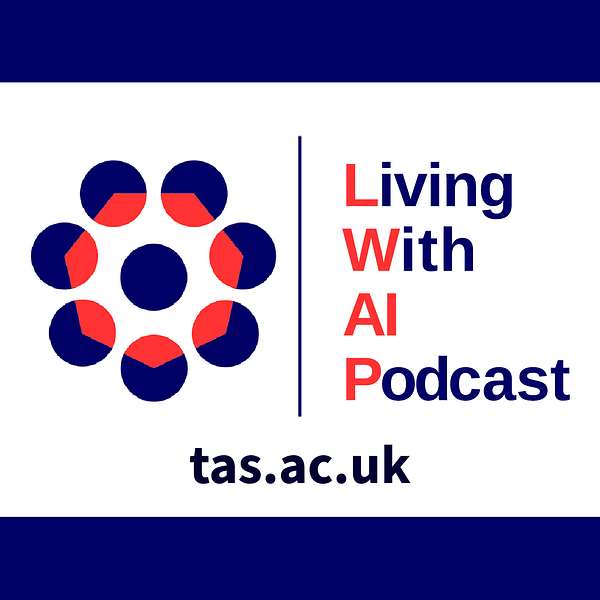
Living With AI Podcast: Challenges of Living with Artificial Intelligence
Living With AI Podcast: Challenges of Living with Artificial Intelligence
AI & Taking Responsibility
A Projects episode focusing on projects dedicated to researching the responsibility aspects of AI: Responsibility Projects – UKRI Trustworthy Autonomous Systems Hub (tas.ac.uk)
Our guests this week are:
1. Lars Kunze - Responsible AI for Long-term Trustworthy Autonomous Systems
2. Shannon Vallor - Making systems Answer
3. Ibrahim Habli - Assuring Responsibility for Trustworthy Autonomous Systems (AR-TAS)
Podcast production by boardie.com
Podcast Host: Sean Riley
Producers: Louise Male and Stacha Hicks
If you want to get in touch with us here at the Living with AI Podcast, you can visit the TAS Hub website at www.tas.ac.uk where you can also find out more about the Trustworthy Autonomous Systems Hub Living With AI Podcast.
Podcast Host: Sean Riley
The UKRI Trustworthy Autonomous Systems (TAS) Hub Website
Living With AI Podcast: Challenges of Living with Artificial Intelligence
This podcast digs into key issues that arise when building, operating, and using machines and apps that are powered by artificial intelligence. We look at industry, homes and cities. AI is increasingly being used to help optimise our lives, making software and machines faster, more precise, and generally easier to use. However, they also raise concerns when they fail, misuse our data, or are too complex for the users to understand their implications. Set up by the UKRI Trustworthy Autonomous Systems Hub this podcast brings in experts in the field from Industry & Academia to discuss Robots in Space, Driverless Cars, Autonomous Ships, Drones, Covid-19 Track & Trace and much more.
Season: 3, Episode: 4
AI & Taking Responsibility
A Projects episode focusing on projects dedicated to researching the responsibility aspects of AI: Responsibility Projects – UKRI Trustworthy Autonomous Systems Hub
Our guests this week are:
1. Lars Kunze - Responsible AI for Long-term Trustworthy Autonomous Systems
2. Shannon Vallor - Making systems Answer
3. Ibrahim Habli - Assuring Responsibility for Trustworthy Autonomous Systems (AR-TAS)
Podcast production by boardie.com
Podcast Host: Sean Riley
Producers: Louise Male and Stacha Hicks
If you want to get in touch with us here at the Living with AI Podcast, you can visit the TAS Hub website at www.tas.ac.uk where you can also find out more about the Trustworthy Autonomous Systems Hub Living With AI Podcast.
Episode Transcript:
Sean: Welcome to Living with AI. Artificial Intelligence is ubiquitous, it is changing our lives in all kinds of ways from Satnav to ChatGPT. This podcast exists to discuss AI with respect to the idea of trust and trustworthiness. We are part of the TAS Hub, that’s the Trustworthy Autonomous Systems Hub and this is season three of Living with AI. So there if you are new to the podcast there is plenty of seasons of episodes for you to discover, just search TAS Hub or check out our show notes and you will be able to find the links there.
Today as we are recording this it’s the 23rd of May 2023, quick check of the computer screen there to make sure I have not misremembered that.
And now it is time to meet some podcast guests. We are doing something a little bit different today, instead of a feature interview this is a set of projects that are all related to responsibility and AI responsibility. So we are going to hear from people involved in responsibility projects.
I am going to ask them all to introduce themselves. So joining us today we have got Lars, Shannon and Ibrahim, so I am just going to go round the room in no particular order. Lars let’s start with you, introduce yourself please?
Lars: Thank you very much for the introduction Sean. My name is Lars Kunze. I am a department lecturer at the Oxford Robotics Institute. I am also the technical lead of the Responsible Technology Institute in Oxford and I am the PI of the RAILS project which is a TAS Hub project on responsibility.
Shannon: I am Shannon Vallor. I am the Baillie Gifford professor in the Ethics of Data and Artificial Intelligence at the University of Edinburgh where I am also Director at the Centre for Technomoral Futures. And I am a PI on the Trustworthy Autonomous Systems Responsibility project called Making Systems Answer, Dialogical Design as a Bridge for Responsibility Gaps in Autonomous Systems. Happy to be here.
Ibrahim: My name is Ibrahim Habli and I am a professor of Safety Protocol Systems at the University of York. I am interested in what it means for complex software intensive systems to be safe, particularly those which incorporate AI and operate at the high levels of autonomy.
For this episode I will present the Assuring Responsibility for Trustworthy Autonomous Systems project AR-TAS on which I am the PI.
Sean: Superb, thank you all for joining us and just sparing your time today to be on the podcast. So we will get a bit more detail on all the projects and I am going to come to you first Ibrahim, just as you take a glass of water, apologies for that. Can you tell us about the Assuring Responsibility for Trustworthy Autonomous Systems project?
Ibrahim: Again thank you of course. So AR-TAS, which is the acronym for this project, chose to explore a very pressing question, posed by the TAS community, as a multidisciplinary community of people of technical and people who are more the social, legal and ethical sides. And importantly a question which is posed by the public at large of who is responsible for the decisions and outcomes of autonomous systems, especially those utilising some AI capability and most importantly why.
So the interesting thing about our project is that it is inherently multidisciplinary. It is a project where technical engineers work closely with safety engineers and of equal importance within the room we have lawyers and philosophers also leading the discussions. So we are posing the question of responsibility from the technical and legal as well as the ethical they mention.
And this is important for our project because we want the important disciplines so they are present in the room, so we have other academics by saying well let’s explore the issue from an ethical and technical perspective. But when it comes to the regulations we leave it to the lawyers. Within our project the lawyer is in the room so we are not leaving them out and we close a number of key holes if you like.
And the way we conceptualise our project was at three levels. The first level is what we call the foundation level, which is do we have a current understanding of what we are talking about? Because as you probably know from other episodes we spend a lot of our time just figuring out that we are talking about the same thing.
So that is why within our project we talk about the foundation level, we don’t have a meta model of responsibility. By this we have this like model of models to describe the different sensors of kinds of responsibility and by this I mean role responsibility, ethic responsibility, moral responsibility as well as the legal side of responsibility, some people refer to it as legal liability.
But also on top of that we do, we try to explore the meaning and the relationships between those kinds as well as the notion of agency, intelligence and autonomy. Again on which we don’t have a consensus of what they mean.
So that is what we are trying to do at the foundational level and we are still going through this exercise and importantly we are trying to deal with it from the different, from the different disciplines and see whether we can just agree on some definitions. So this is the foundation.
On top of that we are building something we are calling Assurance Cases for Responsibility. And the notion of assurance cases comes from work within the safety critical industry on something they call safety cases, which is, if you ask me what is a system mistake I tell you oh it depends, it is a very complicated question. And normally it depends on, well the argument I am presenting and the evidence I am providing, okay. I can’t prove safety in the same way I can’t prove the allocation of responsibility.
So we are borrowing this notion from high risk industry so we can, within multidisciplinary teams and with the public at large, we can reason about who is responsible and why and under what circumstances and how this could change if the world changes. So that is what we call the assurance level.
On top of that level we have the final level which is the more practical level and which is where engineers will tell us like look I don’t have time for all of this, just can you tell me exactly what I need to do? And that is where we are targeting them in terms of we being the output of the research and the method and everything into the design deployment operation and maintenance of autonomous systems. And also weaving those into methods for accident and incident investigations. Because we know inevitably accidents will happen and we have to be prepared for them now rather than after they happen.
And basically that’s the last level if you like within our project. And the last thing to say here is responsibility can be very context sensitive and that’s why we have a number of exciting industrial partners where they can bring the whole discussion to life by working in a healthcare use care, an automotive use case or a manufacturing use case.
So that is why we are challenging ourselves by moving from the comfort of office based discussions to complete use cases on the user of AI in diabetes or when it comes to autonomous driving and the like.
So that is an overall summary of our project.
Sean: Thank you very much. I am going to ask Lars to go next. Lars tell me about the project you have been working on, the Responsible AI for Long Term Trustworthy Autonomous Systems, or RAILS as I understand it to be shortened to?
Lars: In rails we are looking at kind of deploying AI responsibly within the context of trustworthy autonomous systems and we have a particular focus on the long term operation. And the kind of long term operation brings a kind of few challenges, so in particular we are looking at the challenge of change, so this can be two different types of change here we are investigating.
This can be internal change, so that means that the kind of system is evolving over time and so the system itself changes, so either it learns or kind of gathers new evidence. Or there can be external change and that happens in the environment, in the ecosystem around the methods and so this creates another challenge.
And of course related to what Ibrahim said, so kind of we want to make sure that these systems are safe and kind of in particular if they are changing we need to still ensure that they are safe. So the RAILS vision is basically that we provide the kind of technical but also social legal guard rails to have these systems safe and also responsible during their lifetime.
And we are trying to achieve this with three strands mainly, we have kind of core engineering strand where we are looking at the open endedness of environment. So that is kind of one particular challenge we are facing and here we are looking at corner cases in particular in the context of autonomous driving, which is kind of one of the main use cases we are looking at.
And so here we want to extend existing datasets. Because in an open ended world, you cannot really cover everything within your training data so you need new methods for generating new corner cases and also methods for testing and evaluating the system.
So the kind of testing and evaluation is really important so that’s where we bring in simulators. So we are particularly using Comma as a driving simulator and we are also taking part in the upcoming Comma challenge and really trying to extend that and looking into aspects around responsibility for that.
[00:10:02]
And ensuring that these kind of Machine Learning methods act responsibly, we are developing a responsible AI index. So that is kind of measuring different dimensions of these Machine Learning models that can be used, so that measures sustainability for example, we are also looking at transparency or interpretability of these models as well as their robustness.
So designing these index will kind of look at different aspects of these models. For example in terms of sustainability we are looking at the kind of time spent for training, re-training these models and inference time. And so this will have an impact of course on the environment when we are using these models and deploying them.
And so yes this was a very important part which we integrate into this, into the Comma challenge as well later this year. So then this is kind of the engineering work that we are doing.
But then kind of building on top of that kind of another strand looks also at the assurance of the system, this is related, very much related to what Ibrahim said. We are collaborating with York on this, so Richard Hawkins is looking into the assurance part.
And again as I said, so we are looking at change, so here we are looking at something that we call county transfer assurance. So what happens if something changes in the environment and our assurance case might become enveloped through that right? So we want to make sure that we can still operate these systems safely. And so it’s an interesting question kind of whenever the environment changes the software is updated and so on, can we still perform these tasks safely?
And then in the last strand we are looking at the governance of these systems. So that looks more at the bigger picture in terms of regulation, what kind of tests or kind of processes do we need to deploy these vehicles for example safely on our roads. So we are looking in particular at a driving test for autonomous vehicles and involving our assurance cases as well as our kind of simulation part from the engineering.
So this brings really everything together and so we are looking at different ways whether we look at a process model or performance based model and we are looking at different technologies that they can utilise for this purpose.
And all of this is really integrated in the responsible research and innovation approach. So that is kind of led by the Responsible Technology Institute in Oxford with Marina Jirotka and so we are basically working with all the stakeholders and our partners, industrial partners from the very beginning and bringing them on to really understand the problems from the outset.
So I think that is kind of the main work we are doing in RAILS.
Sean: Fantastic thank you so much and great to hear RRI kind of embedded in that. But I did get a sense of like an immediate picture of an autonomous vehicle with L-plates on when you were explaining that last part there. So I have got to try and wipe that out of my brain before we carry on.
Shannon are you able to tell us about Making Systems Answer please?
Shannon: Absolutely. So I think it’s really important to focus in on the concept of responsibility and what we might mean by that. And our project is a collaboration between a number of disciplines, philosophy, cognitive science, socio-legal studies and computer science and Machine Learning. And the insight that we started with is that whether we are talking about moral responsibility or legal responsibility we are talking about social practices of balancing and constraining the kinds of powers that we unleash in the world that impact our relationships and achieve the relationship of trust, right?
So it’s about practices that enable trust and cooperative relationships that is the heart of what we mean by responsibility and so we start with that insight. And the first phase of the project that is led from the philosophy and cognitive science perspective is really about understanding this problem that’s been in the literature for about twenty years now, around responsibility gaps in AI and autonomous systems.
So this is the idea that AI, and in particular AI that is deployed with autonomous capabilities and self-learning capabilities, can present special challenges for responsibility that make it appear difficult or even in some cases seemingly impossible to appropriately assign responsibility. Particularly moral responsibility, which really is at the heart often even of how we think about legal responsibility right. When we ask who should be legally responsible we often start with intuitions about who is morally responsible.
But moral responsibility normally requires that you have an agent who knows what they are doing, who is in control of what they are doing and it’s not really conceivable that the kinds of AI systems that we have today have that kind of knowledge and control. But then the people deploying those systems often don’t have the insight into what the system is doing or the control over that system in real time to be the appropriate holder of responsibility either.
So this is the responsibility gap challenge right and there is different ways in the literature that people have tried to solve it. But one of the things that we began with is the insight that this is not really a new problem, this is not just an AI issue. We have had responsibility gaps in human moral and legal systems forever because humans often don’t know exactly what they are doing or why they are doing it. Humans often do things that we are responsible for even though we didn’t have real time control over the action for various reasons.
And so if you look at the literature in philosophy and cognitive science, there is actually a lot to learn about responsibility gaps between human agents. And so what we wanted first of all to do was to transfer that knowledge over to the AI and autonomous systems context. What can we learn from how humans deal with these challenges? And the insight that we have is that humans learn to become more responsible over time by making ourselves answerable to each other.
So it’s not that responsibility is an on-off switch or it’s either there or it isn’t there right. Responsibility is something that we build because we are not usually fully capable as responsible agents and yet we want to be more capable so that we can be more trustworthy. So that we can have stronger and more lasting relationships of trust with others that we need and want to rely upon and have rely upon us.
So what we are then saying is how can we use what we know about these practices of building responsibility and bridging responsibility gaps between people, how can we use that to accomplish the same goal with autonomous systems?
Then we have a second phase, so our first pillar basically sets out that problem and that goal. The second piece of work is really about getting the empirical data we need to understand what kinds of answers do people actually need. What kinds of expectations do people have of trustworthy agents across particular domains where autonomous systems are operating like health, government and finance? How can we fold these perspectives into regulatory and design approaches to autonomous systems?
So we don’t want the project just to be guided by philosophical intuitions or even kind of science. But we want it to be guided by what people actually think and expect and demand of each other in particular context of application so we have a programme of empirical research, qualitative research that is going on right now.
In fact we are having our focus groups this week in health, government and finance, to really learn more from key stakeholders about what do they think that responsible agents in these domains will be answerable for and how will they make themselves answerable. What kinds of answers should they be capable of giving?
And then the third pillar of research is really about translating that into insights for systems design, particularly using dialogical software design and tools to enhance the capacity of both humans and organisations to answer for the actions and impacts of autonomous systems.
So our project is called Making Systems Answer but that doesn’t mean that we think that software systems alone can be answerable agents, they can’t. They are not the right kind of thing, that’s the whole responsibility gap problem right. But systems aren’t just artefacts, systems include people and organisations, so we are talking about socio-technical systems. An autonomous system is made up of a lot of people, not just stuff, not just code. And so what we want to do is make the whole system answerable.
And what we think is that there are insights from these other pillars of work that I have described to help for example build a mediator software agent that could facilitate better communication between the system as a whole. And users or people who are impacted by the system to make that system as a whole more answerable over time and therefore more responsible over time.
So just as people become more capable of being responsible to one another through answerability practices, we think that is also true for socio-technical systems and for autonomous systems in particular. So we want to then figure out how this learning can translate into better computational tools for enhancing answerability in these kinds of systems.
[00:20:10]
Sean: That’s fantastic thank you so much for that. And obviously there are parallels in all of the three projects and various areas where obviously there is going to be overlap, you are all looking at a very similar kind of area of course, responsibility. And I was sort of moved by the fact that you all seem to have a lot of cross-disciplinary or multidisciplinary kind of, you know, interaction.
And it’s great to hear that those things are being integrated rather than just bolted on afterwards which is obviously all too common. How important is that to have those people involved in the project at these stages?
Lars: I think it’s very important to have this multidisciplinary approach from the outset. And yes we also collaborate with kind of lawyers, social scientists, engineers and I think that brings a really interesting facet to this problem. And also it comes with a lot of challenges of course because we have to agree and kind of try to understand each other. We have to agree on the same kind of language and terminology to be used.
For example autonomous system, autonomy is already kind of a word that is used very differently in the engineering context or on the social science context, so that is something we need to agree on. And also terminology like explain ability for example, that doesn’t, that means something different for different people right.
Sean: Yes the shared language which is both the kind of, what is it, connects us but also separates us? Ibrahim?
Ibrahim: It’s a good question on collaboration. So as you can see from the individual projects the individual we are highly collaborative in different disciplines. But also the four different projects at some point soon, maybe Shannon will say more about it, will come together to collaborate.
But the interesting thing about it is this has taken more than a year to happen. Which is a good thing in my view because you want people to come to the meetings, the workshop, with ideas that they have thought through rather than with just this sheer interest in collaboration.
So it is good for you to do your homework and say well this is what we have done so far, this I can present okay and let’s come together. Versus what you see in other circles which is, no just forget about it, let’s collaborate. And then you are writing a paper by community which takes ten years.
So I think that’s, I think a healthy thing which is happening within I think these four projects I think.
Shannon: Yeah, I mean that’s a great point that Ibrahim just made. And I think when I was talking earlier about socio-technical systems you can’t understand or govern socio-technical systems or build good socio-technical systems without socio-technical expertise. But the fact of the matter is most of us have been trained within disciplines that have much narrower conceptions of expertise and methodologies that have been developed over decades or centuries or millennia to actually facilitate specialist knowledge.
And so as Ibrahim is pointing out, you can’t just sort of force these things together and make a coherent understanding out of them by sheer force of will right or good intentions. There are actually special skills required to bring these different bodies of knowledge together in a way that they can talk to each other and build on each other.
And so as he hinted we are actually planning to all come together on the 10th of July in advance of a symposium that is being held on the 11th and 12th in Edinburgh. The Trustworthy Autonomous Systems symposium will bring a lot of this work together beyond the responsibility projects but include the other nodes of the Trustworthy Autonomous Systems programme.
But we are going to meet together, just the four responsibility projects, on the 10th of July to really share where we have got to about midway right through our projects. And understand where there is convergence, where there is opportunities for further collaboration and where. I think this phase is also about application, so we have now a lot of us done the kind of preliminary scoping and analysis that allows us to figure out where this can be put to work in the world.
So I didn’t mention actually our project partners, we are working with NHS-X which is the NHS AI, Scottish government’s digital directorate and also the software enterprise company SAS, to think about how these things that we are learning can actually be built for practitioners to use. And give them actually the practical guidance that they desperately need to make their own systems more trustworthy and answerable.
So I am really excited about us getting together to be able to think about these. It’s not just about translating academic knowledge so that it is accessible to other academics in other disciplines. We then have that second translational challenge which is exporting that academic knowledge that is now interdisciplinary into non-academic context where it can actually be used. And that’s the really exciting phase but that’s an even greater challenge.
Sean: It is kind of like an hour glass shape this, as you go forward and you refine your knowledge and, you know you get more and more concentrated on a specific area and then we are trying to bring that back out again and eventually hopefully end up being accessible to all I suspect.
I mean I did, Lars I will come to you in a sec. I did some research before this and I think I mistyped responsibility and I found out about responsible AI. But I am just wondering if there is a crossover there? Is that something that, you know is there a connection there? Because all of the big tech firms have huge amounts of kind of statements about their sort of responsibility, well their responsible environment and sustainability kind of like practices, is there a crossover or are we siloed here into responsibility? I don’t know.
Shannon I will stick with you just for a moment. I will come to you in a moment Lars.
Shannon: Yeah thanks Sean. So with my other hat, I am now co-directing a UK wide programme called BRAID, which is Bridging Responsible AI Divides. And that’s really about understanding the whole responsible AI landscape and ecosystem and understanding how to build that into something that is meaningful and effective and sustainable over time in the UK and more broadly.
So there in that project our focus is responsible AI. And absolutely I think there is this intersection between responsibility and what we mean when we talk about responsible AI. And I think it has to do with this element of accountability and answerability that I mentioned earlier. When we ask companies to align with the goals of responsible AI research and practice and to develop systems to promote responsible innovation and then we ask regulators to ensure that that’s happening, right.
What we are asking for is that the power of these platforms. The power of these large software companies, the power of these technological behemoths as well as the start-ups, the small to medium enterprises that also reshaping the landscape of society, what we want is for those actors in society to be answerable for their power, just as individuals are, right.
If I go out into the world and I push someone down to get them out of my way I am responsible and answerable for that, for the way I have misused my power to serve my own ends. And any functional civil society depends upon the trust we have in one another to use our power responsibly.
And so I think responsible AI is really about recognising that power is great, power is welcome, power is how society gets things done. But you can’t have a functional society built on unaccountable and answerable powers and that is unfortunately what we have allowed to happen in a large part of the tech-eco system and that is what we are trying to change right now.
Sean: Thank you Shannon.
Lars: I wanted to add to the kind of discussion around use cases and kind of further collaboration. So I think it is a great opportunity to come around those use cases which are maybe framed by industry and industrial partners I think that’s really important. And I think there is some appetite from the kind of industry to really work with us for example on the context in RAILS on the responsible AI index.
And so you have these kind of different metrics of how you evaluate these systems and you need to do this on the scale, on a big scale. For example we are collaborating with AWS and they have huge systems in the cloud running and they need to assess this kind of automatically in some ways. So I think that together with them we are trying to find and elaborate on some of these metrics.
But also in other cases in terms of use cases we discussed potential collaborations with Ibrahim and his project earlier, I am going to York tomorrow so we are kind of working in slightly different context on drones, so not only autonomous vehicles. But this is maybe another opportunity where we could look at assurances cases and the responsibility aspects over there.
Ibrahim: Yes, maybe I will elaborate more on what Shannon said about the question of power. Because I am talking about it as an engineer rather than from the other side as it were.
[00:30:01]
So in the past when you approached an engineer about the question of responsibility, their immediate answer would be a long time ago, hopefully we will improve this, we are just developing the technology. But as we know now this issue is challenged by AI because AI interferes with the logic in the world, with the main experts of what they are expected to do with it, their judgement as professionals. And that is challenging what engineers are doing and what is acceptable.
And there I can say something, again as an engineer, where I could say that, I will comfortably say that engineers are around twenty years behind conditions when it comes to the question of dealing with ethically sensitive issues.
I will give you an example, if you talk to a clinician about making ethically sensitive decisions and how often they do that, they will tell you every day that’s our job. If you ask an engineer, and maybe I am unfair about this, about how often do they face these kinds of decisions sometimes their answer is what did we do wrong? Why are you asking me this question?
Again because of us as educators we haven’t done enough on telling them that, we are training them to be professional engineers not just developers or programmers or hackers or the like. So that is an important thing however, we are improving on that front, so that is one aspect of that.
The other aspect which is related to not engineering but multidisciplinary is given the scale of the challenge and the different sources of power, I think the issue requires a lot of humility from the different disciplines. Because we can easily say engineers can say to clinicians or drivers what do you know about the technology to challenge me on X? But equally a clinician might say what do you know about diabetes to think that you can answer the question? And that’s why we have to get like initially space, and rule each other and agree on that space, and once we do this we can go for a robust discussion because each one of us can go to the comfort zone.
But given again the scale of the challenge, I think this is what we have been trying to do at least here and in other places for the last five years, which is try and understand this common space and protect it and not quickly go back to the comfort of your space. Because that is the easiest thing to do and because these are engineers, lawyers, philosophers, social scientists. It is a process but I am optimistic that we are getting there.
Sean: Just going back to kind of basics, any engineering project involving the end user in the development is obviously going to be a good thing whether you are building a bridge or a software tool. If you know what is going to be trying to drive over the bridge or walkover the bridge it’s obviously going to be a better bridge for the job.
Something that cropped up a little bit in the initial presentations you all made was the idea of regulation. We have obviously got the EU AI Act that is potentially going to come into force in the next couple of years. How easy or difficult is it for AI, you know, the responsibility idea of AI to kind of fall in with regulation? Regulation seems to be always a little bit behind. Anyone want to kind of have a conversation about that?
Lars: That’s a very timely point. I have just yesterday been to the TAS Regulatory workshop, so meeting some of the regulators from the TAS programme. And it was very interesting kind of going through all the discussions.
There are a few kind of points on large language models and how do you regulate these kind of AI systems in that space. But yeah I think there are kind of challenges kind of everywhere and also because the regulators are constricted to their own let’s say domains and kind of workspaces and industry operates.
So in some sense you would need this kind of regulatory, every regulator looking at this problem in their own way basically and trying to incorporate that. And so we also discussed kind of the role of experts on these AI systems. And maybe feeding into these kind of different types of regulators that might be quite useful to get to grips with it because it’s kind of a challenging technology I guess.
Ibrahim: As we all know regulations lack that is my definition. First you have to understand what is going on and then you regulate. A problem with the current discussion is everyone is waiting for the regulators to tell them what to do. And I think that people have to wait a very long time because first regulators have to agree on how to regulate but then create regulation standards, guidelines, they take a long time.
So I have been on many of these committees and it takes time and effort. And therefore we can’t afford to wait because those systems are being deployed at the moment, if you go to any street you can see them driving by as it were.
And that’s why I think I go back to what Shannon said about rather than waiting on the legal side, we have to invoke a sense of professionalism as far as the people who are creating and deploying those systems are concerned. And appeal to their moral sense if you like in the sense that society or people are trusting them to do the right thing even though there is uncertainty and things are changing because that is part of their job.
So at the moment I think it’s good to think about regulations, they are very important. But I think in the meanwhile we can’t just wait without trying to capitalise on something else that we have as humans and as professionals, which is despite of being a professional engineer or doctor or the like when it becomes uncertainty that’s where the sense of professionalism is important.
Shannon: I think one of the most important sides is that regulation is an essential part of a healthy innovation ecosystem because it enables trust. It enables people to take risks that they wouldn’t want to take in an unregulated environment where they can’t estimate the risk, where they bear the sole responsibility for managing it.
And I think we often hear, and have for years heard, regulation posed as this threat to innovation and this threat to the advancement of the technology. When appropriate and adaptive and informed regulation is actually a vital ingredient of healthy innovation and of technological advancement and we are certainly seeing that with AI.
And so I hope that we are able then going forward to have a more mature discussion of the proper role of regulation in innovation as opposed to seeing these as hostile opponents.
Sean: Thank you. That is a good take on it. There is one thing I would just put out there which is in kind of the bit of research that I did there was one classic thing that Google had said. Which is that you know they had this list of recommended practice including human centred design and test, test, test and the last thing on their list was continue to monitor and update after deployment right, that’s one of the things that they said.
And I find that slightly concerning because how many tech firms have developed something then abandoned it? Google are classics for this I am afraid to say. And Ibrahim you have got your hand up so I am going to come over to you.
Ibrahim: I find this interesting because if you look at any degree in computer science you see that these are basics. Like human centred design, all, I am sure the majority of degrees in the UK they teach this as a managing module as well as assessing. These are the basics, like this is computer science 101.
But when it comes to monitoring of course we have to monitor, that’s what everyone does. If you go to any engineering company and say please deploy your system and just run away they will say no, no of course, that’s why we have recalls in the automotive industry and. So these big tech company by saying this they have to be careful in what they are revealing about what they do, okay.
So yeah I am glad to hear all of this. But the challenge is at the higher scale where we expect them to talk about far more advanced things other than say well of course we take users into account and we monitor what’s going on.
So that’s why I think academia has more research and researchers have to push their agenda further. But also that’s where industry have to reveal what they are doing in terms of going beyond the basics because when they say this, and policy makers here, they reassure oh yes of course monitoring. But when researchers and other engineers hear this and say but that is what we have always done, that stuff is the basics.
So I hope we can advance the discussion beyond just saying that we are considering the users or we are monitoring after deployment.
Sean: Shannon?
Shannon: Yeah, that is so important what Ibrahim just said because as he is pointing out right this is not just computer science 101, this is engineering 101 right, you know build a bridge and then never go back and examine it again, right. Structural engineering, civil engineering and mechanical engineering has always included monitoring of how things are working out in the world. How they are bearing up under expected pressures and strains. How people are interacting with them differently than we expected. This is just basic stuff. And as Ibrahim says, the fact that we even have to have a conversation about doing this as if it’s a question is quite revealing.
But one of the things that really needs to be discussed is who pays for it? And who sets the standards for monitoring and evaluation right. So evaluation doesn’t come cheap, monitoring doesn’t come cheap and there hasn’t been a mature discussion at the regulatory level about how or what are currently being externalised on society. Society, the people that are getting impacted by this systems are now having to do the monitoring work themselves and raise the red flags instead of this being costed or internalised within the system and appropriately distributed.
[00:40:29]
And secondly you know you don’t want to allow companies necessarily to just always be able to mark their own homework. So you have to decide what are the external standards for proper monitoring and evaluation and there is a lot of great discussion about that right now.
But I am very much encouraged that I think we are coming to understand that this is a major gap in the tech ecosystem that has to be filled. So I am hopeful in the next few years we will see a more mature development towards meeting that basic expectation.
Sean: Lars?
Lars: Yeah thanks for this question Sean. So yeah I think it’s super interesting and important the monitoring. And as Ibrahim and Shannon both said so this is also very at the core of the RAILS project. So we are looking at particular at this kind of change as I pointed out earlier so the internal-external change. And particularly if we have autonomous robot systems we cannot control these environments so they operate in this open endedness something in the ecosystem will change, they change themselves. So it is really important to have the monitoring in place.
And as Shannon said, this maybe also requires new standards. So that these might be set by regulators kind of how often these need to be monitored as to be dependent of course on the application. So whether it’s kind of on a regular basis you want to do this yearly or whether you want to do it whenever a kind of software system comes in, basically an update to the software system comes in. So it is very much important from the government side point of view how we deal with it. So yeah I would say it’s super important.
Sean: Thank you for that. And definitely RAILS is obviously connected to, you know, autonomous vehicles or autonomous, you know. I think we are used to this idea of the responsibility for driving a vehicle passing from owner to owner to driver to driver and having this idea of okay what happens, where is the responsibility? Is it in the developer of the software, the manufacturer of the vehicle? The owner, the user, the person who is sitting in the back? I mean I am really glad that you are working on these things.
Lars: Yeah maybe just briefly on that. So yeah, so one of the core aspects we are working on is the kind of explain ability to make these systems accountable to really understand what is happening inside. So we are trying to kind of understand kind of what is happening around these systems in terms of seen understanding and reason about what does it mean? What does it mean for the actions? Why have the systems done certain actions and explain this.
And explain this at different levels, so either to the end user, to the developer or to an accident investigator. So we also need different types of explanations and these are really key I think in making these systems trustworthy.
Sean: Well look the responsibility now falls for me to say thank you all for sparing the time to be here today, it’s really been great to have you on the podcast. So just to say thanks to each of you really so thank you to Lars, thank you to Ibrahim and thank you to Shannon.
Shannon: Thank you so much, it’s been great.
Sean: If you want to get in touch with us here at the Living with AI podcast you can visit the TAS website at www.TAS.ac.uk where you can also find out more about the Trustworthy Autonomous Systems Hub. The living with AI podcast is a production of the Trustworthy Autonomous Systems Hub. Audio engineering was by Boardie Limited. Our theme music is Weekend in Tatooine by Unicorn Heads and it was presented by me, Sean Riley.
[00:44:21]