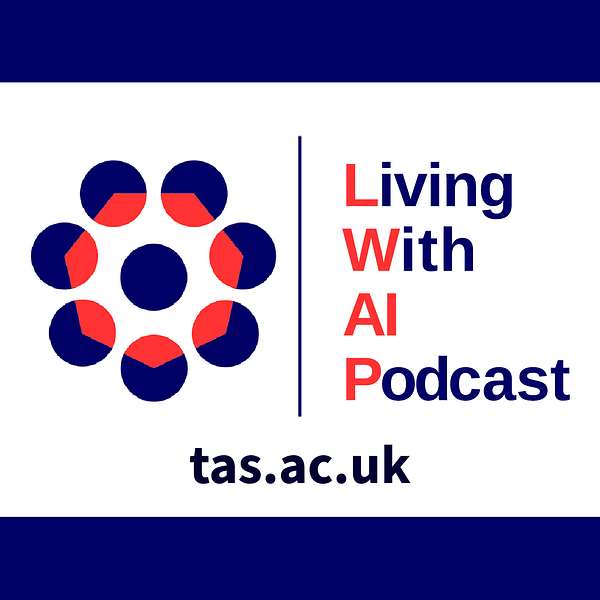
Living With AI Podcast: Challenges of Living with Artificial Intelligence
Living With AI Podcast: Challenges of Living with Artificial Intelligence
The Psychology of Trust
We chat with Professor Thusha Rajendran, Professor of Psychology & Director of the Centre for Applied Behavioural Sciences, Department of Psychology at Heriot-Watt University.
Panel:
Angelo Cangelosi Professor of Machine Learning & Robotics, The University of Manchester, Trust Node Co-I
Dr Pepita Barnard Research Fellow, Horizon Digital Economy, Nottingham
Podcast production by boardie.com
Podcast Host: Sean Riley
Producer: Louise Male
If you want to get in touch with us here at the Living with AI Podcast, you can visit the TAS Hub website at www.tas.ac.uk where you can also find out more about the Trustworthy Autonomous Systems Hub Living With AI Podcast.
Podcast Host: Sean Riley
The UKRI Trustworthy Autonomous Systems (TAS) Hub Website
Living With AI Podcast: Challenges of Living with Artificial Intelligence
This podcast digs into key issues that arise when building, operating, and using machines and apps that are powered by artificial intelligence. We look at industry, homes and cities. AI is increasingly being used to help optimise our lives, making software and machines faster, more precise, and generally easier to use. However, they also raise concerns when they fail, misuse our data, or are too complex for the users to understand their implications. Set up by the UKRI Trustworthy Autonomous Systems Hub this podcast brings in experts in the field from Industry & Academia to discuss Robots in Space, Driverless Cars, Autonomous Ships, Drones, Covid-19 Track & Trace and much more.
Season: 2, Episode: 1
The Psychology of Trust
We chat with Professor Thusha Rajendran, Professor of Psychology & Director of the Centre for Applied Behavioural Sciences, Department of Psychology at Heriot-Watt University.
Panel:
Angelo Cangelosi Professor of Machine Learning & Robotics, The University of Manchester, Trust Node Co-I
Dr Pepita Barnard Research Fellow, Horizon Digital Economy, Nottingham
Podcast production by boardie.com
Podcast Host: Sean Riley
Producer: Louise Male
If you want to get in touch with us here at the Living with AI Podcast, you can visit the TAS Hub website at www.tas.ac.uk where you can also find out more about the Trustworthy Autonomous Systems Hub Living With AI Podcast.
Episode Transcript:
Sean: Welcome to Living With AI, a podcast where we get together to look at how artificial intelligence is changing our lives, altering society, changing personal freedom and what impact it has on our general wellbeing. This week we're talking about trust itself. I'm Sean Riley, I make videos for Computerphile, the computer science YouTube channel but I'm also the host here on Living With AI and shortly we'll hear from Professor Thusha Rajendran, Professor of Developmental Psychology at Hariot Watt University. Before that though here's today's panel. Joining me today are Pepita and Angelo. So if it's okay, if you just to give us your name, rank and serial number?
Pepita: Hi I'm Pepita Barnard and I'm a research fellow at Nottingham's Horizon in computer science and I work with both the TAS and Horizon team.
Sean: Great stuff, and Angelo?
Angelo: Hi I'm Angelo Cangelosi Professor of Robotics and Machine Learning at the University of Manchester and I work on trust in human robot interaction.
Sean: Fantastic thank you both for joining us today, well we're recording this on the 27th of May 2022 so just in case you're listening to this in the future.
I would like to introduce Professor Thusha Rajendran who's Professor of Developmental psychology at Heriot-Watt in Edinburgh. Welcome to the podcast.
Thusha: Thank you very much Sean, pleasure to be here.
Sean: Thusha time for a confession, I'm not very clued up on psychology aside from what little I've read in my puppy training book, but one thing I know has cropped up time and time again in the podcast is the topic of trust and trustworthiness. From, I trust this toaster to make toast and not burn the house down to, I trust this website not to steal my identification and spend a fortune on my credit card, to simply, I trust this process to do what I expect it to do. It means so many different things doesn't it?
Thusha: Yes well in psychology there's different ways of looking at it and the way that I look at it and my colleagues on our TAS node look at it is in terms of what we call theory of mind. So theory of mind for people who don't know it, is basically how we understand other people's mental states based upon their behaviour. And we do this all the time without even realising it and so for example if you see somebody coming into a lift and they're shivering, without even having to think about it you're already wondering, have they forgotten their coat? Are they cold? What's their back story?
We're just hardwired that way to start to look for behaviours based upon that and trust well, there's different ways of looking at trust and the way that we have in our node is to look at this mismatch between what's in the mental representation of the human compared to the representation that's in the artificial agent or the robot. And the interesting thing is that humans have minds so we can kind of disambiguate things, you can ask the person in the lift, “Are you cold, have you forgotten something?”, “What's the matter?” But the robot well what kind of representation do they have? They may be able to give you a, like a pictorial representation or a video snapshot and so this is where there might be this mismatch between what's going on in terms of trust.
The other way theory of mind works is in terms of deception, which is sort of linked to trust and this is where you put a false belief in somebody else's mind. So you might want to suggest that they look in one place whereas you've got your secret stash of whatever it is in somewhere else. And the final way- well not the final way, but another way in which we look at trust is as a personality trait. So in the node we're looking at something called propensity to trust, so is it that some people have more of a baseline to trust than others in a, in a way that some people might have more of a, be more extroverted or more introverted? So there's a, there's a number of, there's a lot of complexity in there which we can tease apart if you like.
Sean: Well yeah I think we're going to, we're going to have to scratch the surface because there's so much to it isn't there? I mean you, you mentioned there the kind of propensity to trust and, and often that sort of thought of, the, thought of like a childlike quality isn't it? Kind of this innocence and, and ability to sort of believe everything you're told, and there's kind of a tendency to think that machines will behave that way. I mean is, is that, is that something that, that gets a lot of kind of study done or research done on it?
Thusha: Not really. I mean I think it's a case of the way that people have researched trust in, in machines is in the, is in the context. So is the machine and authority figure or is the machine a, a companion or is the machine an assistant? So I kind of frame these machines in these three sort of, sort of social categories if you like assistants, companions and authority figures or bosses. And really the research seems to be based upon either of those different categories and also how human like they look. So nobody's really factored in the human, the loop and to say, “Well how much of this variability is to do with individual differences?”
So there's a whole area of psychology that looks at individual differences and the most obvious one is probably IQ. So you can say, “How well did I, did a child do based on this task because they were just smarter or how much it was it to do with their learning?” So you can see how in psychology we try and tease apart these different things to say how much of something is to do with the robot or the context or the personality. So it really is an area that's in need of looking at.
Sean: There's a huge area of human computer interaction already isn't there? Is that something that just needs to evolve into learning you know, incorporating trust into that?
Thusha: Well it's a great question because people have used trust questionnaires but without the psychological background of how we develop these questionnaires. So part of our job as psychologists is to help computer scientists and roboticians not to make the mistakes of psychology, and one of them is to just think, oh I’ve got this idea for a trust questionnaire and I just give it to people. A lot of work goes into creating the items, what we call the items, the individual questions, validating those items, making sure that they're reliable and are stable over a period of time and in HCI and HRI we don't see much of that.
And it would be, the field would improve a lot I think if they took on board some of the real criticism and the real quality assurance that psychologists do to make sure that these traits are stable over time and reliable. Rather than just saying, “We just fire these questionnaires off which we picked off the shelf or made up ourselves.” and so I think it's a case of due diligence I suppose, as well as helping people out to make sure some of the failure to replicate in different studies that you might find in human computer interaction might be simply due to the fact of using different measures.
Sean: And obviously context is king here, but also you know, you've got this old saying beauty is in the eye of the beholder but presumably there are elements of that you know, it depends on the, the specific experiences of the individual that's taking part and how they rate things yeah?
Thusha: Absolutely and this is the core question in psychologies, how much of these things are due to situational factors and how much of these things are due to individual factors? So you might say you know, “Is it due to the fact that you know, is bad behaviour due to a few bad apples?” We've heard that recently, “Or is it the barrels that are rotten?” So if I'm going to put that analogy basically that's what we're trying to do is to tease apart context and individual differences rather than throw our hands up and say, “Oh it depends.” Because you can always say that, but these problems are not you know, intractable if you take a systematic view around this.
Sean: And the other thing of course is that you can obviously, you can change contexts and you can change the, the, the people that you are putting the tests through and the, the feeling with human computer interaction is the system remains a constant. But how does that work when you're using something like AI or machine learning which is itself evolving? Is that something else that really needs to be sort of thought about in these, these sort of testings, testings is that a word?
Thusha: I guess it does but for our purposes we're trying to try and keep that static otherwise it becomes a moving target. So for example if we have some sort of trust scenario, we've got. we've run some studies especially during the pandemic we've had to do them online where we've got people trying to get out of a maze. So there's not a real robot but there's a robot's character and people are primed with robots with different kinds of theory of mind. So they don't have a theory of mind they mimic as if they have a theory of mind.
So we try and keep the maze task static but what we trying then do is vary how they're primed in a video before they're in the maze to see you know, do I trust this robot more if it looks like it has a theory of mind? What happens if it’s just based on behaviour and it just gets me out of the maze, does it really matter if it has a mind? So we, we do our best to try and make sure that the target is not as moving as possible but yes, you're absolutely right, how do you do that? It becomes, once again it has too many variables for us to work out what's going on if you then start to look at changes like that.
Sean: And what's the kind of holy grail of kind of, psychology with related, with, with kind of related to trust in, in this sort of scenario? What, what is what are you hoping to achieve I suppose?
Thusha: So from a psychologist's perspective I'm really interested in looking at dynamic changes. At the moment a lot of the way we understand the mind is static and by static I mean, do you trust somebody at one time point? And it can be binary yes or no but we know that trust develops and so we want to see how it develops, how it's broken, how it's repaired.
So this idea of the development of, of a relationship if you like, it's not really captured very well in psychology. And this is what HRI and HCI offer psychology, this idea of interaction and how we can capture things, because at the moment psychology looks often at very sort of static things and says, “Well I just trust this person yes or no.” Rather than saying, “Well how did we get to this point of trust, how did things break up and how did we get back together again?”
[00:10:18]
Sean: And is trust- maybe this is too difficult a question for me to even phrase never mind answer, is it binary or is it kind of like a spectrum? Do you know, do, is it, do you get kind of like a graph of trust almost?
Thusha: I guess we can look at it in terms of the actual outcome. So one of the things we're keen to look at is behaviour is truth. So people can tell you all, all that they like about whether they want to buy something or a brand or whatever but you have to look at people's behaviours. I don't speed, I don't do this, I don't smoke and then you look at their behaviour and they say, “Oh yeah I smoke.” So often that endpoint is the thing that is the truth and so I suppose we can work backwards from that and say, “What do people tell us about how trusting they are?”
But when it comes down to it what do people do? And one of the key aspects of any experiment is there's got to be some jeopardy I think, and we haven't really put that into it you know, what's the jeopardy of, of not trusting something? Because in real world situations you put your life in somebody else's hands, that has real jeopardy and we do it all the time, we get on a bus, we get on a plane we do it without thinking does that kind of answer your question about that spectrum of trust?
Sean: Yeah it, yeah I think so yeah I, I, I didn't know what I was expecting as I say, I wasn't even sure how to phrase the question. I, I it just occurred to me that you know when people talk about say for instance, autism or something it's a spectrum isn't it? You know people have more tendencies or fewer tendencies or whatever.
I'm just going back again a little bit to the, your theory of mind. You mentioned that in a message to us before we started recording that some researchers call kind of the ability to infer beliefs based on behaviour like mind reading. Do you think that's something that we should be expecting machines to be doing, kind of inferring stuff by you know, looking at a picture or something like this?
Thusha: That would be really impressive if the machine got to be able to do that and that would be I guess a holy grail for AI to be able to do that. One of the things that impresses me most about this work is not necessarily AI, is what it reflects back on to how good humans are at doing it. So how a machine will be able to know whether you're being sarcastic or not or understanding if it was a figure of speech, especially if it was some sort of new term that the young people, the youth of today had coined with.
Sean: What like bad is good, bad is good, sick is good etc.?
Thusha: Yeah a sick you know it's always evolving and so I just don't know how a machine would be able to mind read that and, and the reason why we, sometimes mindreading is used because it's a much more accessible term to the lay public to think, oh you know obviously I'm not literally mind reading. But when somebody tells me something that's sarcastic if I decode what they're saying, I get the wrong message and that's, that's something that we, we know from people with you know development, language conditions or autism for example is that-
Sean: Or, or even just trying to hear, listen to somebody who speaks a different native language. It can, it can be confusing can't it?
Thusha: Exactly.
Sean: I mean these problems as I understand it and having done some stuff on the computer for our YouTube channel about various topics and trying to discuss the idea of the Turin machines and things like this. This idea of a what is it a fruit flies like a banana? You know these ideas are trying to decode this, go back decades don't they?
Thusha: Yeah and it’s, and it's a really, really tricky thing to do. So I think where we have this problem with, with humans or I wouldn’t say it's a problem, an interesting thing is that where there's a human like machine, it looks very you know anthropomorphised you’ve got this propensity in humans to then think this machine has got a mind and treat it as if it's got a mind. And it doesn't we just can't help it, it looks human like-
Sean: Well we do with animals don't we? So never mind-
Thusha: We do it with animals and so we've got this thing that looks like us and we're kind of hardwired socially and so we might have these expectations especially if we're primed like in our study, with a video that suggests it has a mind. And then the machine doesn't have a mind or can't mindread what we're doing it might base its behaviour on us. So you can see where I would- it's almost like a clash of cultures but it's, it's worse than that because when you go to another culture you can kind of default to you know, our basic principles of communication which is pointing.
So if you want something you go to somewhere, you point to it people will generally understand what you're talking about because a lot of our language and communication is bootstrapped on that. Where do you start from with a machine?
Sean: It's like talking to an alien effectively isn't it?
Thusha: Yeah.
Sean: You're part of the TA node on trust right? What, what are you trying to do as part of the TASHub?
Thusha: So we’re really bringing that human element, the human in the loop. So part of what the work we've mentioned is around this sort of development of a gold standard propensity to trust. A set of items which we can then give out you know, both to the, the hub and more generally and then the methodology of why we did this and why it's not a good idea to just cherry pick psychological questionnaires without them being validated so there's a methodological background.
And then there are the experiments, so there's the various trust experiments that we're doing including the mazes task and other ones that we're going to look at. We're going to look at behavioural economics in terms of having a robot and a human and then having some jeopardy, maybe some money or something like that, lots of monetary.
And we want to look at where is this interplay between these individual factors around propensity to trust, how does the robot platform affect it and then what is the context of the interaction, what happens if there's money? What happens if there's real money? So this is the kind of thing we're trying to unpick as well as trying to create a cognitive architecture for trust. So this is the kind of thing that you've alluded to in terms of, what do we put into the machine in order for it to understand. So it’s very ambitious but I think we've got a lot of things to bring to the hub.
Sean: I obviously started it out talking about whether the humans should trust machines and you know whether they can trust them in terms of reliability, whether they can trust them in terms of- what's the word, ripping them off. But there's an, an interesting point here which is, can the machines trust the humans or should the machines trust the humans? Are you doing work around that as well?
Thusha: We would love to do that work and Angelo Cangelosi has done work on this and found that in fact, there are certain context in which the machine absolutely should not trust a human especially if the human is a deceiver. So you can you know, this idea I mentioned before about the false belief? If you, if you give a false answer or put a false answer in the machine should absolutely not trust you so it is bidirectional. We have to do some work based upon that so it may be that over a period of time the, the machine learns that this human is untrustworthy.
Once again this idea of development in much the same way as that you might think that, how do you learn to trust another person you know is it three strikes and you're out, or is it is, is something so good that you can redeem? I mean these things are not weighted equally are they, but this is how we develop. I mean the complexities of our human relationships, we may start off and say, “I believe that I trust everyone equally.”
But that's not really the truth because we might, we'll probably bring our own stereotypes into it, hear somebody speaking in received pronunciation, they may be a little bit older than you the trust dial maybe moves up this way. But it's absolutely bidirectional, I think there are certain circumstances in which, in which machines should not trust humans especially if they are, prove unworthy of trust or they give incorrect information.
Sean: But I suppose there's an element of experience there which kind of works both ways as you know, but an element also of how much experience you have in, in dealing with that character, let's say for instance a fictitious car salesperson who perhaps you know, sells you a dodgy motor let's be really, properly cliched here right? But that-
Thusha: Including sovereign rings and-
Sean: Yeah, yeah sovereign rings, the whole nine yards, hairy chest whatever. The idea of you know, you don't know that person most of the time, the only time you've really had any dealings with them is when they're trying to sell you a car and at that point they're trying to make some money. So they are perhaps dealing with you in a totally different way that they deal with anybody else at any other point in time and it might be the same for the computer, the only time they get to talk to or interact with somebody is when that person wants something?
Thusha: Maybe then that default position is to be trusting because you've got nothing to go back onto. So even, even humans when you go into the used car, already your brain is thinking Delboy, I don't know who other you know, archetypal people mischievous people. We can't help it we've already, we're, we're, we're on all the time so it's, it's- we have a script.
Sean: It's hardwired.
Thusha: Absolutely hardwired. I'll give you a really good example of this, so there's a, there's a bit- you can cut this bit out if you want but there's a bit in Lord of the Rings where- I think it's The Two Towers where one of the- I think one of the, the orcs says, “Meat’s back on the menu boys.” and you kind of think, oh so in the Lord of the Rings world they've got an idea of a restaurant, a maître-d' an order of ordering and everything that comes with that. I'm sure the script writers had no idea that that was implied in that.
[00:20:13]
Sean: Yes, yeah, yeah there wasn't- it was obviously for yeah, for the viewers rather than for the world because I suspect that that yeah that, that that line is in the film but it's probably not in the original book right?
Thuha: So going back to your, your, you know your robot who's got to make, or your AI system that's going to make a decision based upon nothing else it would be, how you set it, whether you set it to just say, “Yeah I trust.” Or, “No I don't trust.” Unless it's with any other information. Whereas humans very rarely don't come into a situation without having some prior information this is, this is why the biases are good and biases are bad we end up you know, stereotyping and all kinds of things.
Sean: It's kind of a survival mechanism isn't it I suppose to a degree isn't it you know? I don't go in and give Delboy £10,000 and walk away with the first car that I see because he's told me it's, it's a right little motor. I maybe haggle or maybe go to more than one dealership or whatever yeah.
Thusha: Theory of mind and lying and deception is part of our psychological defence mechanisms. There's no, there's no morality in this you know you can't say, “Lying is good, being deceptive is bad.” There are times in which you lie in order to save people's feelings you know, “How does my, well my new haircut look?” You know, “What does this dress look like on me? Does my bum look big in this?” You know you, you want to say right thing.
Sean: And people want to appear to know what you want them to know as well right? So there's this element sometimes of you might go for information to somebody who perhaps hasn't got a clue. Let's you know, let's, it's a fictitious person let's really demean them and they might think, well he wants me to say this so they say it right? And you don't get any actual information but you think you've got some information.
Thusha: Yeah and you want to save face so you, so there's- you've already, you're unpacking now like levels of complexity in mindreading theory of mind. So we've had first order, second order, it gets kind of complicated when it gets to third order, he thinks, she thinks, he thinks but we can manage the second order stuff reasonably well. That's how a lot of soap operas work, a lot of books work and how we work. We spend a lot of time thinking about what other people are thinking about whether we admit that or not.
Sean: So we're kind of- I think you know that I think I know that you know that sort of thing, there's a lot of that going on and, and perhaps in the machine world that's too nuanced for that and I know that a lot of the kind of- I know I mentioned machine learning before but I know a lot of that is done by kind of just taking in gigabytes and gigabytes or terabytes just inferring things from that or you know effectively that's, that's what happens. Is there a possibility that some of these machines might be able to sort of just watch human interaction, watch being very loosely and, and sort of come to an understanding from that do you think, or is that quite a long way off?
Thusha: Another great question that I would have wanted to bring up anyway if you hadn't asked it, and it's a question of if you give it enough data will it do it? And there's probably two camps there's one where you give it enough data and eventually it'll do it and another camp that says you give enough data and you're asking the wrong question.
And you've probably worked out that I'm on this side of- because we've, we're at the time where we can actually pump in as much data as you want but it's not going to fix the problem because of our biological system of how we're, we're- you know I mentioned we're on all the time. We're just this you know heuristic, forward planning machine that's always thinking you know, where did this thing you know, this idea of prospective memory come in?
I'm driving along, I’ve got to remember the flowers for my, my wife's birthday you know where did that come from? You know it just came from somewhere, somewhere it's going on and so I don't think no matter how much data you throw at it eventually it's going to be able to do what we can do because we are part of an evolutionary track. So if we look at chimpanzees and bonobos they've got some similarities to us in terms of their ability to rudimentarily mind read. Some people say they've got some pretty good ideas of you know, pretty good abilities of doing that and so we're not just talking about human species we're talking about whole kind of tree of life stuff.
Sean: Crazy and also you know it doesn't matter how much data you have, it's what you do with that data right? If I collected all the data in the universe and had it you know, theoretically in a, on a library shelf I'm not really doing anything with it am I? I could read it all I'd probably forget you know the, the majority of it if I had the time of the lifetime of the universe. Anyway I'm, I'm not sure I'm getting completely off track here or not?
Thusha: No I think there's a- this is why I suppose I would, I would say this but I may be proved wrong but I would say that this is why It's important to have psychologists on board because we provide a sort of a counterpoint to this idea that you can simply solve things by adding more data and simply more maths. I think there's subtleties in this that suggest that it's not going to be that simple and machines are really good you know, give it something to do it'll just do it really well.
But the ability to have a distributed network in the way that human humans have and the idea of sort of connecting with other, other people, and finding the space between mind, so you and I there's a space where our minds are meeting how's that, how's that happening and we're, we're not in the same room?
Sean: Who knows.
Thusha: Who knows?
Sean: Thusha I'd like to say thanks so much for chatting to me today, I really, really enjoyed it.
Thusha: My pleasure Sean. Thank you very much for your questions and our time together.
Sean: One of Tusha's points there which he was quite clear which side of the fence he stood on was this idea of well, if we give it enough data will the system be able to do it? Angelo what are your thoughts on this?
Angelo: So my answer is yes but not the right way, in the sense that it is possible for current machine learning systems like deep learning to show them enough data for the system to discover some regularities which might be related to this concept of theory of mind and trust. But we believe that if you really want to have a cognitive system, a robot that understands the concept of theory of mind you need to go beyond just the statistics and data.
So the approach [s/l where we typically form 00:26:46] is to model different capabilities that we know people use to represent theory of mind, so the first starting point is for example intention reading. I should be able to read your, from your behaviour, from your social gazing, from your verbal descriptions what your intentions are and of course over time I build a representation of your preferences, your desires, your mental state and so on.
Sean: I know Pepi, I know you've been interested in improving HRC is it? Human robot communication I've, I've made that up HRC, HRI that's a thing isn’t it?
Angelo: HRI.
Pepita: And collaboration it, it is the thing.
Sean: Can you tell us a little bit about you know what you've found with this study?
Pepita: One, we have a study that's currently being analysed and one of the things that we did do was to provide three different modes for robots to be in and for people to engage with that robot. And for the first, the first mode was, the robot was continuously improving in its relationship to do a task with the human which was a laundry sorting exercise. And then the, the second mode of robot was, was designed to make an error at certain points with certain items and not to be able to assist in fixing that error and the human would need to kind of intervene and, and deal with it.
And in the third mode we had the robot make the same errors but in this case it was able to show that it could help out and it could make some changes if the human instructed it to do something new. And one of the things that we found was that people tended to prefer obviously, obviously I say now, looking back the third mode where the robot was able to actually you know, interact with them to improve on what it was doing.
But one of the more, more interesting things I think we're discovering from doing that is, what we're trying to develop and it goes back to a bit of what Thusha was saying around pointing. And we were trying to develop these more natural interactions, ways for us to be able to map what humans tend to do as well as what they tend to say. So now what we're looking at while we look through that data are the patterns of behaviour that, that may be apparent in, in what people consistently did.
So nobody did all three modes, they only did one and so we have this opportunity to just look and see how do people respond when something goes wrong? The yes, no, but I meant and some of those are the scripted things that went wrong and some of them are just the natural humans did things wrong in the experiment and you, you get that opportunity there as well.
One of the more interesting things I think about that whole experiment is that it's one of hopefully a series of experiments and what we did initially was, we thought we were creating language for the future Wizard of Oz experiments. So let's create some language through this experiment you know, get people to provoke them to say more, provoke them to get things wrong let's see what we can get out of them.
But we used an actor, a human actor to play the robot and that was fascinating. We put mirrored glasses on them so that the eyes could not be a shortcut so the soul if you like and also what we did find was this uncanny valley was created. We didn't really expect our participants to fall for it as in, that's a robot but they clearly on several occasions did and came out of the room looking at us like, wow you're amazing how did they make this thing? Which is one of three actors that we used, so it's also interesting to see in that kind of hybrid sense where people were uncertain about even were they're dealing with a human or a robot. I find that there's so much more to learn about how people just interact with other when they're uncertain.
[00:30:42]
Angelo: If I can aid something also, I think this shows the importance of designing AI systems or autonomous systems that are not perfect the sense you know, you will normally think, I want an autonomous almost car which is perfect, of course safety is important, I want an AI application in anything which is perfect. But I would say this is not the necessarily the right way to look at it. I want a system which is you know, not better than human, you know it can help humans to do better things better.
But of course we want a system that like humans can fail, can recover and Pepita’s studies clearly show that we are maybe more sympathetic towards other people or robots that make mistakes because we can then understand them for their behaviour, correcting their mistakes, they are, they are really competent you know that demonstrates the full competence.
Pepita: That's right, the calibrating the trust as we go through the process. So the robot’s able to give a, an indicator that I'm not sure of what I should do next and that very action of you know, we can call it dampening trust so they're, they're giving the opportunity for the person to recalibrate their perception of the robot and that builds trust. So even though it's saying, “I don't really know what to do.” It seems to assist.
Sean: I wonder if that's an element of it, it, a character you know, it's seeming to you know, because we all anthropomorphised things right? So you can see that something has let's say is fallible and therefore you go, actually yeah it's, this isn't so far from me maybe- and you start to build I hate to say it, a rapport?
Pepita: Well yeah, infallibility and the vulnerability is important, the ability to be vulnerable with your workmate yeah, it builds trust.
Sean: And the thing is thinking about trust in, in systems like this context is everything isn't it right? I mean we talked Thusha and I talked a little bit about this idea of the Lord of the Rings you know where, where there's an assumed kind of knowledge in a world. And you mentioned it just before about the idea of, okay if worst comes to worst we can kind of point at stuff and we don't have these things in common with, with AI systems. So how do we kind of build a bridge to, to understanding? Is that, is that too much of a cliche I don't know?
Angelo: So one way we can do this is by building robots that have some of the features or human behaviour or human appearance. I'm not suggesting here- I'm suggesting not to build a robot that looks like a human but to build a human robot that shares human features like eyes. So we have a strong tendency to attribute intentionality to other agents even more if these agents have eyes.
So we have demonstrated in studies on human robot interaction and trust, we have two conditions, in one condition the robot is selling objects to a person. After a time the robot changes its mind and proposes a different price, sometimes the higher price. And we measure trust is the propensity to accept the change of mind for the robot.
And we show that if in one condition with a group of participants the robot stares in the void or randomly moves its eyes in another condition the robot once having a dialogue with the participant, looks at the eyes of the participant, at the face of the participants looks at the object being sold and we show that is a strong effect here in trust. That I a social agent, I trust a [unclear 00:34:08] robot rather than an automatic one.
We also demonstrated another interesting phenomenon in this study, the concept of priming. If you prime, if you run a study with the participants is two robots, in one case the robot has a humanoid look or has a social behaviour. So this creates in the participant the expectation that all robots are the same, so when we show afterwards a robot that looks less like a humanoid, more like a mobile robot we showed trust increases when you're being primed with a human like agent.
On the contrary if I let the participants first interact with the robot that doesn't have social capabilities or human like appearances and then I show the same robot which in other groups would result in high trust, this is really at the [unclear 00:34:56]. Which is linked to what again we were saying before, Pepita mentioned this dynamic damping of trust it's, it's a continuous adjustment. We start with a reputation for the person but we adapt this according to the context, their behaviour, the result of the interaction I think.
Sean: There's a, there's another kind of point kind of mentioned there which is that, this idea of prior kind of information. You know we, we kind of make assumptions about people because you look at the age and you think, okay what, what point in life are you at and therefore what experiences might you already have under your belt?
And that's missing isn't it there, from looking at you know, anything from a blank, black box to a humanoid robot to a mobile or a drone or whatever? And I was trying to make it parallel to academia where you know, you don't start a levels until you've already done GCSEs, there's this kind of assumption of a prior acquired knowledge before you approach something. Does, does AI, do AI systems need to start going to school before we can trust them?
Angelo: I guess this is a question for me in the sense this is my area of research. We call it developmental robotics, so the whole epistemological approach to our robot design is that if you want to build a complex system, let's say in language a system they can use the same dictionary 1000’s and 1000’s of words a day as a person and you want these words to be linked to the perceptual motor experience of the robot, we call this grounding. It's not an Alexa system, that there's no understanding of the words just dialogue we are talking about a robot who can physically understand words, who can physically respond to my instruction, “Can you make me a cup of tea by making me a [unclear 00:36:33] physical cup of tea.”
So in this context we have demonstrated that starting at biggest is not a good idea, what we do instead is to have a- we call it curriculum learning or developmental approaches. So we know that in child development the first elements or language development are individual words and then you go through a stage where we combine gestures and words. Before then you can say a sentence with two words automatically. So these are three stages which we know work well in children and we have done experiments to demonstrate it, this is incremental.
What we haven't done really- this is the challenge, is to demonstrate it by starting small you can achieve Alexa type capabilities in a robot in the sense of you know, talking about things that the robot understands. Alexa type in the sense you know, in an unconstrained dictionary it's a very complex task then you know, it will, if it were this easy then it probably wouldn't be an interesting problem, we wouldn't be doing this or animals would have probably found the solution if it were this kind of easy.
Sean: You mentioned Alexa there, do you think people trust Alexa? Is that too, too simplistic question maybe?
Pepita: That's an interesting question do they trust it or do they over trust it? I mean it, it, it probably depends on what you expect from Alexa you know? Like again it's back to the context so if you're expecting to get something particularly intelligent then you are, you are over trusting Alexa. I tend not to trust Alexa very much so you know, I don't use any of these devices at the moment but that's not necessarily because I don't think it can tell me the weather, I'm sure can just I’d rather, rather not rely on these things to, to do and have them all switched on.
Sean: I'd, I've yeah, I've seen lists of my son's search activity through the Google Home app that we have here and, and he'll say four or five different ways of the same thing just to try and get the response he’s looking for. By which point I'm thinking, you could have just got a keyboard, typed this in and found this information a lot, a lot more quickly.
Angelo: Quicker, yeah.
Pepita: I know but again, I think it is, it is something generational probably you know? The moving from modality of finger to keyboard or hand to pen and then, and then it becomes voice and gesture.
Sean: Often a lot of the time if I'm honest, it's multitasking. He's probably watching a YouTube video, playing Minecraft and just shouting out questions to the machine that's also playing him music on a Spotify playlist and you know I, I can't keep track of that.
Pepita: That's going on beyond the wall here behind me. I was interested in Thusha’s point about pointing actually. Only because one of the projects that I was working on, the one I’ve described but also done some literature to look into that area of how to help with computer vision with regards to using natural human behaviours. How to get the machine to be able to you know, identify where you, what you're doing, you're pointing what does that mean and where is that point actually going to with, with regards to the scene that you’re in?
And I found it interesting that you know, you can get devices where you can, can judge the muscle movement. So you can- a wearable wrist device that can do this data gathering for you so I'm interested in actually working with those kind of devices where it can capture the movements of the human as they are happening. And then that can be mapped to something that the AI can learn, learn from and I have seen studies to show that it can. And I question their numbers but it seems to show that it does improve the reliability of, of these models that you know, around computer vision.
[00:40:33]
Sean: Because well pointing itself is quite a difficult thing. I mean animals don't tend to get pointing do they? You know you can a point there and they're looking at the end of your finger thinking, what you know, what's the, what is, what's on there?
Pepita: Exactly yes, yes so we need to teach it something about trajectory where, what, what is that? What, what direction is it coming from and going to if it were to be extended and so on and you know, and funnily enough you know, lots of things seem like they're doable and then we come across problems that seem intractable. Angelo I guess this must be something for you as well? And one of them that we've had recently was around computer vision and being able to tell items, segmentation of fabrics for instance.
So it was all very well saying, “That's a shirt, those are trousers.” It was fairly easy to identify those shapes and, and, and know that you can just fold them up if your robot were to be able to fold and put away. But then when you get to a sock and the robot has to work out- but this is not a thing, it's half of a thing, but it's a whole thing and now I have to find the other thing like this thing, Yeah that, that gets even- we just went, “Oh okay so we can't do socks yet.”
Sean: Is that a question of- I know you’re taking, you know there's a little bit of, a little bit of humour in, in that particular example with relation to, to trust but is there an element here of, what data you need to put into one of these systems? You know, because it's a running theme on the podcast, we always talk about it you know, your training data, the amount of data needs to be kind of properly annotated or you know labelled data it's often called isn't it? Is, is that something we can get beyond or are we always going to need to feed terabytes of data into these systems before they know what they're doing?
Angelo: But at the end of the day we want to go beyond this or we want to go in parallel with this you know, we don't want to say you know, “Data only is not good.” There is a line of research called, one shot learning which focuses on, on how there are some things that we can learn in, in one- with a single experience, single presentation. So there are some methods that look at this and they complement slow you know, learning with lots of data with one shot learning.
I mentioned already the concept of curriculum learning, you can do incremental, acquisitional skills and then there is another element which again counts for the human condition, there is well known paper by Jeff Elman called, “On the Importance of Starting Small.” and it shows in the very simple model, a simple [unclear 00:43:11] neural network which has to learn a series of nested sentences, “I chase the cat that chases the dog, that chases the mouse.” and so on. So in this case you can only achieve complex recursive understanding capabilities if you start small.
Starting small in this concept is not providing short examples, it's actually having a child like memory span which is at the beginning not fully developed, so you can only remember short chunks. So if you start with a network that initially can only learn short chunks even if you present long sentences and then gradually you increase the capability, the memory capability then you get achievement of a complex task.
So two ways to learn complex elements, one is look at mature- we call them maturational, maturational estimate what changes in the structure of the brain, the structure of the learning strategies. The second aspect is curriculum learning, prepare a dataset which is incremental rather than presenting all in one go.
Sean: I'm thinking whether the, that that idea of kind of starting small and building up gives a deeper knowledge or if it just is a different way of fulfilling the same, the same- I've been reading about things like GPT which are these kind of language processing models. Is there a parallel here to, to some of these or is this you know, are they, are those same techniques being used because that's just masses of data isn't it?
Angelo: Yeah I think this is the approach where you only care about the more data the better it is, of course you discover nice regularity, the system can produce a generalised [s/l to novel 00:44:48] the analogies or stories and so on. I was saying in, in a physical, embodied system you need- it's too fast you know, if you start from that it's too far away. You need to get the basics of this robot to understand you know, fundamental concepts, primitive actions, fundamental properties of the world, colours, shapes, weight before you can, you have to worry about complex stuff.
And this is already hard that's why we're working on this, again if you model intuition line of thought these social capabilities in robots starting from reading your intention from observing your behaviour, of observing your social gaze where you look at, it's already something that helps people and robots in these simpler scenarios to handle trustworthy interactions. So trust can build on things of course if we go back to Alexa, if I want to have a full complex conversation with Alexa, instruction for going to a place and routes and navigations then we would expect this to happen I would say later.
Sean: And I think that approach you just talked about there with your colours, you know shapes, all the rest of it I mean it's primary school stuff isn't it? And that seems like those things you need from a robot, what happens if that, those kind of inputs are compromised in some way? I mean you know, we've heard about things like kind of dataset poisoning and stuff like this, is that, is that something that anyone's kind of taking seriously?
Pepita: If the data that goes in is problematic that's just, that's the start of your, your, your road through bias isn’t it? And then you, you do end up with a system that may prefer one group of users over another or some outcome that is not what we would want and that you know, that is problematic actually in, in my opinion.
Sean: Yeah because I'm, I'm thinking you know, we've, we've seen before and are kind of like- this is anecdotal so I can't remember the exact reference but reports that certain AI systems are say, racist for instance or you know, have certain tendencies which could be inferred as racist when it's basically bias in the, in the input info. So when you combine that with some other things we've talked about like you know, driverless cars taking voice input and you know, using their sensors to make certain decisions, these things could really be a problem for trust definitely in some communities.
Pepita: Absolutely, I mean that the one that I can think of, the famous one that was in a recent programme was where the black researcher who was doing an art project could not be seen by the AI until she put a white mask on to her face. And obviously if that was what you were relying on for an autonomous vehicle then there are going to be problems at the crosswalks probably you know? And such like.
So yes it, it, it is critical and it's not just things like that where it's physical danger, it's also about how people's finances get decided by, by systems that make decisions you know, that, that are or you know, basically an AI has had some input. And that, that input may decide that only the males should get jobs for instance because it's, it's used information from only male candidates as one example or you know, or they, or to do with loans and, and ethnicity and such like so-
Sean: If, if we kind of go back to the idea of the kind of primary school education for robot and AI systems then do we think you know, is that is that going to get round some of these bios, biases, bioses- that would be different. Is, do we, do you think there's a way around these biases by not using huge datasets that are potentially tainted and instead using this incremental, very slow- not slow but step-by-step approach?
Angelo: I mean there is research in the field of developmental robotics and there they have a robot nursery school or kindergarten. This area is also called intrinsic motivation, in addition to letting the robot play in a nursery type scenario, learning the basics or school level scenario, you also want the robot to have its own motivation to decide what to learn when to learn. This is intrinsic motivation, I want to learn for the sake of it and for example, there are two methodologies that people use. One is curiosity driven, I want to learn just because I'm curious, so something which is new, I will look at it.
Another one might be competence based, I recognise yellows so I don't really want to learn about yellows, I see another colour and then I ask my teacher or my fellow, “What's that, what colour is that?” So this is happening, smaller scale as I said it's a complex area but of course I would say- this is my, I have to say this is my own field of expertise, developmental approaches are what can lead to more complex capabilities later.
Pepita: I suppose of the things like I just mentioned it briefly, was around the anthropomorphism and the gender element and all the other elements actually, when I thought about it. Because although I had done some research and I was looking It how, how gender gets introduced and how it depends on the context as to how people will accept the, the different, the different genders I then realised, oh it's not just going to be gender is it with a social robot in the future?
It's going to be all sorts of different socio-economic demographic factors that may come into play as to if you think- I think you, you know it was mentioned before about the received pronunciation and imagine what, you know what kind of pronunciation a robot chooses to use may make it more or less accepted for different roles. Is it going to be your boss or assistant or your buddy you know and so on. So I think that it's very interesting to get into the, the minutiae if you like of you know those contextual experiments where people are using things and, and, and, and you're trying to get a sense of, well what are the factors in this particular context that make a difference to acceptance or unacceptance?
[00:50:54]
Sean: Well yeah because I mean if a, if a, if a robot is coming across like you know Jeeves or you know, I forget what the Downton Abbey butler’s called but you know with that kind of an accent then it's perhaps going to be perceived differently. Just to kind of step sideways to sci-fi for, for an example here the robot from Star Wars that's very famous C3PO which is the translation droid. There is a story that says he was originally going to sound like a New York cabby that was going to be the original accent that they were going use for him and then he ended up being this rather quintessentially English, very well spoken character and it just makes me wonder how you know, would he have been perceived very differently? Of course he would if he just sounded like he was from Brooklyn?
Angelo: There is actually research done on accents and trust, so Ilaria Torre, she used to be a student of mine in Plymouth together with my colleague Jeremy Goslin, she did a PhD on accents and trust and now she's a postdoc in Stockholm and this is, she’s changed the topic slightly but this is exactly what she looked at, how accents change trust in robots by also in general, Ilaria Torre.
Pepita: In general ah, I remember hearing something that this the, the Yorkshire accent was very much trusted I thought that was sweet.
Sean: And there'll be, there'll be those underlying biases again though won't there because you know, the no nonsense Yorkshire folk versus-
Pepita: Yeah it's an assumption made based on some stereotypes that we have yeah and there's a lot of projection of the, the stereotype onto people so, “Oh they’re from Yorkshire they’ll be a straight speaking, honest” and then you just make it, go with it don't you? And so you accept it.
Sean: Yeah, yeah but what, but whether that translates across international boundaries is, is another kind of question but then as you know Angelo says there's, there's research on this. So rather than reinvent the wheel what we'll probably do-
Pepita: I’d love to read it.
Sean: -is, is put a link in the show notes to, to that research. I think we've, we've had a fantastic chat there Pepita and Angelo that's really great to have, to have heard your thoughts on, on what Thusha was saying and, and I’d just like to say thank you both for, for sparing your time for us. So thanks for Pepita.
Pepita: Thank you.
Sean: And thank you Angelo.
Angelo: Thank you to both of you very, very interesting afternoon.
Sean: If you want to get in touch with us here at the Living With AI Podcast you can visit the TAS website at www.tas.ac.uk where you can also find out more about the Trustworthy Autonomous Systems Hub. The Living With AI podcast is a production of the Trustworthy Autonomous Systems Hub, Audio engineering was by Boardie Ltd. and it was presented by me Sean Riley.