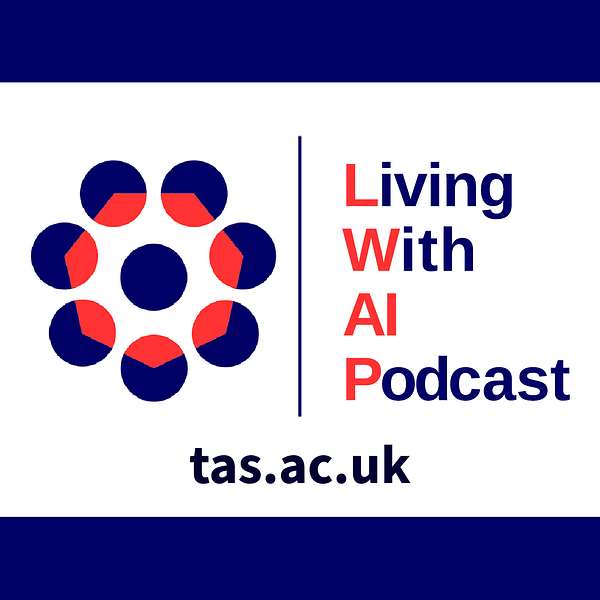
Living With AI Podcast: Challenges of Living with Artificial Intelligence
Living With AI Podcast: Challenges of Living with Artificial Intelligence
AI & Science, Innovation and Technology
Does academia lead and industry follow? Or are there breakthroughs made on both sides? How siloed is AI innovation? We discuss some of the Innovate UK initiatives to bridge industry and academia.
Podcast guest:: Trias Gkikopoulos, Innovation Lead - Robotics & Artificial Intelligence at Innovate UK
Podcast production by boardie.com
Podcast Host: Sean Riley
Producer: Stacha Hicks
If you want to get in touch with us here at the Living with AI Podcast, you can visit the TAS Hub website at www.tas.ac.uk where you can also find out more about the Trustworthy Autonomous Systems Hub Living With AI Podcast.
Podcast Host: Sean Riley
The UKRI Trustworthy Autonomous Systems (TAS) Hub Website
Living With AI Podcast: Challenges of Living with Artificial Intelligence
This podcast digs into key issues that arise when building, operating, and using machines and apps that are powered by artificial intelligence. We look at industry, homes and cities. AI is increasingly being used to help optimise our lives, making software and machines faster, more precise, and generally easier to use. However, they also raise concerns when they fail, misuse our data, or are too complex for the users to understand their implications. Set up by the UKRI Trustworthy Autonomous Systems Hub this podcast brings in experts in the field from Industry & Academia to discuss Robots in Space, Driverless Cars, Autonomous Ships, Drones, Covid-19 Track & Trace and much more.
Season: 4, Episode: 8
AI & Science, Innovation and Technology
Does academia lead and industry follow? Or are there breakthroughs made on both sides? How siloed is AI innovation? We discuss some of the Innovate UK initiatives to bridge industry and academia.
Podcast guest:: Trias Gkikopoulos, Innovation Lead - Robotics & Artificial Intelligence at Innovate UK
Podcast production by boardie.com
Podcast Host: Sean Riley
Producer: Stacha Hicks
If you want to get in touch with us here at the Living with AI Podcast, you can visit the TAS Hub website at www.tas.ac.uk where you can also find out more about the Trustworthy Autonomous Systems Hub Living With AI Podcast.
Episode Transcript:
Sean: Welcome to the Living with AI podcast from the Trustworthy Autonomous Systems Hub. This is Season 4 of the podcast, so there are plenty of back episodes in Seasons 1 to 3 if you want to go and check those out. I’m your host, Sean Riley, and shortly will welcome our guest for this episode, Trias. We’re going to talk about science, innovation and technology today.
But let’s just first of all just get it out of the way that we’re recording this on the 9th of April 2024, so if there’s huge developments in May 2024, we’re not going to be talking about those because it’s the 9th of April 2024 as we record this.
Let’s welcome Trias Gkikopoulos. Trias tell us about yourself, what do you do and, you know, what organisation are you with?
Trias: Yeah, thank you, Sean, and good to meet you. I’m an innovation lead in the artificial intelligence and machine learning team at Innovate UK which is part of the UK Research and Innovation that are the Research Councils and Innovate UK as well.
Sean: Fantastic, and it’s great to have you on the podcast, appreciate you sparing the time for us. Science, Innovation and Technology, we’ve got this overarching title for this episode, massive field, where do we start? Can you tell us about the projects that you’ve been working on that are concerned with the Trustworthy Autonomous Systems Hub?
Trias: Yeah, so, part of my role is to try to identify opportunities where we can support industry and collaborations with academia to address sometimes market failures that might exist within the innovation ecosystem. And trying to support the translation of research from academia to the industry. So part of those activities are addressed with the BridgeAI programme currently in delivery. And part of this programme involves the responsible and trustworthy AI.
So what do we mean by responsible and trustworthy AI, and why that should be any different than other digital technologies. So, I think the key part of AI that makes it a bit unique and different from other digital tech is really the pace at which it is advancing. And it’s also different area enough from other tech to allow this new domain, what is called responsible and trustworthy to be formed to address really how we ensure that there are no harms made and it is used in the best impact in society.
So, the trustworthy bit is really to ensure that the people are being affected, organisations are being affected in the society can have tech and trust what the technology does. So, that means it has to be lawful. It has to be explainable, to be able to understand its actions. And responsible, I guess the responsible is with- In relation to the actors, organisations that develop and deploy the system. And it’s really their responsibility to ensure that they maintain it in the right way to ensure that, again, it is trustworthy, it does not harm and provides a positive impact in the society and economy.
Sean: And, obviously, if you’re bridging academia and industry, is one leading the other and the other catching up or is there progress being made on both sides and you have to keep the bridge going in both directions?
Trias: Yeah, I think that’s a very interesting area, especially in the last three years. When you look at where the advances are coming with respect to new technologies. So, obviously we see US companies producing new products that are performing, you know, beyond what we have been able to imagine probably a few years back. But at the same, of course, research is providing those fundamental parts for the mathematical science, logic and semantic to be able to continue to develop more tech in the future. So, yeah, it is an interesting intersection I think that we are at this moment.
Part of what we do at Innovate UK, and I should just mention, is really providing that bridge and links between academia and industry. And a lot of what our programme is trying to do at this moment is to- In addition to ensuring that we can translate the cutting edge, if you like, research from academia in to the industry for the benefit of new products and services, is also to accelerate that early part of the adoption and diffusion curve.
So, sometimes, what that means is that the technology that we’re looking to translate from academia might not be coming as early as [s/l GRL 00:05:23], I don’t know if you know the GRL levels, but it could also come as a consultancy support. So, looking at translation of the high GRL, again, with the help of academia in to the industry.
Sean: Not to go in to it in too much detail, but it seems to me the industry are able to throw a lot of money at these things. So, things like the generative Ais are being developed by throwing a huge amount of money at compute power, which academia doesn’t have. But academia hopefully has the brains to, as you say, come up with the ideas and maybe the techniques, as long as industry doesn’t poach all of the academics of course.
Trias: Yes. I think you touched upon a couple of very important areas. One is the talents, you know, it is a very scarce resource at the moment, especially in this domain. And the other one is- You mentioned industry putting a lot of money for us as investment, but actually there is also the utilisation of resources that comes together with that investment, for example, for the training of the very large language models. The consumption of what is required for the training or even for inference.
So, you’re absolutely right, it’s a very topical point and, of course, yes, academia doesn’t always have those resources to be able to compete with the Access to Compute. However, the UK government has committed a significant amount of investment to enable Access to Compute with more computers to the UK and help academics access the right amount of compute to be able to be competitive and continue to develop those academics.
Sean: I look forward to seeing that. I mean, you know, you did touch upon something there though which is kind of like the resources side of things and the fact that ecologically maybe this isn’t the best direction to be heading in, but anyway. I think if we step to the side of that, it’s slightly outside the scope of what we’re talking about today.
I was thinking a lot of automation tools get used to kind of speed processes up and to take repetitive tasks away from maybe fallible humans. AI is no different but what really big innovations are happening at the minute rather than just kind of taking on those mundane tasks. Is there anything that’s really big that’s happening at the moment that’s changing things or is it just the scale?
Trias: I would say it’s easy to overlook the fact that- What you just said, you said, you know, replace those mundane tasks, but actually that is not an easy task if you want to be able to make this repetitively and accurately. And the ability to automate tasks that are very much at a human level kind of capability, yeah, I wouldn’t overlook this at all.
So, the productivity improvements that can be achieved through a good utilisation and application of those general proportionate transformers, the LLMs, I think is probably one of the most transformational things, you know, we’re going through in terms of technology in the last few years.
And although I don’t believe that the GBT based AI will eventually lead to the general focus and general AI I do see that with increased complexity and a larger size we’re very much likely to see something that could be very close to the capabilities of human intelligence but it won’t be the real thing. I don’t think the actual general AI will come from GBT based transformers.
Sean: And I’m intrigued to know if anyone would necessarily know the difference though, that’s the problem, because it appears to act like it. Is that not the big problem working out if it is or it isn’t?
[00:09:41]
Trias: Yeah, no, absolutely. And I do agree. I think it would certainly pass the Turing test, for sure. But there would only be those cracks, I think, in the [s/l real 00:09:55].
Sean: And it’s interesting you say, it would, I think, pass the Turing test and I’m sure most of the listeners would understand what that is, but as I understand it that’s- If you had somebody talking to this machine and unable to distinguish it from a human. And we’re definitely at that point aren’t we, I think with ChatGPT and these different- You know, even some of the other large language models, again, at maybe significant ecological cost as well.
But that brings it round to something else, because it’s being used, or it’s seemingly being used for things like misinformation. So, there are trust elements there, aren’t there, because you can con, if you like, if that’s not too anthropomorphised, con ChatGPT in to doing things it’s not supposed to do and creating large amounts of misinformation, for instance.
Trias: Yeah, that’s right. And I think this very part has been picked up and was one of the reasons for the objective that the AI Safety Institute is set to achieve. I think very clearly the UK government has identified that there is a large potential for risk coming from what they call Frontier Systems, part of those, the general purpose, [unclear 00:11:10], the LLMs, the ChatGPT. And exactly for the points that I think you mentioned, the ability to manipulate and I think in a general scale as well. And obviously, you know, we also have the vulnerable groups that, again, they need more protection and, obviously, they would be very susceptible.
So, yes, a big part of the priorities is really to understand more the systems. Understand how they could potentially be used to do harm and place regulations and safeguards in place in order to ensure that this doesn’t happen. But, yeah, very interesting to see what comes from the work that will start to be delivered from the Safety AI Institute, but of course from other domains of AI innovation ecosystem.
To give you an example Innovate UK has invested £21m to support innovations in the responsible and trustworthy space. This has come from a number of different markets looking from financial assistance to health and agriculture and from a number of different technologies looking at explainable AI. Looking at [unclear 00:12:47] Euronet and different AI assurance methodologies.
So, yeah, it’s a very intriguing, interesting, fascinating- And I think that space of AI assurance responsibility, trustworthiness and safety is going to advance, I think, as fast as the rest of the tech. I think that there is a requirement for incentivisation, again, because obviously, if we leave the market in its own accord we are likely to gravitate towards the obvious market solutions and the obvious tech development. So, yes, some incentivisation is required but I believe it is there and the investments are there as well, I mean in addition to Innovate UK, EBSOC and other parts of UK AI are also investing in responsible and trustworthy AI. The TAS Hub that you mentioned earlier, being one of them, of course.
Sean: And I’m glad you brought it back round to Innovate UK, you know, I wanted to talk a bit more about the project you mentioned before, BridgeAI, and ask about some of the challenges you faced, you know, when you- Is BridgeAI complete? Is it still ongoing? Where are you at with that?
Trias: Yeah the BridgeAI is currently one year in delivery, so it’s a very new programme. And it’s a programme that sought to mobilise the actors of the innovation support ecosystem. So, what I mean by that is organisations like the Alan Turing Institute and the Digital Catapult, STFC is part of the Hartree and [s/l Trading 00:14:31] Standards institutions. They all have unique strengths. They all do interfere and play with ethical AI and general AI technologies as well.
So what the BridgeAI programme sought to achieve is to create a collaborative support mechanism that includes the strengths of all those different players and try to address and lift the barriers currently limiting adoption of artificial intelligence and machine learning in the UK, economy.
I can almost hear your question, why should we be investing more money to help the private sector adopt artificial intelligence and machine learning, and I think the reason is really around market failures. It is really about pinpointing and identifying the right tool for the right reason. And in this particular case we have seen, based on a number of different evidence, that because of the impact of COVID, because of the impacts of the EU exit, and because of inherent qualities with different industries, the artificial intelligence and machine learning technology was not being adopted and diffused at the same rate at different sectors.
I think this is very important, and it is very important also not because this entails a classical market failure from an economics standpoint point of view, but also what has been happening the last maybe five or six years, there has been a trend that shows that there is an acceleration in the divergence between those firms that can adopt technology and may create a better economic environment for themselves and become more profitable and those that actually cannot really adopt as efficiently as the other firms and then the economic impact is much worse to them.
And this divergence has been observed at firm level, at sector level and also even if you change and move up a scale, at a national level as well. So, it’s an interesting phenomenon and I think it really has to do with the ability of exploiting the best use of those resources. And, you know, as we are becoming more digitally and AI driven economies, the ability to have access to those on the edge tech gives you even more and more advantages.
Sean: And you touched upon something there that I was wondering about. Presumably there’s an economy of scale here. I mean is there some kind of pooling that can happen, you know, multiple firms using a similar kind of, I don’t know, model for instance?
Trias: Absolutely, I think there are use cases where they will see the benefit of multiple firms from this tech and obviously, yes, we’ve been talking a lot about large language models. There has obviously been a huge appetite to be used across market use cases. If anything, maybe we are falling in to the trap of trying to use a tool just because it’s easy to try to solve everything. This is not unique to AI or even Large LLMs. I think a few years back when the drone industry was exploding we saw exactly the same thing. Drones were attempted to be used to solve every imaginable problem that they could possibly solve regardless of whether the actual economics made sense or not.
Sean: Yes.
Trias: Having said that, I think the more powerful technology and innovation then the more likely that you will see another investment. This also happened, for example, with other general purpose technologies. This happened when we were building [s/l Warwick 00:19:08] canals. There was a huge investment and what that huge investment was followed by bankruptcy across some of those investors. But, it is a period where- What normally happens is that, that increase in investment from different actors who try to capture value, is followed by the inevitable saturation of that market. But I believe it is something that we require to go through in order to see us ourselves successful from the other end.
[00:19:40]
Sean: Yeah, I understand what you mean, it’s like something shiny appears and everybody thinks, ah, something new and shiny, let’s try and use that for our means but it might not be the best tool for the job.
I mean talking of tools for the job, there was an article last year in Nature called Scientific Discovery in the age of Artificial Intelligence. And basically I think it all boiled down to the fact that this is just yet another tool and it’s got to be used in the right way because you can, you know, you can use any tool in the wrong way.
And the other thing that kind of was clear from the article is that bad data in equals bad results, you know, garbage in, garbage out, which I don’t think is new in anything to do with technology, it’s probably not new in anything to do with human nature in general. But how do we start to approach this, you know, scientifically to try and, you know, fix these problems, I suppose?
Trias: It is part of the broader responsible and trustworthy AI. So, I think the data science that underpins what is happening with AI is important. Again, we tend to focus on one specific technology, which currently seems to be large LLMs, because they’re extremely useful. But we’ve come a long way and I think that it’s also another huge amount of different techniques that are available within machine learning, and more yet to be discovered. So, I think, yeah, it is important to bear that in mind.
When it comes to what we expect to see in the future and trying to address that data quality in terms of how is this being utilised, I think we are likely to see, again, more tools that do an evaluation of the early stages of training and developing a new programme to assess the quality of this data to be used. Again, I think this is another area of interest that we’re likely to see more growth in the future. And, yeah, you’re absolutely right, bad data in, bad results out, I think that’s inevitable.
Generalisations perhaps is something that AI systems are not good at doing, are there systems to be able to do right now, but on the other hand humans are probably doing a bit too much of this.
Sean: There’s that, exactly, yeah. And the other thing that comes up time and time again on the podcast, and maybe it’s worth mentioning here, is the bias that is inherent in data sets, which, again, as you say, is also inherent in humans as well. I mean that’s another thing that’s something we have to try and combat, isn’t it?
Trias: Yes. This is also, yet again, another part of the responsible and trustworthiness ecosystem. So, as we use more and more systems that have the ability either to self-learn, evolve or do change through more feedback from the developers or the end-users who are using it. So, it is important to be able to monitor how the suggestions made are produced by that system, change and drift. So, what that means is that the decisions could improve or at the same time that’s not really- This is not really a definite one direction here.
So, part of systems that end techniques and architects have been used as a test really, is things such as the machine learning ops, which involve using a number of different types of metrics in order to continually evaluate things like you mentioned, the bias, accuracy the time that a model takes to infer its result. So, while this really provides that robustness to the actors and the organisations who maintain the systems in ensuring that they do, and they continue to, deliver what they are meant to.
What I have personally seen based on the engagement with different organisations, is that companies that are working in the health sector are much better both from understanding the data science that underlines the techniques that they’re using but also in putting together the right methodologies to ensure that they serve robustness and the understanding of bias on any model drift within the system is well accounted for. Because, obviously, the consequences are high and at the same time they are required from the regulatory environment to be that accurate and accounted for.
And I see where there could be a little bit of risk when it comes to the approach that we are taking in regulating or non-regulating systems based on the potential risk that there is perhaps a concern that for those applications that are not perceived to be high risk then there will be less incentive for those developers working on those models to ensure they have the same robust architecture and performance as those on the higher risk applications.
Sean: I think also, as you mentioned health, you know, they’re probably a little bit more used to having those kind of processes in place which, you know, are traceable. I’m thinking of the idea of AI being sometimes somewhat a black box, that actually how do you know which developer was responsible for which. And I don’t mean from a blame point of view, but as much as anything from a re-training, from a fixing, from a repairing, from a fault finding point of view. It could be quite challenging that, can’t it?
Trias: Yeah, you’re right and what that means is quite often even if a system is a black box, which essentially sometimes it is, there can be methods in order to be able to identify, again, how parts of the system will behave when they are encountering new types of data. So, again, it’s a matter of having the methodologies in place to be able to monitor the best you can and have the accountability in place to test performance of those. However, what I’ve foreseen- I think it goes back to a little bit what we were saying earlier about a new kind of technology that is being used to try to solve everything. Not only I think this is true, but often because of, again, the pressure perhaps from organisations trying to make sure they can make the most out of these technologies in order to get a match in the market, often, or sometimes they might not consider the nuances of the architecture of complex systems, again, in a way that we are trying to use- For example, two years back we were trying to use convoluted neural nets to do optics identification and in general convoluted neural nets not only try to address everything or every problem that we have, to the extent that- I remember there was a paper that was trying to analyse cyber security of Wi-Fi networks. In order to ensure that we can make sure of using convoluted neural nets, they transformed the signal strength in to a [unclear 00:27:59] and then they pushed this through the modals to do the analysis, which, of course it works.
Sean: But, it’s- Yeah.
Trias: Having said that I think what we also saw, things- For example, there was one of the famous cases of the, unfortunately, fatal accident with one of the early autonomous cars back in 2019. Again, the failure of a particular sub-system in this particular case, it was an AI system. It was an object identification. The failure of that classifier to identify in time, or correctly, a particular object should not be the cause of having that failure in the system. And really I think addresses the point of whether we have the right software engineering tools in place. Whether we have the right considerations for the design of complex systems and, again, not over-relying on a single particular technology in order to solve all the problems within this context.
Sean: Yeah. I think there is definitely a danger of the marketing department leading the developers down a certain path for, yeah, for [s/l eyeballs 00:29:17]. I mean, you know, this is not new though is it, you know, a few years ago it was deep learning, a few years before that everything was Cloud based. And of course these technologies still exist and are still useful in certain fields, but, as you say, we’re all talking about generative AI. So, everything has to be generative AI based when actually it might not be the right tool for the job.
I’m going to ask you to put your kind of magical future seeing hat on now, what do you think we’re seeing in a few years’ time, what does the future hold?
[00:29:44]
Trias: Yeah, I’m very bad in predicting the future I shall say. But one thing I can predict that is going to be fairly exciting, and, again, we are likely to see, even faster advances in the ability of AI to produce results that, once again, we will not be able to predict.
So, I don’t know if you’ve seen the outputs from the multimodal system by OpenAI and its ability to create a video from text prompts, it does really blow one’s mind in the ability to produce the outputs that they’re doing.
In where we’re moving to at least for what appears now- I think we are moving slowly towards the ability of there being- Around AI assisting. And I think you can see this also from how Microsoft is branding their own AI tool. And it’s really a natural next step from where we are now with the LLMs.
And once we are able to get a bit more logic and reasoning inherent in those systems, and, again, this is not likely something that’s going to come through more training. I think we need to consider the development and creation of the hybrid tools that are used, LLMs, semantic graph networks in to- Again, thinking about complex systems, it’s not just one solution, it’s about how we best use the right tool for the right job in the right way to produce the desired outcome.
So, I think it’s not going to be too long before we see something- We have the support from a computer, like in Space Oddity. So, maybe a few years down the line, I tell you what if Innovate UK allows me to use it, it will make my job so much easier.
Sean: I’m slightly concerned about the open the Bombay doors, how, inevitability. But then, you know, there’s problems with all sorts of tech, you know, at some point tech goes wrong, it, unfortunately is the case isn’t it, for the millions of people’s lives that it makes better. As long as we can trust it, I think that’s the main thing. I know in researching this I realised there was a report that came out last year, was that something that your team had something to do with? Can you tell us about that?
Trias: Yeah, that’s right. So, part of the activities of the BridgeAI programme and understanding again, that responsible and trustworthy AI is not really a sole responsibility for the developers of the tech. It’s not the responsibility for the commercial users, it’s really a holistic approach, all the different actors are being affected, are being developed need to consider and need to understand what are those different areas that create the response contrast with AI.
So, what we did, we wanted to create a report that provides the core principles of responsible and trustworthy AI and allows the different actors to develop a common language that would allow them to easier communicate about this topic. It can be very difficult when we have new sub-domains of science and technology, you know, evolving. There is the new link that has been created, that is not really necessary across different areas of public life. So, yeah, we did develop this report with help from [unclear 00:33:46] research. It is available on the BridgeAI.net website. And I’ll be sharing with you a link if we can make available to your listeners.
Sean: Yeah. We’ll put that in the show notes, that’s no problem at all. Trias it’s been fantastic to have you on the podcast, I appreciate you sparing your time, thank you very much for being on the Living with AI Podcast.
Trias: Thank you, Sean, it’s been great to be with you.
Sean: If you want to get in touch with us here at the Living with AI Podcast, you can visit the TAS Hub website at tas.ac.uk where you can also find out more about the Trustworthy Autonomous Systems Hub. The Living with AI Podcast is a production of the Trustworthy Autonomous Systems Hub, audio engineering was by Boardie Limited and it was presented by me, Sean Riley.