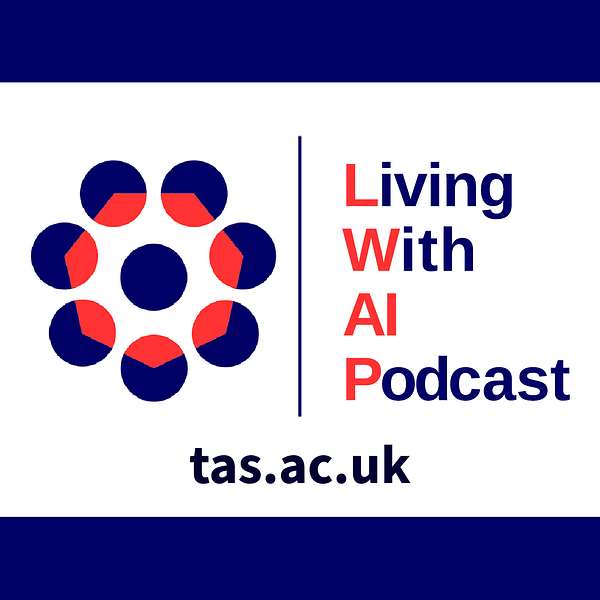
Living With AI Podcast: Challenges of Living with Artificial Intelligence
Living With AI Podcast: Challenges of Living with Artificial Intelligence
Trusting Autonomous Transport (Projects Episode)
Communicating liability in Autonomous Vehicles - Mohammad Naiseh
XHS: eXplainable Human-swarm Systems - Mohammad Soorati
Safety and desirability criteria for AI-controlled aerial drones on construction sites - David Bossens
Source Interaction Interface for Human-Swarm Teaming - Mohammad Soorati
Podcast production by boardie.com
Podcast Host: Sean Riley
Producers: Louise Male and Stacha Hicks
If you want to get in touch with us here at the Living with AI Podcast, you can visit the TAS Hub website at www.tas.ac.uk where you can also find out more about the Trustworthy Autonomous Systems Hub Living With AI Podcast.
Podcast Host: Sean Riley
The UKRI Trustworthy Autonomous Systems (TAS) Hub Website
Living With AI Podcast: Challenges of Living with Artificial Intelligence
This podcast digs into key issues that arise when building, operating, and using machines and apps that are powered by artificial intelligence. We look at industry, homes and cities. AI is increasingly being used to help optimise our lives, making software and machines faster, more precise, and generally easier to use. However, they also raise concerns when they fail, misuse our data, or are too complex for the users to understand their implications. Set up by the UKRI Trustworthy Autonomous Systems Hub this podcast brings in experts in the field from Industry & Academia to discuss Robots in Space, Driverless Cars, Autonomous Ships, Drones, Covid-19 Track & Trace and much more.
Season: 3, Episode: 10
Trusting Autonomous Transport (Projects Episode)
Communicating liability in Autonomous Vehicles - Mohammad Naiseh
XHS: eXplainable Human-swarm Systems - Mohammad Soorati
Safety and desirability criteria for AI-controlled aerial drones on construction sites - David Bossens
Source Interaction Interface for Human-Swarm Teaming - Mohammad Soorati
Podcast production by boardie.com
Podcast Host: Sean Riley
Producers: Louise Male and Stacha Hicks
If you want to get in touch with us here at the Living with AI Podcast, you can visit the TAS Hub website at www.tas.ac.uk where you can also find out more about the Trustworthy Autonomous Systems Hub Living With AI Podcast.
Episode Transcript:
Sean: Welcome to Living With AI. While, artificial intelligence is changing our lives in all kinds of ways, one place AI is constantly in the news is transportation, whether it’s claims of autonomous drones bringing online orders to your door or Johnny Cab autonomous travel systems straight out of the movies, today trust and autonomous transport is our topic. This is season three of the podcast and there are plenty of back episodes for you to go and binge on. I’m sure you’ll be able to find us if you search TAS Hub or you can probably follow some links in our show notes. Today is 12 May as we’re recording this. Particularly with AI moving so fast it’s worth mentioning the date so that you know if you’ve got into your Johnny cab and it doesn’t do what you thought it would do, don’t hold us liable. Liability will come up later, I’m sure in this podcast, but hey. This is one of our project episodes on the podcast where we feature a few TAS Hub projects grouped around a theme and as I’ve mentioned, today’s theme is whether we trust autonomous transport. Each of the project you’re about to hear is related to this in some way. So joining me on this podcast we’ve got three contributors today who I’ll welcome in a moment. Just to set the record straight right at the beginning, we’ve actually got four projects, three contributors and two of our contributors are called Mohammad, so Mo is going to be one of our contributors. So we’ve got Mohammad and Mo. So I’m going to go round the room, just get you to introduce yourselves. So if we could start with Mohammed that would be great. Let us know your name, rank and serial number.
Mohammad: Hi, thank you very much. Thank you for inviting us. I’m very happy to be a part of this podcast. I’m Mohammad Soorati. I’m a lecturer at the University of Southampton school of electronics and computer science. As you mentioned Sean, I’m going to present two projects, one agile and the other pump priming projects that I’d be happy to describe later. My work is mainly around human-swarm interaction and I’d be happy to describe more later.
David: I’m David [s/l Bostons 00:02:03 ], I’m a research fellow at the University of Southampton. I’m running a project on safety and desirability constraints for AI controlled drones on construction sites.
Mo: Hi everyone and thanks Sean for the introduction. I’m Mo Naiseh, I’m a lecturer in AI and data science at Bournemouth University and I will talk today about liability and autonomous vehicles and how actually the general public perceive that concept from their perspective.
Sean: Thank you all for joining us today and actually, we’ll start with you Mo if that’s okay. Tell us about that kind of- It is the kind of like often quoted thing, isn’t it, the liability? Whose fault is it if this goes wrong? Is it the manufacturer? Whoever’s sitting in the vehicle? How did you approach this and tell us a bit about the project.
Mo: Yeah, basically liability in general in autonomous vehicles it refers to legal responsibility of damage and injuries that could happen during the driving, let’s say, journey. As the development of autonomous vehicles now have been- Well it’s beginning to whisper around the world actually, there are a lot of questions now, who should be liable in case of accident. And since, like, if we compare it to the human driven vehicles, there is a clear frame- Legal frameworks and clear understanding from the general public, if an accident happens, we know the human driver who caused that incident will be liable and there is a lot of insurance companies that actually cover a lot of legal aspects. But when we move towards now autonomous vehicles, there are a lot of, let’s say lack of understanding about this area because of, first of all, the lack of understanding coming because of lack of legal frameworks. We still do not have that much of legal frameworks to tell us who is liable in this particular case. And also, another factor that actually, like, contributes to this issue is the understanding from the general public, there is like lack of familiarity, let’s say, still around autonomous vehicles and the results that are coming from our studies and other studies says that the public is still claim to put more liability to the autonomous vehicles in case of an accident compared to similar situations to human drivers. And that has led to a lot of stories about, as I said before, lack of understanding of the capabilities and the limitations of autonomous vehicles that actually make a lot of effort here to make us improve, maybe, the public education around this and help us more in actually putting things in the right way in the future. In our project particularly, we’re looking at how this liability is perceived from the public when we move from low level of automation, because now we have some sort of cars that have some low level of automation like cruise control, stuff like that. When we move from that level of automation to fully autonomous cars, how liability perception could it change when we move from one level to another level. And that’s what we are trying to understand, to help us in the future to shape better maybe legal framewokrs, and maybe shape more public education to actually make it more trustworthy and understandable in the future.
Sean: Thank you Mo, that’s fantastic. I’m just going to ask Mohammad, I’m going to ask which one you want to talk about first. There’s two projects here, I mean, XHS maybe? Because they’re both human-swarm related projects aren’t they? You let us know which one you’re going to talk about first.
Mohammad: Yeah I can talk about XHS first. Yeah. So XHS is about explainable human-swarm systems. A challenge that is hardly understood because we don’t know how to properly explain behaviour of an AI system. So now there’s a lot of trends around ChatGPT and autonomous driving as Mo was explaining, but why are they doing what they’re doing is a huge challenge. We’re looking at a scale which I want to say is more complex, which is around explaining a swarm system and not only a swarm alone but how a human or a group of human operators can work with a large swarm of, let’s say, UAVs, ground robots or groups that are a mixture of these two or many other devices. So this project is focusing first on trying to find ways to be able to explain the swarm. In the past, we’ve worked on understanding the elements that contribute to trust. We went out, we asked the actual operators of robots and told them, hey can you please tell us what is it that contributes to your trust? What is it that makes you gain trust? What is it that makes you lose trust and how can we gain the trust back? Then we try to put that as a foundation to be able to create some rules and then use some formal methods to verify, so basically using formal methods that are like prolistic methods and so on, to be able to really verify that this complex swarm system is consistent with the requirements that would make them trustworthy. Another part we report on is on workload measurement. So if a human operator is using an interface to interact with a swarm, don’t we want to reduce the workload on the operators? Do we want to display everything? Or do we want to just try and customise and find that right subset of information that is relevant for that particular point of time? So the way we described the aim of this project was to formulate it in a way that we want to know what needs to be displayed, how, when and basically why. And in this way, it would be easier to deal with issues such as [unclear 00:09:48] operators or when the operators are not really getting the information they need to make the decisions. So when we put a lot of agents together, we get scalability, flexibility, and robustness. If half of them do not operate, still there are many more agents that could contribute to that. But then the problem is that this collective will not be able to make immediate efficient decisions. It’s hard to reach consensus,as we know in a social society or other occasions where it’s really hard to get the best decision. So the same thing happens when you have a lot of machines working together. So overall, the aim of this project is to put a human next to the swarm of machines or robots and try to create an efficient teaming between the two and answer some of the questions that we need to develop such as this.
[00:10:57]
Sean: That’s a great explanation of that, thank you very much. We’ll go over to David now if that’s okay and hear about using aerial drones on construction sites. Thank you David.
David: So my project is about drones on construction sites. So having autonomous drones there it would give tremendous benefits because they can assist with basic oversight, monitor some faults, transport goods from the ground to the site. Also monitoring trespassers or just the building progress. So to do this in a fully autonomous way is challenging for at least two reasons. So first, the world is incredibly complex and so even when you train your system to appropriately do that, if you put it in the real world there will always be unexpected scenarios and even minor deviations from the expectations can completely disrupt some systems like neural networks and such. So the second problem that is very challenging is that there are actually a lot of behaviours that are not allowed when you put systems in the real world. So these might be simply dangerous behaviours. They might also be violating legal or social norms. So these two problems of on the one hand the robustness to unexpected situations and on the other hand, the various constraints that drones would have to follow, this is the focus of the project. So this project focuses on, on the one hand, the legal aspects of this problem, but also the technical challenges, in particular from the algorithmic point of view. So we’re designing a reinforcement learning algorithm to help cope with this double challenge and then also developing a simulator to incorporate this challenge so that we can actually let an algorithm learn how to solve such a problem. So in this simulator, you give the drone some feedback from the human operator and the human worker. Obviously the construction site is not very complex yet but we hope to increase the complexity and then eventually transfer it into an actual lab environment which mimics the construction site. So that’s a quick intro into my project.
Sean: Fantastic, thank you very much for that. I’m going to throw back over to Mohammad for the other project that you’ve been working on with the human-swarms.
Mohammad: Yes sure. So this second project is the development of an open source interaction interface for the human swarm system that we discussed earlier. So in the project that I’ve already discussed, we deal with some of the fundamental research questions that are involved here. Whereas in this particular project, I’m going to talk about an existing interface that we’ve been developing over the past few years and we made this now open source and this is, I want to claim, the only open source platform that exists that allows a lot of researchers and practitioners in industry to run some online tests of how a small group of human operators can actually interact with large swarms of robots. So we’ve developed this simulator which is an online platform. We hope that soon, we’re going to be able to properly host it on an online service and make it available to everybody to go there and familiarise themselves with the challenges that are there when they want to operate, let’s say, a swarm of UAVs and we’re dealing with a disaster. We live in Southampton, so in an area such as Southampton Common Park where there are people that we suspect might be injured but we’re not sure, so we have a group of let’s say five or ten drones, and the question here is, how can the responder actually try to use these swarms, use this interface and actually get some useful information out of it and have control over the swarm but not really dealing with every single parameter that needs to be tuned for these UAVs in operation. So we actually did a couple of demos already. We’ve been in the AI UK event to demonstrate some of the benefits that we can gain by having a human next to autonomy. So not many people discuss about the fantastic opportunity that is there when you put the human next to the autonomous agent. Everyone wants to say how AI is enough, or what kind of future is AI going to have in the future, but we think there are some aspects that will continue to exist in us humans that will not be there for the AI systems, so we tried to build this interface- So we’re trying to make it understandable. So we’re trying to actually have elements that are displayed that are useful. We tried to make a system scalable, so having three autonomous agents on this platform and 30 does not sacrifice efficiency. We made the system usable and easy to maintain. So a lot of research groups will have some expertise in developing software, so we’re making- Documenting it in a way that everyone can easily create a branch of it and try to maintain and have their specific scenario implemented. So that’s the process we’re doing right now. So another thing I want to mention and then I will stop, is a fantastic opportunity that we had to demo this to children and we gave them this scoreboard of the suspected casualties that were lying on a map and we asked the children to go, deploy these UAVs and really see what is the precision of the outcome and we see how easily they can learn. So they don’t do pretty well in the first round obviously, but in the second round, actually we had participants who actually outperformed our developers. So we really think there is this opportunity to go about this interface to allow people to get a hand on it and don’t have to develop something from scratch themselves.
Sean: It’s a really interesting point actually that all these projects really have something to do with the connection between humans and the autonomous systems. Because from a self-driving car liability, often it’s in- I might have misunderstood this, but it’s often in the changeover between the autonomy and the human control. When you’re talking about construction sites, I’m assuming there are going to be workers underneath the aerial drones. All of these have to do with that, for want of a better word, handshake I suppose between the tech and the humans. Is that a particular challenge? Does anyone want to weigh in on that one?
[00:19:32]
David: All of these projects are actually funded by the Trustworthy Autonomous Systems Hub and we were given these grand challenges of thinking about improving the physical and mental wellbeing rather than harming them or being able to benefit the economy rather than damaging it and so on, so we actually put the human first in the human-centred approach. We actually were asked to put the responsible research and innovation as a core centrepiece of our development and this is why a lot of our projects are around developing a technology that will be driven by the human needs and not just be useful but really have that core usability and trustworthiness at its core.
Sean: And obviously trustworthiness is so key in anything we do with respect to the TAS Hub. I’m thinking about the liability side of things Mo, what is it- Do you think people will trust autonomous vehicles more if they know who’s going to get the blame?
Mo: Yeah actually, and this is very linked to what Mohammad was explaining at the beginning about the human explainable swarm systems. And yeah, of course, like when we have more transparency about how the system works, what is the limitation of autonomous vehicles, who is responsible in case of accidents and in different scenarios. For example, particularly in autonomous systems, we have different types of accident- Well, not accide nt, let’s say risks that could actually lead to an accident. For example, sometimes the accident could happen not because of the manufacturer fault or the driver fault. It could be a cybersecurity attack because autonomous vehicles could be prone to be attacked through the network or something like that and this is actually nobody’s, like, if, say the party’s at fault. There are a lot of challenges to be explainable and transparent in this particular environment, especially in the case of swarm or multi-agent system or multi-vehicles involved on the road, how can you collect all the information in the right time? Because sometimes- And we’ve seen that in- Because I work with Mohammad in this one project, we’ve seen that sometimes if you want to be explainable or transparent, the information is not available at the moment to be explainable or transparent. So you have to collate that information and then provide that explanation after a while and will that be a challenge? Yeah it will. And there’s a lot of research now around this area to be honest.
Sean: Okay. I was also thinking about the construction sites. Obviously, a construction site can be a dangerous place in its own right, David, and then introduced unmanned aerial vehicles, drones to that site, is that going to make that more dangerous? Or is it more that we take some jobs aware that are previously dangerous and replace them with these drones?
David: So on the one hand, you could argue there are safety risks. So yes, the drones need to be careful not to endanger the workers because they need regulations about having a safe distance. But yeah, these regulations are not really modern because they’re not really made for autonomous systems, they’re all based on human controlled drones. But then on the other hand, there are also safety benefits because, for example, it can start with structural monitoring of buildings, and if you have a power plant it’s a very risky environment, so the builder should be careful what they do there. So there are a lot of safety benefits as well. And there are also other human aspects which, I mean, yeah, if you assume that you have these drones there are a lot of other constraints you could consider which relate to humans, so as they were obviously neighbours and such other local residents who don’t necessarily trust you that you don’t want to harm them or record them. So there are some safety, privacy, and wellbeing aspects there as well.
Sean: Projects to do with control policies, where- This is just from a techy point of view, but where does the computation happen for those control policies? Is it similar to this swarm- Well maybe we’ll talk about the swarm control in a moment but just thinking about that, you know, you’re talking about some potential machine learning or whatever to decide how they’re controlled but where does that computation happen? On the device itself?
David: So there are different kinds of approach to this problem. So one would be to take some safe exploration approach which I will not take in this project because in this project we will actually try to learn the policy in simulation. So that means we do have to account for the gaps between the simulator and the actual real world, so that’s why we make our system robust to deviations from the expected dynamics.
Sean: And that’s interesting. Simulation is probably a massive part of anything to do with swarms is it Mohammed?
Mohammed: Yeah, so I think the actual problem we’re facing is that AI by nature is a black box system and we’re all trying to understand what could be the potential outcome of the system. So one thing we do in order to ensure that this safety exists is that we try to calculate all the possible actions of these multiple agents that we have and then really try to draw boundaries around it and say that it is impossible, for instance, for a swarm with such property to exceed this area because of the actual hardware constraints that they have, and therefore try to have a boundary into what could be trustworthy in terms of the risks involved. We do have to have some sort of autonomy on each agent obviously, but then it makes sense to have a central system that tries to actually do a double check of what are the potential outcomes of this system working together and how could they actually be trustworthy. There are also other research that are happening in Southampton, in another school, that they’re looking into for instance the consequences of things going wrong and trying to predict the trajectories of the drones, for instance, falling down and where they could land and try to construct this map. But it isn’t something we really understood as a research community yet and that is why we’re taking this extreme approach that drones are not allowed to fly in this area because it’s just easier this way to say that there’s no possibility that we would allow any drone to fly in this area or at this time because of this concurrent event that is happening. But we hope tht soon, we’ll be able to understand how one or multiple agents can possibly behave and design a system that allows us to be more dynamic and allow more drones to be deployed in real world applications.
Sean: Because that’s how drone control for humans works at this moment, you know? There are certain areas that are completely off limits, various segments around airports and airstrips for instance. But I’m intrigued by what you said about the black box. I mean, obviously, lots of AI systems are black boxes and the only way you can determine what’s going to happen is by running multiple tests is it? I mean, people are using things like ChatGPT for the brains for alternate systems and yet do they even know what’s going to happen under all circumstances? How do we approach that problem?
[00:29:07]
Mohammad: So the issue of- I would hope that Mo can weigh in whenever he thinks I’m on the wrong track here, but the issue of explaining AI, there’s one thing to really try to understand what the system is doing. The other thing is is how you’re trying to explain it to the human. We might be able to actually understand the underlying math that is happening because most of the AI systems that we’re talking about are basically large graph with information that calculates how one information is going to be put together into another and then a logic comes out of it. So we are able to say what’s happening inside. We’re just not able to predict that whether the next output, given this input, is that really the output that we’re going to get? So this is an open area here because if we want to be really designing a system that is fully understandable, fully transparent in terms of being able to predict every single outcome then the AI loses its emergent property as we need to leave it to go wild a little bit and try to really be explorative. But then try to really mitigate the risk by explaining that this is a system under training. So then the human feedback to the outcome could help to reinforce some of the good behaviour and try to eliminate the behaviour that we’re not interested in, but we have to always have that element of exploration. I don’t know if Mo has something to add there.
Mo: Yeah I mean I completely agree with you. As you said, if we have fully, let’s say, rule-based system, there’s also a debate, is that an AI any more because everything is rule based, whereas the AI, as people define it now or talk about it now, it’s more like generative AI or creative AI like ChatGPT or these kind of AI tools that generate pictures from nothing, from text. So explainability issue is another part because when the complexity of the model or of the AI increases, the ability to explain the decision decreases. So there’s like- There’s an issue in explainability. Even the literature around explainable AI now, how people do it, it’s not fully agreeable between all the researchers. Some people still think that the way explainable AI research is moving is not the right way because what people are doing is actually getting the output and getting the input of the model and try to find some sort of a relationship between this input and output. So even explanations that are sometimes generated by other models is not fully truthful to the actual mechanism of AI. It’s more like approximately of what could happen, you know? Even the explanation is not fully complete and truthful to the underlying model.
Sean: Yeah because as you say there’s a tipping point presumably past where trying to keep your head around what the rules are becomes more and more difficult as, for instance, a neural network is involved with multiple nodes, the more nodes that you get the more complicated the decisions are, it’s so difficult isn’t it?
Mo: Yeah it is. That’s why what they call- They try toactually, as I said, approximate explanation not try to explain what actually happened. This explanation might, you know, these factors might have affected the output. But they don’t actually know what exactly happened inside. Even if you explain it, it’s maybe sometimes uninterpretable by the humans. The explanation is too complex to the normal human, or not normal, like non-data scientist, or non-machine learning human can really understand that. So there’s an issue here that actually people who work on a human computer interaction try to mitigate this gap between explainable AI work and how to actually present these explanations to humans in order to may be able to understand it and maybe the explanation that’s related to, for example coming back to the liability part, the explanation that’s generated to the low people is different to the explanation that’s generated to the drivers. A completely different perspective. They want completely different things. And yeah, this is what actually also the new GDPR rules are talking about as well.
Sean: And it’s interesting you mentioned law there because it comes up in a lot of the podcasts we do, you know, legislation, regulation. AI moves so quickly. A lot of things are still being managed under laws that perhaps were designed for a completely different purpose potentially decades ago relating to very, very basic autonomy, maybe autopilot in an aircraft or whatever. How have you approached it? Maybe we should go around the room and see about the different projects, how you approached the regulation side of things? I’m just going to start with David.
David: Yeah, so in our case, we looked at some of the regulations around privacy and safety. So with privacy, actually the main concern would be if you intentionally set out to capture materials from your neighbours which, I mean, that’s not what we would do, but I think this law is maybe a bit too weak because how are you going to actually check that and, yeah, I mean it’s good for us, the expectation is that there aren’t too many hurdles on that. From the safety perspective, the main thing they mention is the distance to the buildings and humans, but these are way to big for any sort of setup that we would consider. But you can always apply for approvals. So- Because there’s no regulations at all, it seems, that are focusing on autonomous drones. They’re all based on basically hand-controlled, like a remote control drone. So for us, this means that primarily we’d have to get case by case approval. So we’re arguing for a more- Like a-
Sean: Yeah because I think the current basic licence allows you to be 50 m away from a residential property when using a handheld controlled device. I think you’re allowed to go 35 m away under certain circumstances. This is my drone knowledge. That’s the limit of my drone knowledge there. Mohammad, how about in the projects you’ve been involved in. How did you approach regulations?
Mohammad: So what we did is we looked at regulations as a subset of the expectations that the swarm has to meet and we looked at it in an abstract way where we said what are the ways to guarantee that we will meet some of the rules, that be regulations, or the operator or public expectation, how can we guarantee that we meet that? So we grouped with a group of researchers in Glasgow where they have looked at verification techniques where we try to actually calculate all the possible outcomes of the swarm and then try to see what are the possibility of the things going wrong or what are the possiiblities of the things actually meeting the criteria put there. So it’s one way of approaching this at the moment. We have not done any hardware tests or no fly outside yet, but we hope that this can be done in the next coming year.
Sean: Regulations is all- Autonomous vehicles have so many regulations right?
Mo: Yeah, so basically our project is, yeah, it touches the regulations and the law from many, many parts but since we’re interested in the public perception of liability, what we’ve done is we’ve connected with people from actually the government and from the Connected and Autonomous Vehicles Department and we had a couple of workshops with them to try and understand what are the main, or the vague scenarios that they really want to maybe understand and to explore from the public perspective. And all we found from this workshop, where we also built our scenarios to do our experiments, we found the shared responsibility in the autonomous vehicles brings a lot of liability issues. Because when you have an AI and a human, there’s an issue of time- The handover time. If the autonomous vehicle, for example won’t be a driver about something and the driver maybe wasn’t really paying attention and stuff, these kind of dynamics and scenarios we’re really interested to explore and discover from also public perspective. Because this will, in the future, bring a lot of implications. How they are going, for example, to shape the regulations and also from a manufacturing perspective. How are they going to price these kind of cars based on the liability assigned to them and the insurance and all these kinds of issues.
[00:40:19]
Sean: Yeah you pay more for a car that’s going to keep you safe in terms of legal reasons as well.
Mo: Yeah, and also will the public be willing to pay that money to actually have this car? It’s another question.
Sean: So it’s been great to hear about all four projects today and I really appreciate you sparing your time to join us, so it only remains for me to go round the room and say thank you to both Mohammads and to David, so thank you very much Mohammad.
Mohammad: Thank you very much for giving us this time and yeah, we hope to come to another podcast with another project.
Sean: Fantastic. Thank you Mo.
Mo: Yeah thank you Sean. I enjoyed listening to all this amazing information and thank you again.
Sean: Great stuff, and thank you David.
David: Yeah, thanks for the discussion and it was a very nice time. Hope you all enjoyed the podcast.
Sean: If you want to get in touch with us here at the Living With AI podcast, you can visit the TAS hub website at tas.ac.uk, where you can also find out more about the Trustworthy Autonomous Systems Hub. The Living With AI podcast is a production of the Trustworthy Autonomous Systems Hub, audio engineering was by Bordie Ltd, our theme music is Weekend in Tatooine by Unicorn Heads and it was presented by me, Sean Riley.
[Silence 00:41:56 to 00:48:48]