that service that you're expecting from the plane, you do not expect it to do anything out of the ordinary.
So your trust in this particular case is defined for a certain duration. Now, there could be lightning, there could be thunderstorms, anything of those kinds of things. You really would not expect your plane to go down, right? Whether it's one single flight or thousands of flights or millions of flights or the other. So again, you can define your trust for a certain duration, or you can define your trust by saying, there's a certain computer programme or your toaster or your car or something, which will repeatedly keep on doing exactly what you expect it to do. That could be over a much longer duration of time.
Then you can define different notions of trust. So if you say, when your car is brand new, it behaves in a certain manner. But if the car is a few years old, it may not be as performant as you like, but it still does the job and delivers you. So there are all shades of notions of what you call trust. And again, I'll come back to human behaviour. Whatever your notion of trusting another human being or a service or a facility or something, exactly the same thing works in a cloud or a computing system. That's perhaps a longer answer than what you wanted.
Sean: No, it's fantastic. It's really good because it starts to sort of touch on the idea of people's experience. Because like you said, if I've booked something online, first time I do it, I might not be sure that it's ever going to happen and did my booking go through and did it work? And yet my experience of doing that again and again shows me that I can start to trust something.
Neeraj: Exactly. It's repeatable behaviour where you can put your trust into, so Amazon or a hotel or an airline has reviews of the other based on repeatability of experience. So think of that as trust. That's the easiest way to figure it out.
Sean: I suppose the question comes to the idea of this black box. So nowadays a car, maybe go back a few years, you might be able to open the bonnet and twiddle with some screws and things and hopefully maybe it'll start working better. But nowadays you can't, can you? There are lots of computer systems in a car and the same thing is happening online, isn't it? We can't necessarily go and tweak the settings and check what's going on.
Neeraj: Exactly. So when you asked about the cloud, although I work in cloud computing, I really have no idea exactly what combination of services is going to deliver what I'm expecting it to do. It could be physically located in the US or in Europe or somewhere or the other. A, you don't care. B, you don't want to care. All you really care is there's an interface which provides you with a service, as long as you're getting the service out of it, that's good enough.
Now, you mentioned a black box. Now, when it comes to things like AI, AI is even a bigger black box because you don't even know the processes that are executing behind it that is giving you the service. But again, it's basically forget about the infrastructure, forget about the techniques. You're expecting a certain service. You want it in a reproducible, reliable manner and that's what you care about and that defines trust. And of course, you can define all kinds of technical metrics, but it's the notion that is more important than anything else.
Sean: So we're loosely talking around the idea of AI security. In what areas of security is AI playing a role then?
[00:20:05]
Neeraj: So first of all, you need to look at the fact what people call artificial intelligence, all right? Now, what exactly is artificial intelligence? Any set of techniques that either replicate human behaviour and ideally enhance it computationally to be better, faster, all of those kinds of things, is the big definition of artificial intelligence. Now, the aspect of that, so artificial intelligence has two different aspects in it. I'm elaborating because there's a lot of confusion people think what AI is. One part of AI deals with dealing with huge amounts of data and interpreting data, the cognitive replication of the human behaviour, reasoning, decisions, pattern. This class is usually called machine learning and that's the one that we really care about. The second aspect of AI is natural language processing, speech to text translation, so let's not worry about it.
So machine learning, basically, one way of looking at it is by saying, given huge amounts of data, the human eye, the human mind can extract certain patterns out of it and you don't know how it actually does it, right? If we can get AI or machine learning in this case to mimic similar kind of behaviour, that's going to be the context of this conversation, right? So if I say, I'm going to be using AI to, let's say most of the planes that fly right now are more sophisticated than the humans can handle. So there's a phenomenal amount of data that is coming in, which has to be interpreted in a very rapid manner. You cannot react fast enough as a human.
So the machine learning process or AI, I'm going to use them synonymously from this point on, is basically dealing with huge volumes of data. You have given it a rough parameter of what you consider to be acceptable behaviour and the machine learning process, again, black box in this particular case, is delivering what you expect it to do, all right? So now let me come to the security aspect, which will apply both to AI and to any other system roughly the same way. In the case of machine learning, there are two basic components, A, whatever processes are executing the machine learning process, they have to be fundamentally correct. And they are running on data, all right?
So basically think of it as a computational engine, which is either analysing, interpreting, predicting something out of data. If I start tweaking your data to start giving you bad data, the system has no clue what it's actually dealing with, all right? So if the system says, I will be looking at five different numbers, I will be adding them, subtracting them, dividing them, and I'm going to do it repeatedly with huge volumes of data. If it is expecting the numbers five, six, seven, eight, nine, and somebody changes it to six, seven, eight, nine, ten, you have just drifted it a little bit off. The system is a mechanical process, it doesn't know if the data is good or bad, right?
So you can compromise a machine learning process by compromising the data it is getting. So this class of security breaches we normally call adversarial machine learning. There's an adversary who's basically going after the fundamental premise of data and bad data means you're going to get bad results, right? The second aspect of security is all these computational processes, no matter how they're defined or the other, need to operate on some computational infrastructure. There has to be a chip or computers or cloud or whatsoever that's executing.
So supposing I say, my machine learning or AI algorithm is rock solid, my data is rock solid, but someone has now compromised your computational devices that are executing the whole thing, that goes haywire. This third nuance, which is considerably more complex is the fact that machine learning is basically replicating human behaviour, all right? So there are two big categories. One is called supervised machine learning, where you basically like telling to a child or an apprentice, where you show them something and say, “Do this and keep doing it faster and faster.” So you have supervised, you've given someone a frame of reference and then they're repeating it. Here, when there is some distortion or some disruption, it's fairly easy to see.
The second one, which becomes much more complicated, whether it's for planes or autonomous cars or anything on the web whatsoever, is the unsupervised learning, where you basically tell the machine or the algorithm, “This is the data that is coming in. These are the rough parameters.” So you tell you're flying a plane, therefore something vertical up or vertical down is probably a bad thing. But within general parameters, you basically ask the system to interpret patterns and then learn for itself, all right? So you start with slow data, then faster data, complex data or the other.
The problem out here is you have asked a system or an algorithm to now interpret patterns for the other. If someone starts skewing the data or starts skewing the environment or the other, you're basically going to end up having a system which is learning something wrong. So if a bad or a malicious actor is now training you the wrong way or giving you data that will cause you to do something, you will end up with a security breach and you have no idea what you've done wrong. So, there are shades of complexities that come. So again, security is not a binary entity, whether you have security or you don't have security, it's a common fallacy people think that way.
So there are many subtleties depending upon the machine learning process, depending upon the machine learning infrastructure, depending upon the context in which you're using machine learning, and it becomes more and more complex. And one thing that turns out to be very interesting is when you introduce humans into the loop with technology and humans, humans are probably the most unpredictable entities or the other. Now, I like my car example a lot. Supposing a car is heading towards you, so you're a pedestrian and a car is heading towards you and an observer will think, “Hey, the pedestrian has seen the car, is going to jump out of the way.” But what if a human just does the opposite thing and mis-reacts and moves in the direction of the car and the accident happened? Is that normal behaviour? No.
But now to expect a computing system which has been given rational parameters to see some irrational behaviour makes it very complex to figure out what is normal, what is not normal. So even the definition of what is trustworthy behaviour or reproducible behaviour changes dramatically because the environment has become very convoluted. I hope you're getting the examples, I use examples very often. I prefer to avoid technology.
Sean: I think examples are the best way to do this because you can think of that situation. On the podcast before we’ve discussed the odd kind of driverless car situation and one of the things we talked about is the idea of does the car decide to, in a horribly contrived example, kill two people or one person, as in if you're driving the car. But to steer away from that there was, pardon the pun, there was another example which was that certain cars weren't taught to avoid animals, for instance. That was an interest in its own right.
Neeraj: You're opening up a gigantic can of problems and this is part of the thing that we are doing in this project. Who gives you the priorities, what is right or wrong? From an ethical viewpoint, from a legal viewpoint, from a liability viewpoint. So again, talking about examples, supposing there's a police drone which is following a bad guy, all right? And you are here, the bad guy is there and you want to take the shortest path and get to the person as close as possible. Unfortunately, getting to that path means you might have to go through a building, all right?
Now the building may have children or it may have human occupants or nothing or the other. Is the collateral damage you're going to do to the building justifiable or is apprehending a bad person more important? Similarly, when there's a driverless car and you hear of the different examples of accidents or the other, you have to figure out priorities. Is it okay to hit one old person or five young children? Is it okay to destroy, let's say, a glass window outside a shop or is it okay to hurt a human being? Who makes those decisions?
Sean: Yeah, this is the thing in a normal, in a non-technology situation, there would be a panel of jurors and a judge making this decision potentially over weeks or months.
Neeraj: So now it's a computing system which has been trained or taught that these are the guidelines, right? If it was, if each and every single scenario could be anticipated out of the infinite number of things, it would not be machine learning, it would be now executing a process. So machine learning also implies that given the rough parameters and the data coming in, you will behave in a predictable manner. But these priorities have to be determined based on anticipating scenarios. Since you cannot anticipate everything out of the sky, guidelines have to be translated. Sometimes people get it right, sometimes people get it wrong.
[00:30:03]
So there's a beautiful example I came across with a friend of mine in the US. So when the driverless car is based, is using radar or lasers or those kinds of things or the other, they're defined according to a certain scenario. So in this particular case, there was a person dressed in the uniform of a clown coming in the street or the other, and it was dressed as a penguin or some other animal or the other. The system cannot interpret at this point whether it's an animal or a human being that you're looking at. What do you do? Is that a scenario that you're going to come across on a regular basis? Or it's Halloween and people are dressed up in different costumes? Things go berserk. And since you cannot anticipate each and every single scenario, that's where the complexity of most of the systems lie, not in normal behaviour, but in whatever you consider to be out of the ordinary.
Sean: Yeah, these outliers are really important and these edge cases. And I think part of the problem is having a plan, isn't it, for the technology to behave in like an emergency situation. But even in kind of, again, non-tech situations, people have different interpretations of what the best thing to do are, don't they?
Neeraj: People have not only an interpretation, but I'm going to go a little bit abstract out here. Every single thing in computing is based on assumptions. So you design the brakes of a car or a train or something based on the fact that it'll be reasonable weather, there won't be too much snow on the ground, there won't be a deer or something or they're jumping. And then you make an assumption there are X number of bad things that can happen at the same time. So instead of saying that there's going to be a single animal out here, I'm going to say there's lightning, there is snow, there's an animal, there is a person trying to cross the rails, your model or your assumptions are no longer valid.
So whenever things go outside the zone of comfort, this is when things start getting very complicated and you have to anticipate the scenarios. You cannot simply say, “This is beyond what we had designed for.” You need to have some guidelines which say, this is the range or the envelope of expected behaviour or good behaviour and anything beyond that, I'm not going to interpret, I'm simply going to consider it to be unacceptable. It's a human problem, it's a very physiological problem to say a better word.
Sean: It is one of those things whereby even in a driving test, for instance, here in the UK, you are, I don't know, told to do certain situations, certain circumstances in your test. But then outside of that test, you're going to encounter all sorts of different things and be expected to hopefully cope with them in the right way and the technology is doing the same thing, I suppose. It's what do you do in those circumstances that counts then, isn't it?
Neeraj: Absolutely and sometimes you don't even know what the circumstances are. So whenever, I mean, I'm a pilot, so very often I read accident cases and by the time they're describing what went wrong in the whole thing, you look at the complexity of the situation. There's actually a fantastic book which is called ‘Normal Accidents’ and it's a book which simply makes the conjecture that things have become so complex that it's a series of, or the classic example is a butterfly in the South Pacific flaps its wings and something changes in the US. So that chain of interactions is what we don't know. But that's what makes life interesting.
Sean: And that's the thing, when we come back to kind of thinking about AI and machine learning and security, there are these, well, I was going to say there are sort of negative connotations, aren't there? How do we get past that? How do we escape this sort of negativity of this?
Neeraj: I think with time you go, so let's say, since the whole discussion started with the notion of trust, right? So if certain technology continuously, repeatedly does what you expect it to do or gives you a normal, or let's say reasonable behaviour or the other, you build a trust in it. There is no other way to do it. So in most of the, I mean, why do you fly? So the first time people sat in a jet plane, you would look out of the window and say, “Gosh, the propellers are missing out here. What do we do?” And then you look at the fact the plane flies, then you look at the fact that it's lightning, thunder or the other, planes do not crash. So you build up trust by using something on a regular basis.
That's the same thing with AI or the other. Of course, there are going to be anomalies. It's the limitation of design, anticipation, usage. And there was a time when you would not trust your mechanical brakes to be replaced by the analogue braking systems. Now you do. There was a time you would not trust your phone to be doing the banking, or you would fear about it being compromised. Now you do. So when things do not happen wrong on a regular basis and the frequency decreases over time, that's the process of building comfort.
Sean: This experience, I think we have discussed on the podcast before. I gave an example where back in the late nineties, I booked a ferry journey online and I was very tentative about whether I was even going to get on the ferry or not. And then 20 years later, nearly everything I book is online. Don't pick up the phone. I think the other side to the trust element though, is though, what are they doing with my data? What's going on in the background? Is that something we need to worry about?
Neeraj: Yes and no. Yes, because it's a natural worry. B, because a lot of people worry about this who need to worry about it and do make sure this kind of checks and bonds do exist. So I work in this area and I started with the same fears of, I mean, there is always an element of common sense. So if there is a choice that is given to you, do you want to share your location, right? One generation will say, who cares? The other generation says, do I have to give up any information that I don't think they need to know about that information?
Now, a lot of these things are based on, let's say bad experiences or the other. Now, let's say you're in a driverless car, all right? The only way it's going to work is if it knows your profile, your location and lots of parameters and lots of information is needed to provide the service. Are you going to share that information or worry about if that information is going to be compromised? Or would you rather have a safe car versus a car which says, “Gee, I didn't have this information. So now I'm going to make a decision based on something else.” So yes, data will get compromised.
There are, in a lot of technology, bad experiences are the one that help shape and improve the technology. It's the same thing with any technology. So I think we will go through this phase of data privacy and hopefully we'll get away from it. But it's a natural worry and it's perfectly natural to worry about it.
Sean: It's one of these standard things, isn't it? In any kind of security, you have to have convenience and security and there's a trade-off or a balance between the two.
Neeraj: It's always a trade-off, security, privacy and convenience. The three of them go hand in hand.
Sean: The triangle.
Neeraj: And you will make your own judgment. I mean, an enterprise will make a different decision. An individual will make a different decision. A younger person, an older person. Sometimes it is based on awareness. Sometimes it is simply based on functionality and they're hard to capture. So one way of thinking that I very often tell my students is, if everything is designed perfectly from day one, that's a bad situation. Because when something bad happens, your system is likely to be very brittle. So it is better to have a few kicks and bruises along the way. You learn better to anticipate what to avoid.
Sean: Professor, it's been really great to talk to you and I've really enjoyed the examples you've used. So thank you for joining us on the Living With AI podcast.
Neeraj: Well, Sean, this has been a pleasure talking to you.
Sean: Well, we discussed all kinds of technologies and all kinds of trust there. And what are your thoughts on this, Paurav?
Paurav: This was an absolutely fascinating talk, Sean. I enjoyed it so much when thinking about it, you know how AI and the world is changing and particularly how Neeraj separated out the world. And in a way, he separated out this issue of AI, not just as an AI mechanism, but a trust as an overpowering mechanism wherein in that cocoon, AI sits, I thought it was very, very fascinating. And the point he brought about in regards to the consistency of performance and that reliability associated with it was really, I think it's the paramount problem in some sense.
It's a great thing, but at the same time, it provides the greatest of challenge for AI researchers and AI practitioners, I think. Because when you think about consistence performance, a machine can always give me the answer as what a mathematics would suggest. I'm a marketing professor, so many a times I tell this to my students, what is two plus two? And obviously the answer would come out to be four or anywhere in the world still on this earth. But in marketing, the answer is, what do your customer want?
[00:40:00]
And I think that is where the key lies is in customer's level of understanding of that consistency is as important as us delivering something consistently.
Sean: I was going to say in graphic design, two plus two is 22, right?
Paurav: Absolutely, can't agree more, can't agree more.
Sean: But that idea of the kind of consistency and it sort of works a little bit on reliability as well, doesn't it? The more that you have experience of something happening when you ask it to, for instance, the more you start to trust it and you use your experience. So I remember having perhaps an old car that I bought second hand and the longer I owned it for, the more journeys I did, which it completed successfully, the more I trusted it, despite it still having had an unknown past for the five years before I bought it. What do you think, Alan?
Alan: No, I think you're both right. I think there were some important points that came out. What I really liked about Neeraj's talk and sort of the way that he presented stuff, it was, for me, it felt as though it was a more of a social science talk. So the idea that, if I go back to Paurav’s idea, it's almost like if you want to build up a relationship with something, and yours as well, Sean, you kind of have to spend time with it. So trust is not built up. I have a go at something. Does it work? Yes, it does. Oh, so therefore I trust it to have another go.
The more, I suppose, time you spend with understanding something, the more you can explain it to other people and yourself and understand what sort of state the system is at the moment. And then you start to sort of build a trust in your product, which you might encourage you to use it again and again. At the same time, you might feel really comfortable using something that doesn't work and is really sort of clunky, but is cool. All your friends use it and you're comfortable using it, and that might override trust because it might be really useful to you and you have to use it because everybody else does. But it might be, you might not trust it.
So it's, yeah, it's an amazingly complex set of variables that you're trying to understand when it comes to usefulness, usability, efficiency, how cool is it, you know? And then if you've got to pay for it, crikey, that's a whole different set of understandings.
Sean: There's another angle on that as well, though. I remember doing a little bit of work on a, I won't say classic car, but certainly an old car that I needed to do some repairs to and actually feeling less trust in it having done the repairs. Not just because I've done the repairs, but mainly because I knew how many different working parts and possible ways and places it could go wrong. So despite having done the work and some people say, “Oh, I did it myself, so it'll be, you know, everything will be fine.” I was more like, “Oh, I didn't realise quite how many working and moving parts there are in this thing. How many of those could go wrong?”
Paurav: I couldn't agree more, Sean, in terms of what Alan and you are saying is, is that it is not just the trust because it is re-usability as in terms of reconnection with the thing. It's the comfort. Sometimes it is just pure habit. You know, I don't trust the thing, but I'm just habitually doing this. And so it's the habit which is driving me and that's why I'm doing it.
Or it could be related to other variables such as, I know that it is going to go wrong at some point in time, but you know, like, for example, you say, you know, five-year-old car, you know that at some point in time, it's going to go wrong. Something's going to happen, but at the same time still, you happen to just go ahead with it. So trust in that sense is such a loaded expression with so many different permutations and combination that would affect it. And especially with new technologies like AI, I can imagine our distrust towards it would be far higher than our trust in the system itself.
Alan: Yeah, I love that thing there because you know when you, I mean, I work, well, pre-Covid, I'd go out and talk to lots and lots of people about what they do, how they do stuff. So, I, yeah, do lots of field work to try and understand what people actually did. And like all of us, we don't like change. We're creatures of habit. And if we have a sandwich once and we really like it, we might eat that for the next 10 years, you know?
So people really fight against change. So it's, yeah, so it's a very, very complex set of things that you're trying to negotiate when you're showing people new technology, particularly when they don't understand it and particularly when terms like AI are loaded and people think it's about robots and that these things are like sentient beings. And it's just not that at all, is it? It's, a lot of this stuff's about feelings, about habit, about brand, about ownership, usefulness, old cars, shoes that you've been wearing for 10 years that are reliable. It's just, you put all this stuff on top of other things to try and understand it.
Sean: There's also that term, you know, that gets used and bandied around of black box. Okay, so the idea of a black box in theory is that it is something that does a job that you don't have to worry about what's actually doing the job, how it's working, et cetera. So for instance, in an AI trial, it might be that there's a team of people in the next room pretending to be an AI to do the trial. It's effectively a black box. How do you work on getting trust out of something that you're not supposed to know how it works?
Alan: I don't know, in my research lab, we push a little bit to try and do research in the wild. So if you want to understand about how, you know, how penguins exist and what they do, and if you go and look at them in a zoo, they sit in a concrete pond and they get thrown a bit of fish and people look at them, but that's not what they're actually, that's not their behaviour in the wild. So we try and take stuff out there, even quite experimental things that might be artist-led just to try and get some sort of understanding. And I think that's the way the world's going at the moment.
It's important to try and get things taken outside of the lab in order that you can at least get some understanding of the implications that your technology is going to have. I suppose there's ethics and all kinds of stuff thrown into there, aren't there?
Paurav: I remember this wonderful cartoon, Sean. This was by, I don't remember the artist's name, but there were two scientists standing next to a blackboard and it is on one side, you know, humongous number of calculations made. And on the other side, there is an answer and it's like something like answer to life or something. And in between there is a little word and a miracle occurs. And that miracle occurs is the black box. And that is where our problem lies with AI right now. The more I read about it within scientific journals, the more I read about media around AI, this is the key problem to getting AI to be trusted within the marketplace. And that is this black box because neither the operators of that AI nor the user of that AI understand how the AI came to that conclusion.
So we are throwing a lot of things inside. And that's why that pixel hacking and all those kinds of examples have been shown to demonstrate to us that it is still not a perfect thing because we don't understand it. And until we don't understand something, we are not going to be very happy about it. And that's why if you remember, or rather you won't possibly, because neither of us were here on this earth at that point in time, but historians talk about that when horses were being removed from London and cars were being brought in, people have tremendous mistrust of those cars and public vehicles because they were thinking, why? Because I can see this horse. I know what this horse does. I don't know what this engine does.
Slowly they understood that, okay, this engine is like this and so on and so forth. Education happened, trust went up. With AI right now, the problem is, is that even the creators themselves don't know what's inside, what's going on.
Alan: I've spoken to lots of people about this. I'm not a technical person per se, but when you try and explain to people about AI, if you chat to somebody who's very technical, they'll say, “Which AI?” And then they're looking at it in terms of, oh, is it a tool that can predict based on previous understandings? Is there randomisation in there? Is it based on a, I don't know, that something's been learning based on a set of instructions and then can take that and learn about other things.
So it's, I think that mixed in with a healthy dose of IT hype is, makes people start to get a little bit worried because you just, we all know in the tools that we're using now, there's probably some intelligence in there. We're not, we don't know where it is and we don't really understand how it's working, in the same way that we probably don't understand the way that our engine work, engine management system exists or works, sorry, works anymore. It's too complex. So I think there's something that could be taken away and it's from all of this and it's trying to make the system sort of explainable, but also intelligible.
[00:50:14]
So it's kind of, what is it doing? I don't need an explanation in a very basic way. I need to know what the system state is. If I'm working on something in a specific context, how is that part of it? But to kind of throw this discussion back, one thing that I have been thinking more and more and more is maybe it's kind of what we're lacking here is an understanding of the way that us, as people collaborate with intelligent tools.
Sean: Yeah, yeah. I mean, there's a, I've been racking my brains for a parallel for this to try and think about it in a slightly early age that perhaps doesn't go all the way back to horses changing and making way for the combustion engine. And the closest I can get to this is when the first kind of home computers came in in the 1980s. I remember my family decided we were going to go and try, buy one of these computers. It was a huge outlay, very expensive for us at the time. And we went to, I think it was the local Dixon's where clearly the salesperson hadn't actually necessarily gone to the right course or whatever. He'd been used to selling stereos or washing machines or whatever it was. And we went in and we said, “We're interested in this one here.”
Now, experience now tells me about the wide variety of different types of computer. But at the time we go into a shop and there are four, five, six computers on a rack or on a table. And he just picks up the nearest information sheet for the nearest computer and uses it to explain the other computer to us because it's a computer, right? And computers are the new thing and computing is universally a bit like we talk about AI now. Where actually at the time there were eight bit computers, 16 bit computers, 32 bit computers. There was the Dragon, there was the Spectrum, you name it, there were different kinds of things and they worked in different ways, but they were computers.
And I feel like we're at that stage with AI where everybody's going a bit like a branding exercise, “Hey, it's got AI in it, it's powered by AI. Hey, it's running the latest AI.” And actually it's what computers were in the 80s. It's a computer. Hey, this computer generated, it's computer driven. I don't know, Paurav this is kind of a marketing exercise isn’t it?
Paurav: It is in part and that is also a great selling point for many AI companies who have been, their startup values and all that have gone so far up by using certain type of words, AI related words in their brochures and all that because people don't understand it, so valuing that becomes quite a tricky thing. And at the same time, I'm reminded of that last podcast we did, Sean, a fool with a tool is still a fool. And I think that is where it is all, it all falls apart. And right now there are so many AIs, they may be good AIs and there is clear understandability into it, but there are so many other AIs where there may be good things happening, but we don't have any clue why that good thing is happening. And there are quite a few bad AI examples wherein we know how that is being used because we are seeing.
Anyway, yesterday only my wife came to me in the evening and say, “Hey, Paurav, I've got this message from HMRC about tax rebate, we are due a tax rebate.” And I said, “I have never seen HMRC act this fast that we have just submitted three days ago the tax return and they've come back saying we've got tax rebate.” And she's a PhD in languages, but she's still a PhD, so very intelligent person. And so we sat there and we tried to decipher the text and we saw that the text had something, tax rebate dot HMRC dot com. And I said, “Did you see this?” “Oh no, okay.” “Did you see what they were saying, the perfect number as we would be getting? How would they know all those type of things?” And it was just a random text, but if an intelligent person can also be made this gullible at the contextuality of the information, AI can do enough harm also. And that’s why those trust issues would remain.
Alan: If you think about, in a different context, the last time this happened really properly is, and you can see people are trying to mirror this, it's like an industrial revolution. And you get people making calculating machines, based on Lovelace's work and Charles Babbage. But the amount of money you must have needed to make something like that, that you could calculate something in a matter of minutes that could have taken years to do. So it's very like AI in that it's an intelligent, it's adding or extending human capabilities by using this machine.
But at the same time, the AI that we're saying, is available to anybody. So I could be sitting out in the Hebrides on my phone, I could be, I don't know, I could be sitting on Everest. I could be sitting in the Bahamas. I can be rich, I can be poor, I can be intelligent, it doesn’t matter, I still get to use these tools. And that's crazy when you think about the power that you're wielding, particularly if you want to scam people or sell stuff.
Sean: Absolutely. I'd like to thank our panel for taking part in today's recording. So thanks, Paurav.
Paurav: Thank you, Sean, for having me, a reliable partner in this journey on Living With AI.
Sean: I hope you trust me. And thanks, Alan, as well, for joining us today.
Alan: No, thanks a lot, Sean, for being reliable and intelligent.
Sean: We look forward to chatting again soon and we hope to see you soon again on Living With AI.
Sean: If you want to get in touch with us here at the Living With AI podcast, you can visit the TAS website at www.tas.ac.uk, where you can also find out more about the Trustworthy Autonomous Systems Hub. The Living With AI podcast is a production of the Trustworthy Autonomous Systems Hub. Audio engineering was by Boardie Limited and it was presented by me, Sean Riley. Subscribe to us wherever you get your podcasts from and we hope to see you again soon.