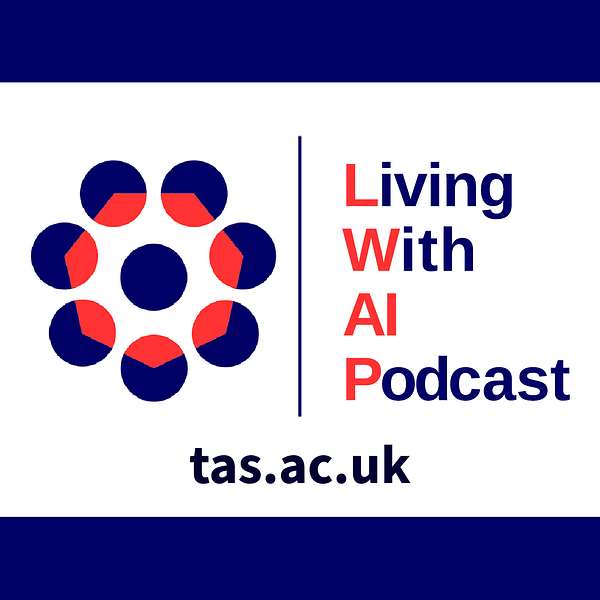
Living With AI Podcast: Challenges of Living with Artificial Intelligence
This podcast digs into key issues that arise when building, operating, and using machines and apps that are powered by artificial intelligence. We look at industry, homes and cities. AI is increasingly being used to help optimise our lives, making software and machines faster, more precise, and generally easier to use. However, they also raise concerns when they fail, misuse our data, or are too complex for the users to understand their implications. Set up by the UKRI Trustworthy Autonomous Systems Hub this podcast brings in experts in the field from Industry & Academia to discuss Robots in Space, Driverless Cars, Autonomous Ships, Drones, Covid-19 Track & Trace and much more. If you have any questions or suggestions for future topics, please email us at contact@tas.ac.uk - You can find out more on our website at www.tas.ac.uk#LWAIP #TASHub
Living With AI Podcast: Challenges of Living with Artificial Intelligence
TAS for Health and Social Care
•
Sean Riley
•
Season 3
•
Episode 10
This projects episode features four TAS Hub projects associated with Health and Social Care:
1. Tunde Ashaolu - DAISY
2. Yasmeen Rafiq - Verifiably Human-Centric Robot Assisted Dressing
3. Cian O’Donovan - Empowering Future Care Workforces
4. Navamayooran Thavanesan – Imaging predictors of Oesophageal Cancer MDT patient outcomes
Podcast Host: Sean Riley