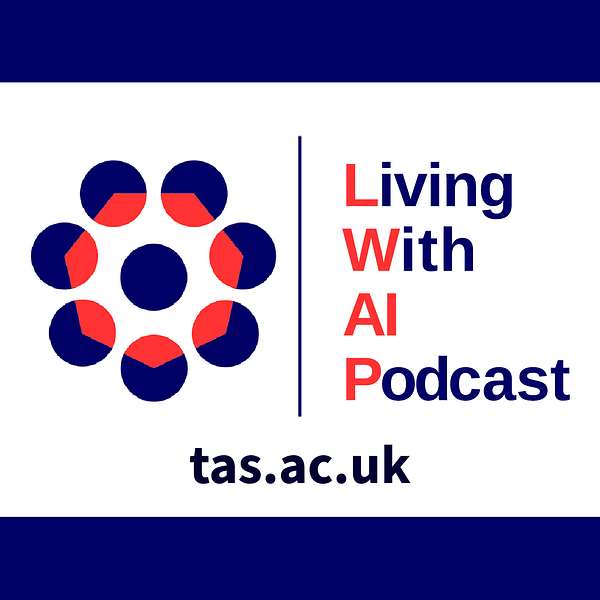
Living With AI Podcast: Challenges of Living with Artificial Intelligence
Living With AI Podcast: Challenges of Living with Artificial Intelligence
AI & Misinformation
Misinformation online is a huge problem. How can you trust the things you read?
Jeremie Clos joins Sean to discuss the project "Privacy Preserving Detection of Online Misinformation"
Podcast production by boardie.com
Podcast Host: Sean Riley
Producers: Louise Male and Stacha Hicks
If you want to get in touch with us here at the Living with AI Podcast, you can visit the TAS Hub website at www.tas.ac.uk where you can also find out more about the Trustworthy Autonomous Systems Hub Living With AI Podcast.
Podcast Host: Sean Riley
The UKRI Trustworthy Autonomous Systems (TAS) Hub Website
Living With AI Podcast: Challenges of Living with Artificial Intelligence
This podcast digs into key issues that arise when building, operating, and using machines and apps that are powered by artificial intelligence. We look at industry, homes and cities. AI is increasingly being used to help optimise our lives, making software and machines faster, more precise, and generally easier to use. However, they also raise concerns when they fail, misuse our data, or are too complex for the users to understand their implications. Set up by the UKRI Trustworthy Autonomous Systems Hub this podcast brings in experts in the field from Industry & Academia to discuss Robots in Space, Driverless Cars, Autonomous Ships, Drones, Covid-19 Track & Trace and much more.
Season: 3, Episode: 11
AI & Misinformation
Misinformation online is a huge problem. How can you trust the things you read?
Jeremie Clos joins Sean to discuss the project "Privacy Preserving Detection of Online Misinformation"
Podcast production by boardie.com
Podcast Host: Sean Riley
Producers: Louise Male and Stacha Hicks
If you want to get in touch with us here at the Living with AI Podcast, you can visit the TAS Hub website at www.tas.ac.uk where you can also find out more about the Trustworthy Autonomous Systems Hub Living With AI Podcast.
Episode Transcript:
Sean: Welcome to the Living With AI, a podcast where we look at how artificial intelligence is changing our lives and the impact it has on our wellbeing. Today’s topic is AI and online misinformation. I’m joined by Jermie Clos, an assistant professor at the University of Nottingham, and he’s been working on a TAS project titled Privacy Preserving Detection of Online Misinformation. Welcome to the podcast Jeremie.
Jeremie: Hello.
Sean: Before we get started, we’re recording this on 6 September 2023, so just keep this in mind whenever you’re listening to this. Jeremie, I mean, where do we start with this? Can we- Just give us a bit of background to the project, and I’m not going to ask why it’s important, I think we should all know why this is important, but tell me a bit about it.
Jeremie: Yeah, okay, all right, so basically this project comes from- We have multiple rationales for this project, right? So there’s two bits. There’s the online misinformation bit which, as you said is quite obvious, right? We know that this is a problem. Everybody knows it’s a problem. We hear it all the time. But then there’s the privacy preserving bit which is the bit that actually, people don’t really seem to understand the reason for, until we explain it to them. And the way I usually explain this project is actually quite simple. Imagine if I told you that I would like you to count, right, how many times you are exposed to swear words online. What- the traditional way of doing something like this is that I would go- And when I say you, I mean in general, the public, right? I would go on a website, like, let’s say Twitter, and I would just grab all the data I can and I would analyse what’s happening there. Then I would just take whatever statistics I have, divided by the number of people and that would be my number and, we know, just say like that, that’s fine, right? Except of course it’s not for multiple reasons. First, nobody actually gave you, you know, permission to use that data. You just grabbed it without asking. The second one is that you’re making a very wild assumption about people by selecting a specific dataset like this and then grabbing it and assuming that it’s representative of the world. For instance, if the website in question was Twitter, that means that I’m assuming that people who go online are just Twitter aged people and that’s making some very strong assumptions about who we’re talking about, where they come from, and so on. And finally, maybe the most important one, is that we’re headed towards a lot more encrypted communication into unencrypted, and thus an issue that we debate quite a lot is whether we should have this encryption. But the fact is, it is here now and if it is encrypted, it means you can’t even see what’s happening there. So the way we need to do this is that we need to observe those signals, well, at either end of the communication. So either the person that is generating the swear word or the person who’s just hearing it. And this is where the privacy preserving comes into play, right? Because swear words is a very simple problem to solve. But misinformation is a lot more complex and nobody who’s generating misinformation is going to let you analyse what’s happening on their computer, for obvious reasons. But people who are receiving it on the other end might let you analyse things in a privacy preserving way, and then kind of collect this signal over a large population without violating their privacy. And that’s kind of it, in a nutshell. So we want to make sure that we have a way of analysing those kind of phenomenon without violating privacy and without making very strong assumptions about the people that are victims of these kinds of things.
Sean: There’s a problem here in terms of qualifying stuff though as well, because I would say there’s a sliding scale of swear words right? I mean, you might say some very mild swear words and then some people might have different cut offs as to what is swearing and what is kind of severe profanity, if you like. Is the same thing true in misinformation? Is it, you know, is it okay if someone’s maybe telling a white lie online and then, you know, how does that work?
Jeremie: Yeah, so you’re completely right, there is a cultural- The cultural kind of aspect to this. Swear words are a thing, but misinformation is a lot more complex and for that reason, why I said that we’re going to analyse it at the end, what we mean by that is that we’re going to go and put like a little bit of software on your computer and it’s going to be analysing what you see. Nothing is going to come out of it. Like, we don’t receive anything. Like it stays in the computer, and then you’re always in control of your data, and that’s the key to this, right? And because you’re in control of this data, that means that you have- You’re kind of involved, almost like a citizen science aspect to it. I say Sean, can you go and like check that this data is actually misinformation. Like, we think that all those things you’ve seen might be attempts at propaganda, do you agree? Why? Why not? And so on. And then once you’ve done that step, then are you okay with sending us some aggregated statistics of what you’ve observed? And this way, you’re always in control of the data, whether it’s- When it’s generated, when it’s sent, and you’re like nothing- Like it’s all under your control.
Sean: I think you know, you’ve hit the nail on the head with the propaganda thing. That’s like the headline for this, isn’t it? You know? We’ve seen people talking about the US 2016 election, the Brexit vote, things like this. People have claimed misinformation targeted campaigns have affected them, if not entirely decided them. But how do we approach that? Because often, people are seeing what they want to see aren’t they? I mean, it’s sort of self-reinforcing. Who’s going to choose to be told that what they’re reading and they want to know- Sorry, I’m kind of making assumptions here, but often, people are reading something that supports their existing sort of beliefs right? And they enjoy that kind of self- Not certification, but yeah.
Jeremie: Yeah, I mean, you’re completely right. Like, you cannot help people against their will. That’s a simple fact that we have to deal with. However, what we found- So this is a pilot project and I need to qualify this, the data collection is still in progress, so we’re starting to see results but it’s taking time because it’s quite a complex thing to do, and it’s a very small project in terms of manpower. But what we’re seeing is that people are actually interested in whether they’re being lied to, right? They just don’t know what to look for. And we take a very specific angle to this. Like, we’re specifically looking for linguistic constructs. So it could be stuff like turns of phrases, specific vocabulary, specific ways of writing things which tend to be indicative at an attempt at misleading people. So we can’t say, oh, look at this, this is misinformation, this is not misinformation, and so on. However, what we do say is, look at all those words, look at those specific sentences. These tend to be indicative of misinformation and now you can take the steps to actually check whether it is. But of course, we can’t force people to do that for good reasons I think.
Sean: Yeah, absolutely, absolutely. But I mean, you’re absolutely right, I think people do want to know if they’re being lied to. I mean, that’s really important and that kind of feeds back into the trust element of the Trustworthy Autonomous Systems Hub. We want to be able to trust stuff. So it’s interesting that you’re taking this linguistic view. Are you going to have things like satire coming up as false positives though? Is that a bit of a challenge?
Jeremie: That is a challenge, but fortunately, satire does tend to be a very small percentage of online traffic. But it is a challenge. And not just that, a lot of misinformation is a lot more subtle than sentences. Things like manipulation of images, manipulation of sounds, like some complex- But this is like a starting point basically. What we’re trying to find out is whether people are amenable of having this kind of software on their computers and they tend to be, which is very encouraging because now that we know that people don’t mind participating in basically, you know, cleaning the web, like seeing how bad is it now, then it means that we can build more complex things in future projects for detecting images, sounds, videos which might be manipulated, and kind of pushing it forward as much as we can. But you’re right, it is very complex and it’s good that you said the date at the beginning because I don’t think we’re going to solve misinformation now or in 10 years or in 50 years.
Sean: It’s an ongoing fight isn’t it? I mean, the other thing, of course, you mentioned manipulation of images and videos, but kind of probably more importantly here, right at this moment, is the ability for AI to generate huge amounts of text that might not be misinformation, and perhaps that will learn about phrasing things differertly. Is that an issue?
[00:09:41]
Jeremie: It is an issue.
Sean: I’m thinking, yes, I’m thinking of, kind of, you’re looking for those linguistic sort of phrases, but then it’s an arms race then isn’t it? It becomes, well, we’ll try not to use those linguistic phrases.
Jeremie: Yeah, you’re right, and I mean, I assume you’re referring to things like large language models and like GPT and all that. It is a problem and I think the thing is, we’ve always had ways of generating text like this. It was not as complex. What we have now is it can make it look extremely realistic, but generating text has never been an issue. It’s all tech. It’s just that now it’s becoming harder and harder to distinguish artificial from naturally generated. So I don’t know that it’s going to be an arms race in that aspect. We take more of a, you know- We want to build an observatory and then we can decide what to do about it. This is the first step and I don’t know that there is a solution for the large language model issue. It’s more of a social thing, like being sceptical of what you read online, rather than a technical challenge.
Sean: But that also feeds in a little bit into kind of the education element right? So people need to learn about this and, for my money, I think they already probably learn a certain amount in schools, but that definitely needs to be enhanced doesn’t it? You know? People need to have the old adage, don’t believe everything you read, sort of drilled into them. Because sometimes it seems too convenient, doesn’t it, when you see it on the screen in front of you.
Jeremie: Yes, and especially if it confirms already existing biases, which is the dangerous bit. But I think there is, as you say, that’s an education issue and more of critical thinking, like I want to say, scientific culture aspect of it, like, you know, knowing what is in the realm of possible helps you in distinguishing misinformation from information. But I don’t know. It’s a very complex issue. But yeah, you’re right, education is definitely part of it, if not most of it.
Sean: Yeah, the other thing I was thinking when I saw about this project is that companies like Facebook and Twitter have- Are we supposed to call it X now? X, formerly known as Twitter, I don’t know, I’m just going to make light of that one, but, you know, have tried to implement misinformation kind of tools before and I think there can sometimes be an issue with assuming somebody is making a decision for you. So how do we get around that? That idea that these academics, they think they know what’s true and what’s not. How do we get around that kind of preconception?
Jeremie: Yes, that is part of the reason why we kind of strayed away from making a specific statement about what is and what is not misinformation and then focused on advising people to look at and then confirm information instead because, as you said, like, and it’s been observed I think, I don’t recall the specific papers but I know Twitter specifically, they have this like committee notes/fact check per note, like, well, this has been found to not be true. But in practice, if you really were convinced of something, this is not what’s going to sway you one way or the other. So I’m not too sure that- I mean, this is probably part of the solution but it’s not the entire solution. It’s not going to do anything about existing biases.
Sean: But I see- I mean, from what you’ve described it feels a bit more like when people voluntarily put, say, I don’t know, a virus checker on their computer, it’s like you’re thinking, you know, if you’re educated enough to know that there might be things out there and you see that there’s a tool that can help you with that, then hopefully people will download this and add it. Is it a bit like an extension on a browser or something like that?
Jeremie: It is yeah. It’s an extension in the browser as a first step, because we want it mostly focused on online information that you can see, like websites, social media and so on. It’s a browser extension which analyses basically all the text that’s displayed and just computes statistics and then runs some very small machine learning algorithms embedded in the extension to analyse basically what’s happening and try to make guesses as to something might or might not be indicative of misinformation. But yeah, as you said, this is almost preaching to the converted. It’s like, if you install something like this, you already are the kind of person who’s going to be sceptical of what they read and therefore- But on the other hand, I like to think that this is more of a feasibility study of embedding this kind of application rather than a proper long-term study of how do we make people care about this. Because from the data that we have, we see that people do care about it. But then, as you said, well, if they didn’t care, they wouldn’t have participated in the experiments. So who’s to say?
Sean: So it’s self-fulfilling in some respects. I think the other thing I was thinking is- Maybe, me being a layperson in this means I may have got this slightly wrong, but if you enter into this sort of misinformation sphere- I may be making more of it than I need to, but you have obviously the browser possibly collecting cookies and then maybe relating or referring you to- Or certain sites referring you to more misinformation, and is there kind of a snowball effect that occurs when that sort of happens?
Jeremie: Yeah, that does tend to happen in websites which are driven by recommended systems. So things like YouTube right? If you want a video on YouTube then it’s extremely fast at learning about what you’re watching and then recommending you more of it and it’s very easy to go on YouTube. But that’s not the case for like Facebook groups, TikTok videos and so on. It’s very easy to go from an innocent video that you click on to the most vile like far right propaganda you can think of just in a few clicks, just because it learns from a very weak signal and because those websites are based on engagement, they learn that if you click on this and you watch the entire video, it means that by giving you something slightly more extreme they’ll keep you here longer and shove more ads in your face.
Sean: Yeah, yeah. I mean, you know, it does come down to the mighty dollar at the end of the day often with these things. Unfortunately, the side effect can be that you just radicalise somebody in one direction or another. Do you think there’s a kind of possibility that the tools that you’re describing might be able to apply to things like online videos as well? Or is it more- At the moment, as a proof of concept you’re looking at text and websites or news articles or whatever and-
Jeremie: Yeah, so yeah, I do think that things like videos are potentially things that we could be analysing. Pictures might be easier because it’s easier to find out if something has been manipulated just by analysing images. Videos are a bit trickier, but there are algorithms that we can use to detect whether something has been manipulated. I say manipulated, that’s the wrong word for it. Like, the most common form of using videos or images for manipulation is actually just recycling existing videos out of their context and then using them in other things. So if you can detect that this certain image of something comes from a movie or a very old news article and has just been repurposed to propel some hate speech or whatever, then you know that this is misinformation.
Sean: One of the very obvious ones I remember seeing quite a lot a few years ago was an old photo of the UK’s House of Commons with nobody in it and then underneath would be the quote “MPs when voting on” delete where applicable. Whatever very important topic, not there. Then next to it would be a house full of- You know, the house was full of MPs all voting- And this is the MPs voting on their own payrise or something like that. And then, you know, these photos, there’s no verification that these photos came from anywhere or any specific time period and you would have to do quite a lot of fact checking and research to prove or disprove these things but it’s easy to see that and think somebody’s already done that work and just go along with it, right?
Jeremie: Yeah, yeah, yeah, it is. I mean, there are some measures of information literacy that it can take, almost like information hygiene to an extent in terms of fact checking, but it is tricky and time-consuming. So-
Sean: And as you say, we’re preaching to the converted. The people who are going to do that are already sceptical and therefore- Is there any mileage in looking at the metadata with some of these things? So, for example, because I work with YouTube quite a lot, I know that we upload video descriptions and hashtags and all sorts of tags to try and set the, if you like, context for that video so that the right people find it and I’m assuming, of course, the algorithm uses that in a way to do the recommendations etc. So could that be something that gets looked at? The metadata around the images or videos?
[00:19:55]
Jeremie: Assuming we have access to it, potentially yes. But once again, because we’re working with client side of things, we’re not assuming anything about the person serving the content, then it can be tricky because sometimes we don’t really have access to those things. They’re used in the background to search and propose and push data, push information on people, but they’re not really accessible all the time.
Sean: And that comes back to your central premise of not sending data off the computer and keeping it client side right?
Jeremie: Exactly, yeah.
Sean: I’ve been a bit technical with you and perhaps we need to be a bit more kind of TAS Huby.
Jeremie: It’s not that technical in the sense that we don’t go into details, but the autonomous aspect of it, the autonomous system is really simple, right? You have very small algorithms and they’re deployed on your computer and because they don’t communuicate any data and they ask you for permission all the time, then you trust them. Or at least you should trust them. I guess one more thing that I always find interesting and surprising is that we find two categories of people. There’s the ones that really bought into the citizen science thing and they were like, yes, I want to help fight misinformation, and some people just want to give their data away. Like, yeah, just have it. I’m not even going to read the consent form. You look like a trustworthy person, just have my data. I was like no, you shouldn’t do that. That’s the opposite of what we want. You should be doubting us all the time.
Sean: Yeah, that’s quite interesting isn’t it? I mean, was this sort of when you were recruiting the people to be part of this? Were there people meeting people or was it online? How did you do it?
Jeremie: No, it was physical because we wanted to observe them using the software and then see the pain points and make sure that we have an idea of what disturbs them, do they understand it, do they understand what’s happening? And also, after that, we had like group discussions just to see what was their experience of misinformation online. Do they think they might have populated misinformation by accident before? A lot of them do and they feel bad about it. It’s not their fault. I mean, nobody has the time to fact check everything. But yeah, like some of the things, like- Oh, the software is fine, just take my data. I don’t want to see all of this. And then some really bought into it. They were like yeah, I want to have tools so I can like send some information, like modify this, give you more stuff. It’s very interesting, the diversity in the recruitment process.
Sean: it’s the opposite of what you wanted. You want people to kind of question stuff and think about stuff and you’ve got people thinking no, don’t worry about it, everything’s fine. And those are still, even within the group of potentially sceptical people, there are still people who, yeah, make a snap decision perhaps.
Jeremie: Exactly, exactly. I think it’s just focused so much on the trustworthy systems but some people just trust whatever. They trust based on appearance, based on oh, that’s a university person and so on, and we need to disentangle that right? Because I want people to trust it, even if I was a random person off the street who built the software.
Sean: And also, you know, I suppose, and obviously thinking about my own experiences of things that I’ve seen online and things I’ve potentially shared and mistakes I’ll have made down the line of, you know, accidentally sharing something that’s misinformation, I’ve kind of found myself looking at the source and trusting certain sources because of my past experience. Perhaps national broadcasters or academic institutions, but you also need to realise that they make mistakes as well, never mind deliberate misinformation. There’s also mistaken information isn’t there? But yeah, life’s short and we don’t all have time to double check and treble check everything, so a tool that would help us with this would be, yeah, I think it’s an excellent idea.
Jeremie: Yeah. I mean, the core idea is that by pointing out those turns of phrases and so on, there could potentially be a training effect to it of making people more sceptical when they see those things. Like, for instance, a very common one, right, over emphatic language. Like hyper kind of opinionated kind of things. I mean, I think you and I both know that this is usually a signal that something should be taken with a grain of salt in the sense of a news article or news adjacent article. But that’s something that’s not obvious to some people. And then if you can tell them well look, look at this kind of language, this tends to be used sometimes to just convince people of their viewpoint despite facts, and we- So we haven’t run the experiments yet, because they need to be a long-term thing, and we just didn’t have the time basically, but we are hoping that down the line we can have a bit of a training effect by pointing out those things repeatedly to people, then they can kind of embed that in their own information consumption habits.
Sean: Yeah. I mean and that is going to be great, kind of longer term. I suppose there will be always some sort of subtle misinformation that kind of informs people as well, but you know, you can only do what you can do. I’m wondering, is this something people might be able to try or download, or is this a closed trial at the minute? Is this something- You know, your extension, is it released widely to possibly try it?
Jeremie: Oh, so it’s- The extension is open. That being said, it does rely on a central server which is not always running because it’s like currently being worked on, but both parts of the software are open source and openly downloadable. They just might not be open to the lay public in terms of you have to run and everything, it’s a bit of a pain. Yeah, it’s a piece of research software. So down the line, we’re hoping to develop that further and then make it accessible. But yeah.
Sean: That would be great as a kind of next step I suppose, and try to kind of make it a bit broader and see if you could open it out. Some nice sponsorship from someone like Amazon AWS or something like that, just to kind of, you know, pay for the server support would be great. So if anyone’s listening, contact Jeremie.
Jeremie: Yeah, if you want to ethics wash your business, do something good.
Sean: Jeremie, it’s been brilliant to talk to you today and I wish you all the luck because the project’s ongoing isn’t it?
Jeremie: Yeah. It’s- We’re towards the end but we started very late so it’s still- We still have some things to close, yeah.
Sean: Good stuff, well look, all the best for the rest of the project and thank you so much for joining us on the living with AI podcast.
Jeremie: Thank you.
Sean: If you want to get in touch with us here at the Living With AI podcast, you can visit the TAS website at www.tas.ac.uk, where you can also find out more about the Trustworthy Autonomous Systems Hub. The Living With AI podcast is a production of the Trustworthy Autonomous Systems Hub, audio engineering was by Boardie Limited, our theme music is Weekend In Tatooine by Unicorn Heads, and it was presented by me, Sean Riley.
[00:28:02]