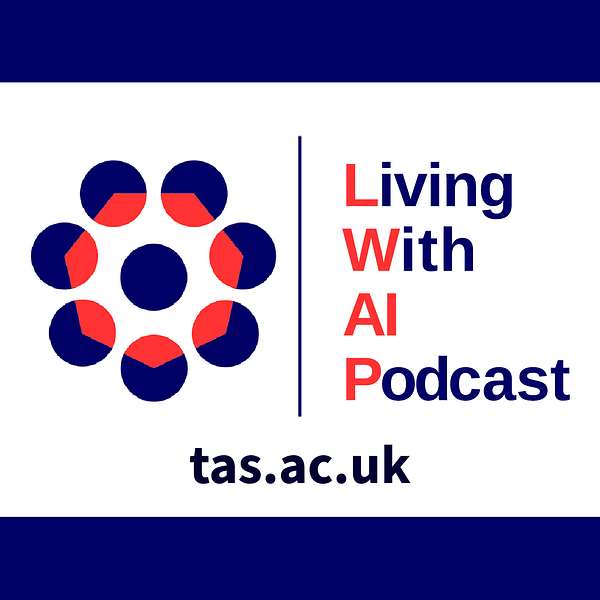
Living With AI Podcast: Challenges of Living with Artificial Intelligence
Living With AI Podcast: Challenges of Living with Artificial Intelligence
AI and Defence
Will machine learning abide by the rules of war? We're talking AI & defence and whether AI is already revolutionising the battlefield.
Our guest is Professor Chris Johnson, Pro-Vice-Chancellor, (Engineering and Physical Sciences), Queen’s University Belfast
Podcast production by boardie.com
Podcast Host: Sean Riley
Producer: Stacha Hicks
If you want to get in touch with us here at the Living with AI Podcast, you can visit the TAS Hub website at www.tas.ac.uk where you can also find out more about the Trustworthy Autonomous Systems Hub Living With AI Podcast.
Podcast Host: Sean Riley
The UKRI Trustworthy Autonomous Systems (TAS) Hub Website
Living With AI Podcast: Challenges of Living with Artificial Intelligence
This podcast digs into key issues that arise when building, operating, and using machines and apps that are powered by artificial intelligence. We look at industry, homes and cities. AI is increasingly being used to help optimise our lives, making software and machines faster, more precise, and generally easier to use. However, they also raise concerns when they fail, misuse our data, or are too complex for the users to understand their implications. Set up by the UKRI Trustworthy Autonomous Systems Hub this podcast brings in experts in the field from Industry & Academia to discuss Robots in Space, Driverless Cars, Autonomous Ships, Drones, Covid-19 Track & Trace and much more.
Season: 4, Episode: 1
AI & Defence
Will machine learning abide by the rules of war? We're talking AI & defence and whether AI is already revolutionising the battlefield.
Our guest is Professor Chris Johnson, Pro-Vice-Chancellor, (Engineering and Physical Sciences), Queen’s University Belfast
Podcast production by boardie.com
Podcast Host: Sean Riley
Producer: Stacha Hicks
If you want to get in touch with us here at the Living with AI Podcast, you can visit the TAS Hub website at www.tas.ac.uk where you can also find out more about the Trustworthy Autonomous Systems Hub Living With AI Podcast.
Episode Transcript:
Sean: Welcome to the Living with AI podcast from the Trustworthy Autonomous Systems Hub. This is season four, so there’s plenty of back episodes you can go and have a binge on. I’m your host Sean Riley, and surely we’ll welcome our guest, Professor Chris Johnson. You may have already guessed but this podcast is here to discuss AI and trust and trustworthiness. Now we’re recording this on 8 April 2024 and today’s topic is defence. So please welcome Chris to the show. Chris, thanks for being part of living with AI.
Chris: Good to meet you. I’m happy to help.
Sean: Great stuff. Could you give us a brief introduction? What’s your name and what do you do?
Chris: I’m Chris Johnson. I’m a professor of computing science and I run the science and engineering faculty at Queen’s University in Belfast.
Sean: Great stuff. Thanks for sparing your time. Now I will confess, I took the obvious and lazy approach to starting the research on this and I asked an AI about defence AI and trustworthiness and classically, it came out with some wonderful, you know, often repeated things. So I will give a quick read of it, and I’m not going to read the whole thing because it’s far too long, but the response was: Certainly, the approach to AI enabled capability in defence emphasises ambition, safety and responsibility. Let’s delve into the key principles. And then it lists ambition and safety and responsibility and trustworthiness and then it follows up with a summary of our commitment lies in ambition yet responsible AI adoption fostering trust amongst stakeholders which is what I want to call almost legalese. But I’m left wondering who our refers to, you know? The AI I used was Microsoft’s Bing, so is this Microsoft’s official statement? I don’t know. It just opens up that idea of this is the training of an AI right? So it’s just whatever it’s been trained with right?
Chris: I think it’s a lot more complicated and a lot more nuanced than that. So in the statement that you read, I can hear echoes of the US and the UK policy in the use of machine learning in defence.and I think that there’s a lot of kind of things that we need to consider. So, one is that other countries around the globe are looking at machine learning in defence and we have to decide whether or not we will engage in research and in development that uses those systems, you know? Because the alternatives might be that 17, 18, 19-year-olds end up being faced by systems, it doesn’t necessarily just need to be weapons. It could be organisational systems and planning systems that are machine learning equipped and do we want our sons and daughters to be put into that situation? On the other hand, there’s extreme concern in society about a range of moral dilemmas that arise from providing greater autonomy to computational systems, whether or not they use machine learning. And both in the UK and in the United States, the military organisations have decided to adopt ethical frameworks that constrain the use of machine learning. So I think that’s a really appropriate approach given the dilemma that our adversaries may use these technologies but with fewer scruples than perhaps we have.
Sean: This is the other sort of thing I was going bring up was the kind of the elephant in the room, is the bad actor, you know? The terrorist, the rogue operative, whatever, using this equipment for things that we all, maybe all, stand up and say we’re not going to do but that doesn’t stop somebody from doing it. I mean, how do we try and guard against that?
Chris: Well I think by adopting ethical principles that align with the rules of war and of conflict for existing conventional systems and finding a way through the questions that arise from that to ensure that if machine learning is going to be deployed that we have confidence that that machine learning will abide by the rules of war. So a very specific example would be that one of the ethical principles enshrined within the rules of war would be proportionality and that means that the degree of force that you use is proportionate to the military effect that’s going to be achieved and I think there’s an argument that machine learning, developed with ethnical principles in mind, may actually be more proportionate than a human equivalent.
Sean: Yeah, that’s quite an interesting take on it, you know, that actually it might be fairer than not using it. But I think you touched on something earlier about the idea of kind of not just weapon systems but systems in general, organisation systems, logistics systems, that other kind of- I’m going to say adversaries because we’re talking defence. Adversaries may be just better- I’m not going to say equipped, but more efficient, and that becomes a problem in its own right, doesn’t it?
Chris: I think that’s the concern but my feeling is that the approach that western governments have taken actually increases confidence that the machine learning will perform correctly and there are a host of concerns about the use of machine learning in wider organisational contexts, so in planning for instance, and if the algorithms and the training are not subject to verification and validation, it’s highly likely that those systems will induce incorrect behaviour and so verification and validation, improving the confidence, the testing and the processes that go around the development of machine learning applications, you know, I see them as an enabler and as something that improves confidence that they won’t have adverse side effects. And so while adversaries may pay less attention to these issues, by taking them seriously right from the start in this arena, I think not only will we be doing ethically the right thing but we’ll also have more reliable systems that do what we want them to do as the outcome and I think the military are perhaps slightly more nuanced in this than some other areas of industry that have used machine learning algorithms without thinking about the side effects.
Sean: I think that’s an interesting point and it’s also interesting we’re talking a little bit as if this is the future but of course, these sorts of systems are already in use aren’t they? You know? For things like logistics, for- Even down to using, I don’t know, Google Maps to organise where your supply set up might be and things, these things are being used now aren’t they?
Chris: In a very limited way within the military and in defence but I think the point you’re making is a good one in that in the future it’ll be very hard to extract machine learning from the operating systems and from the other applications that are embedded within commercial off-the-shelf tools and so the work that the military is focusing on is part of a broader continuum about trying to improve quality and confidence and trustworthiness in machine learning across the piece.
Sean: What I was wondering is I know the UK have a long statement on the use of things like machine learning etc and I know that the Trustworthy Autonomous Systems Hub has certain people to do that and have been involved in that. The top thing on the list, or one of the top things on the list is algorithmic bias and how do you deal with that in a situation like this?
Chris: Yeah, I think, I mean, algorithmic bias is a, you know, kind of a set, or if you like, a subset of a wider set of problems that are beginning to be better understood and there are approaches that can be employed that have an application in the military context but also are probably, or should probably be used more widely even in commercial or non-military applications. So an algorithmic bias is, you know, where you would have an unintended consequence for a subgroup of your input set, whether it’s a socioeconomic group or a racial subgroup, you would want to make sure that a side effect of an algorithm did not unduly discriminate against those people and so you know, an example of a technique that can be used is counterfactual reasoning.
[00:10:06]
So, an example there would be if I trained on a subset of people from one part of the world and then examined the behaviour of the alhorithm on a group of people from a different area, that the system wouldn’t be biased in its outcome based on particular regional grouping or other properties of people from that part of the world. And these sort of counterfactual techniques are kind of like a form of argument or test cases that you could apply to improve confidence in the system. So, for instance, an online shopping system might discriminate against people from a certain postcode because there had previously been evidence of a high number of payment defaults from that postcode. A counterfactual argument might be used to say well, what would have happened if a similar approach was used in a different area without the same degree of evidence? So, you know, generalisation that then restricted the goods that were offered to people from other areas based on observed effects in the first area. And that would clearly be unfair. So there are these approaches that are emerging that people can apply in everything from online shopping through to military systems and in a- What I think’s really important is that they’re well-publicised and the people who commission or deploy machine learning algorithms have enough understanding to be able to ask the questions about whether these biases exist.
Sean: Yeah I think that’s obviously massively important in all sorts of ways. The other thing that’s quite clear, certainly in the UK’s approach to this is that it’s- I mean, it’s spelled out, it’s human-centric, isn’t it? The idea is having a human in the loop. But also, you know, what are the implications for people? What are the potential unintended consequences? These are things that are spelled out in the UK’s approach to things like machine learning aren’t they? Do you think- Again, I kind of return to the question before in a way, just because we’re doing it, does that, you know, is it going to be okay or are other people going to take the human out of the loop, things are going to operate more quickly, more deadly?
Chris: I think that’s a philosophical question and you’re asking me to predict the future. I mean, so I don’t know. But my feeling is that we’re in a transition period because there are systems being developed around the globe which stretch the human ability to control them in the way that’s proposed to the very limit. You know? High energy weapons systems are capable of maintaining multiple targets simultaneously and engaging those targets at a rate that’s far faster or higher than a human might be able to cope with. And similarly, the conventional systems, you know, when you fire a conventional battlefield weapon you don’t have human control of that right up until the point it hits the target in many situations. And so we’ve willingly chosen to limit the application of machine learning beyond areas that would be acceptable within a conventional system and I think that reflects the need to gain experience and understanding of these applications. Whether we can continue to hold onto these restrictions and follow these restrictions against an uncertain future with adversaries using equipment that may be guided and equipped using the technologies, I think remains to be seen.
Sean: Yes, apologies for the guess. I tried to get you to predict the future there. I mean, just to move slightly to move one side of that, I mean, obviously we talked about some advanced weapon systems and people have this kind of movie trope of the killer robots, Skynet, Terminator and everything, but moving to one side away from that, one of the real problems with AI in defence is things like computer viruses, worms, this sort of approach, this sort of attack rather. Viruses and worms have been a problem since the 1980s, but with AI powering it, is that going to be an even bigger problem? Or is it a bigger problem already, you know, these sorts of attacks?
Chris: Yeah, I mean there are machine learning algorithms that drive fuzzing attacks which are basically generating input sequences to try and expose vulnerabilities of a target application, and that, you know, that goes on in a broader security context and also criminal. So it’s not necessarily so closely associated with defence. But it’s definitely within the intelligence and security community and also the criminal community a greater topic of concern and there are already well-documented cases that using training sets of previous vulnerabilities and perturbing those using machine learning algorithms has exposed new vulnerabilities. So yeah. But it, you know- The phrase the arms race is an appropriate one in this context. As fast as people are using machine learning in an offensive context, people are developing new ways of applying machine learning in a defensive context and, you know, the use of adversarial machine learning as a way of improving the quality of your own software is routine now amongst larger companies.
Sean: That’s just cybersecurity.
Chris: Yeah, well its not- You know, it is really, really important and we shouldn’t downplay it. But we should anticipate the challenges of cybersecurity will be with us for the rest of the foreseeable future and that we need to be aware of the implications of that. I mean, within the military context, people have been using machine learning to study network traffic and then try to identify whether a system has been compromised if unusual patterns of traffic are seen on the networks and that sort of technology can be deployed just as it would be in the civilian context without raising many of the ethical questions that you- And we’ve discussed in the last half an hour.
Sean: One of those things it just brings to mind is the kind of unstoppable nature of the internet, you know? All of the countries, well, all of in inverted commas, there are obviously some exceptions, but the countries of the world that are connected to the internet despite being at war with each other, there’s still that kind of technological path through. Is that something that, you know, is an issue? I know there are, as I say, exceptions to that. Things like the great firewall of China, have I got that right? I don’t know. There are exceptions, North Korea being another one. You know? But places are all connected together so there are kind of paths between them.
Chris: Yeah, but I would anticipate again, you know, I would anticipate that the future may contain a greater diversity of network protocols. The internet protocol is simply an address scheme and a way of passing data from one machine to another but youcan layer things on top of it using Tor or using technologies from the dark web and these other geopolitical information infrastructures I think will see an increasing partition of information technology potentially into the future, both for the integrity of systems but also for the political control that we see around the globe.
Sean: Well it’s interesting that you mention that because I was thinking also about the idea of kind of one of the other AI problems we’ve got is the misinformation and the kind of that sort of, I suppose, phishing attacks and things like that are all kind of vectors aren’t they, for attacking?
Chris: Yeah, I mean, behavioural science is a key component of both offence and defence in cybersecurity and you know, I think that the UK national cybersecurity strategy makes it really clear that this is an all of society concern and that, you know, we need to, as far as possible, protect all areas of society from the threats that emanate in a changing cyber landscape, and ideally, the defence should require minimal intervention from members of the public. But ultimately, in the situation that we’re in now, there’s a strong need to educate people about the nature of the threat without introducing paranoia that undermines the benefits that we get from connectivity and digital literacy. So it’s a really difficult balance, and I think we see that most carefully- Or most clearly worked out in the education that we provide to schools. You know, on one hand, we want to inform the next generation about why antiviral systems matter and what the threats of impersonation and phishing are, but at the same time we don’t want to make people so paranoid that the next generation is reluctant to embrace technology. So it’s a difficult set of challenges. But if we want to be a digital nation into the future, they’re the things that we need to care about and spend time and energy educating all sectors of society in an appropriate manner.
[00:20:34]
Sean: It reminds me a little bit of the campaign for getting people aware when nuclear was a threat, you know, all those decades ago and you know, duck and cover and, I don’t know, the Young Ones parodying it of paint yourself white and all this sort of stuff. But you know, not to make too light of it. How is TAS- Or how has TAS been kind of working on, you know, some of these problems and what’s the TAS hub been doing in this area?
Chris: The Trustworthy Autonomous Systems community have been developing technologies like the counterfactual arguments that I described before and more complicated mathematical approaches to improving confidence and these are the foundations that we will need to really address the challenges going forward. And similarly, as you mentioned, in the use of psychology and behavioural science, both in offence and defence, you know, these are key concerns of the Trustworthy Autonomous Systems community. And really, while I think government and the military have done really excellent work in explaining the importance of ethics and also the UK’s attitude to machine learning and defence, at some point there’s a need to back up high-level philosophical concepts like proportionality and discrimination which involves, for instance, not targeting civilians, somebody has to be able to provide the technology that demonstrates we can do this. So, there are just a vast array of projects and programmes most of which are not purely military but they develop applications in those domains that will keep us and our future generations safe in a world with uncertainty about how machine learning will be used not only by our friends but also by our enemies.
Sean: How do you see this research going forward into the world of kind of responsible AI in, you know, the next few years?
Chris: I think that there’ll be a greater consensus about the approaches that can be used at scale and also a very specific example would be we want to use machine learning often because we can retrain. So if something in our environment changes or we want to optimise or achieve some new element of performance, we can take a new training set or extend an old training set and our system will rapidly reconfigure, even if we don’t understand the potential differences that the system is paying attention to, and so kind of the ability to cope with change and complexity are the key operational benefits of machine learning. However, every time we retrain, there’s a danger that, for instance, the resulting algorithm as we described before is in some way unfair or victimises a certain portion of society in a way that was never intended, and so what that means is in conventional software, we can test and retest every time we alter the software. In machine learning, we have to increase our confidence every time we try a new training set and so there’s a kind of a compromise between trustworthiness and flexibility and change in complexity, and I really hope that the Trustworthy Autonomous Systems community enables us to have scalable means of coping with change and ensuring principles, ethical principles like fairness, that discriminate or distinguish what our society believes in compared to others. It’s about providing the tools, the technical tools that enable us to uphold the values that distinguish us from others.
Sean: That’s a great point there. One final point, if you’ll permit me, one thing, again, that is mentioned in the UK’s approach to this is the problem- We have mentioned it a little bit in this podcast about unintended consequences, and as you just said there, when you add more data into a training set or you modify a training set and you retrain, you can’t always be sure and you literally just said it there about, you know, does it discriminate etc. Well you can‘t always be sure if there are unintended consequences that are going to come out of any changes and with traditional software, as you mentioned, you run tests. I mean, is that something you can do with machine learning? I mean, can you automate some tests that, you know, you put the system through and make sure that, you know, it hasn’t changed dramatically in the wrong way?
Chris: Yeah, and that’s- Absolutely that’s a focus of the Trustworthy Autonomous Systems’ work is identifying appropriate test cases. But to be honest, there’s kind of catch 22 which is that you choose to use machine learning because there is uncertainty in the environment and very often, the unintended consequence arises from something that you hadn’t anticipated at the start and, you know, if you were to look at, say, autonomous vehicles and the millions and millions of miles that have been driven to train those systems, and yet even so, unexpected things happen when those vehicles are confronted with pedestrian behaviours. For instance, breaking road traffic rules in a way that wasn’t predicted by the designers. And so, I think there will always be unintended consequences so there has to be as rigorous and approach as possible, and that includes really intensive surveillance of the behaviour of the system when deployed into environments. And also, there may need to be, where machine learning is connected to a physical system, a greater protection and guarantees in the environment, you know, for instance interlocks or this human controllability that you referenced before within the UK military environment, that enable us to protect people from the side effects that arise from this use of technology.
Sean: Well that’s about all we have time for today. Many thanks for joining us today on the Living With AI podcast, Chris.
Chris: Brilliant, thank you for your questions, it’s been really thought provoking and it’ll be really interesting to look back in 10 years and see where we are.
Sean: If you want to get in touch with us here at the Living With AI podcast, you can visit the TAS hub website at tas.ac.uk, where you can also find out more about the Trust for the Autonomous Systems Hub. The Living With AI podcast is a production of the Trustworthy Autonomous Systems Hub, audio engineering was by Boardie Limited and it was present by me, Sean Riley.
[00:28:02]