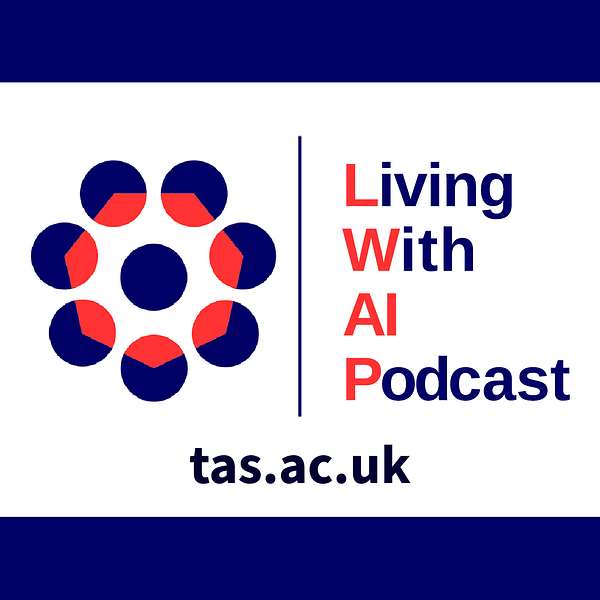
Living With AI Podcast: Challenges of Living with Artificial Intelligence
This podcast digs into key issues that arise when building, operating, and using machines and apps that are powered by artificial intelligence. We look at industry, homes and cities. AI is increasingly being used to help optimise our lives, making software and machines faster, more precise, and generally easier to use. However, they also raise concerns when they fail, misuse our data, or are too complex for the users to understand their implications. Set up by the UKRI Trustworthy Autonomous Systems Hub this podcast brings in experts in the field from Industry & Academia to discuss Robots in Space, Driverless Cars, Autonomous Ships, Drones, Covid-19 Track & Trace and much more. If you have any questions or suggestions for future topics, please email us at contact@tas.ac.uk - You can find out more on our website at www.tas.ac.uk#LWAIP #TASHub
Living With AI Podcast: Challenges of Living with Artificial Intelligence
Trusting AI With Defence - It's Not About Killer Robots
•
Sean Riley
•
Season 2
•
Episode 6
This episode is all about AI & Defence. We've spoken to Ministry of Defence's scientist Steven Meers
Panel Guests:
Professor Jack Stilgoe - Professor of Science and Technology Policy, UCL
Nik Bhutani - Science and Technology Lead - Northrop Grumman UK and Europe
Hector Figueiredo - Head of Technology, UAS Business, QinetiQ
Professor Meers is speaking in a personal capacity. His views do not necessarily represent the views of the Ministry of Defence.
Podcast Host: Sean Riley
The UKRI Trustworthy Autonomous Systems (TAS) Hub Website
Podcast Host: Sean Riley