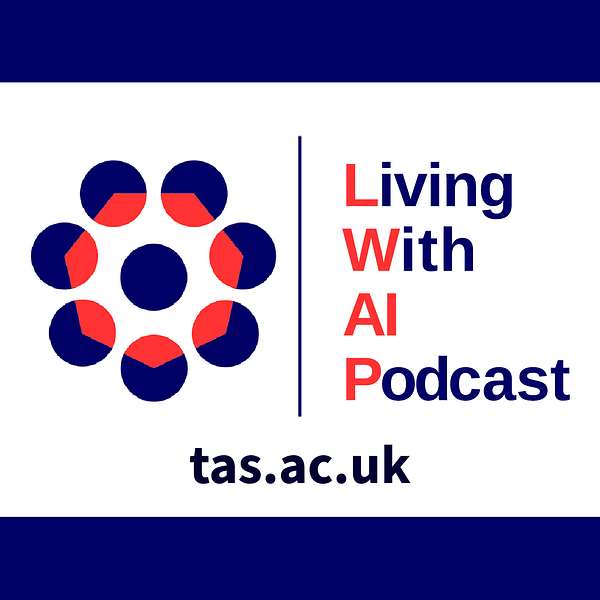
Living With AI Podcast: Challenges of Living with Artificial Intelligence
Living With AI Podcast: Challenges of Living with Artificial Intelligence
Health & The Reformist Project: Mirrored Decision Support Framework for Multidisciplinary Teams in Oesophageal Cancer
The Reformist Project is developing an AI support tool to speed up decision making in critical healthcare situations. Use of this could revolutionise the necessary meetings where different clinicians assemble to plan patient care.
Guests on this episode:
Ganesh Vigneswaran, NIHR Clinical Lecturer in Interventional Radiology, University of Southampton
Tim Underwood - Professor of gastrointestinal surgery, University of Southampton
For more information on the project: REFORMIST: Mirrored decision support fRamEwork FOR Multidiciplinary Teams in Oesophageal cancer – UKRI Trustworthy Autonomous Systems Hub (tas.ac.uk)
Podcast production by boardie.com
Podcast Host: Sean Riley
Producer: Stacha Hicks
If you want to get in touch with us here at the Living with AI Podcast, you can visit the TAS Hub website at www.tas.ac.uk where you can also find out more about the Trustworthy Autonomous Systems Hub Living With AI Podcast.
Podcast Host: Sean Riley
The UKRI Trustworthy Autonomous Systems (TAS) Hub Website
Living With AI Podcast: Challenges of Living with Artificial Intelligence
This podcast digs into key issues that arise when building, operating, and using machines and apps that are powered by artificial intelligence. We look at industry, homes and cities. AI is increasingly being used to help optimise our lives, making software and machines faster, more precise, and generally easier to use. However, they also raise concerns when they fail, misuse our data, or are too complex for the users to understand their implications. Set up by the UKRI Trustworthy Autonomous Systems Hub this podcast brings in experts in the field from Industry & Academia to discuss Robots in Space, Driverless Cars, Autonomous Ships, Drones, Covid-19 Track & Trace and much more.
Season: 4, Episode: 2
Health and the Reformist Project: Mirrored Decision Support Framework for Multidisciplinary Teams in Oesophageal Cancer
The Reformist Project is developing an AI support tool to speed up decision making in critical healthcare situations. Use of this could revolutionise the necessary meetings where different clinicians assemble to plan patient care.
Guests on this episode:
Ganesh Vigneswaran, NIHR Clinical Lecturer in Interventional Radiology, University of Southampton
Tim Underwood - Professor of gastrointestinal surgery, University of Southampton
For more information on the project: REFORMIST: Mirrored decision support fRamEwork FOR Multidiciplinary Teams in Oesophageal cancer – UKRI Trustworthy Autonomous Systems Hub (tas.ac.uk)
Podcast production by boardie.com
Podcast Host: Sean Riley
Producer: Stacha Hicks
If you want to get in touch with us here at the Living with AI Podcast, you can visit the TAS Hub website at www.tas.ac.uk where you can also find out more about the Trustworthy Autonomous Systems Hub Living With AI Podcast.
Episode Transcript:
Sean: This is Living With AI, the podcast from the Trustworthy Autonomous Systems Hub. Today, we’re tackling health. I’m your host, Sean Riley, and this episode will be a hybrid because we’ll look at heath in relation to a TASO project called Reformist: Mirrored Decision Support Framework for Multidisciplinary Teams in Oesophageal Cancer. We’re recording this on April 12 2024. Let’s welcome our guests for today, Tim and Ganesh. Thank you for sparing some time for Living With AI. First of all, I’ll just get you both to introduce yourselves. So we’ll start, just because you’re on the left of my screen Ganesh, we’ll start with you. Ganesh, tell us, what’s your name and what do you do?
Ganesh: Yes, I’m Ganesh Vigneswaran, I’m a clinical lecturer in interventional radiology and I spend half my time doing research and half my time in the interventional radiology lab.
Tim: Hi, I’m Tim Underwood, I’m professor of gastrointestinal surgery at the University of Southampton. Like Ganesh, I do half my time doing academic work, research, and trying to build AI models and that sort of thing, and the other half is spent operating on people who have cancer of the oesophagus among other conditions. So intimate relationship with the patients and then bringing the problems from the patients to the academic side to try to answer them.
Sean: Super. Well thank you so much for both of you to spare the time to be on the podcast today. We really appreciate it. Is it possible one of you could give us just an overview of the project and you know, what you’re trying to achieve? Because I believe it’s ongoing isn’t it? What’s the project about?
Ganesh: So oesophageal cancer is a devastating condition with a very poor prognosis. But decision making for these patients is critical. So classically, decision making is done in the form of a multidisciplinary team, that’s where a bunch of individuals, specialists, come together every week and they basically have to determine what the best treatment decision is for that patient. The best decision going forward for the management of that patient. And you can understand that that can be incredibly complex and so I think, you know, the MDT has been under this incredible pressure, especially over the last decade or so where it’s almost doubled in terms of workload capacity, but our workforce hasn’t matched that. So what we kind of wanted to do was use AI to see if AI or machine learning could have a role in improving these MDTs, standardising these decisions and providing some health equality and perhaps streamlining these workflows.
Tim: Yeah, just to add to what Ganesh has said, this is- It part stemmed from a series of questions that we were asking ourselves during the MDT meeting. Like Ganesh says, an ever-increasing workload and you’re trying to fit more and more patients into a finite amount of time, so how could you streamline that? That is one thing. But also I became interested in possible disparities in decision making from week to week, determined by who was in the meeting and what night sleep you’ve had the night before, and the performance factors that go around being a human being, and whether or not we’re making the absolute best decisions all of the time. And let’s ne honest, we’re probably not, because we’re humans. Could we strengthen that by deploying a decision support tool that is data driven? So could we make these decisions better for the individual and better at a population level too?
Sean: So it’s more objective, there’s a bit more consistency. Because by the sounds of it, continuity’s a bit of an issue as well isn’t it? So having some kind of, well, system is going to help no end, isn’t it?
Tim: Absolutely. From a practical, pragmatic point of view, I might be away for two weeks on a conference and when I come back for the next week I am then present in that meeting. But for the two weeks I’m not there, my colleagues have had to take the slack, and then equally, they’ll be away and then I’ll be there and we might have a different oncologist and a different radiologist, because that’s the way we work in teams. So how do we get- We try to be consistent and we’re doing our absolute best. But how can we support that to be even more consistent? To give the best decisions for the right patient at the right time?
Sean: That’s great to hear. I mean, in a lot of other areas we hear the- I don’t want to be flippant about this, we hear the phrase it’s not life or death, but of course here it actually is life and death and it’s really, really important we get this right. How do we trust an AI system though? You know, the overarching idea of this podcast is trust AI, have you got some kind of percentages, numbers on how much better it would be working with or without, or are you waiting for the end of the project for things like that? How do you measure it and how do we trust it? Sorry, lots of questions in one sort of spiel there. Ganesh?
Ganesh: Yeah I think you’ve got very important questions there. I don’t think I’ve got all the answers for you I’m afraid, but I think we’re getting there. I think it’s about finding out what that balance is and I think Tim and I have had a chat about this yesterday, it’s about finding what we would consider is okay in terms of a decision, whether how much, you know, whether we can trust that decision or not. We don’t know what part of the operating curve that we’re going to be working on. So yeah, I think it’s difficult to know what exactly the number you’re going to say, like, oh an AUC of more than 0.8 is great and 0.75 is not good enough. We haven’t got there yet. But I think we’re in a position now where we can start to test that without models which are showing some real promise.
Tim: And one of the things we’ve been really careful to do is to allow clinicians in particular, because clinicians are going to use this initially, to understand how the support tool might be making its decisions and how that corresponds to how human beings are making their decisions. We know intuitively how we make a decision. We look at the patient, we see them in the clinic and we say they’ve git a range of comorbidities, they might have a heart problem or they’ve got diabetes or they’ve got lung problems. That puts them at higher risk for a variety of things that we might have to do with them. We’ve then asked the model, as we’re building it, what characteristics of this patient is the model using to make its decision? And we can then say to the clinicians, look, it’s using similar characteristics than us, but it’s using them robustly. It’s using them consistently. And it’s giving a, what seems to be, an accurate decision based on similar things that we would use. So actually, you can trust it and you can trust it to run alongside your gut intuition. There is- I just mentioned something that’s really important, there is a clinician gut intuition that I’m not sure a model like this will ever get. But we’ll see.
Sean: It’s probably very difficult to define, right?
Tim: Nearly impossible. But you kind of see a patient case and go I’m just not sure, or you’re much better than you look and we’ll give you a crack, and that sounds odd, but that is what really experienced clinicians do.
Sean: I’m going to state the obvious here that you’ve just laid out some of the problems with implementing a system to diagnose or to give a treatment plan which is that there are underlying conditions, there are complications, there are all sorts of things that you are trained as clinicians to deal with that computer systems don’t usually have to deal with. You know, we have the classic computer says no on the comedy sketches, the mortgage application etc, without taking into account any others. Is that something that you’ve come into, have you had to make it more and more and more complicated to add more and more detail? How does the system deal with those sorts of things?
Ganesh: So I think it’s important to stress that this is a decision support tool that we’re making. It is your 12th man. It is the sense checker in the MDT. At the end of the day, it’s still going to be a clinician, human based decision, but what we want is that confidence, knowing that this is what we would have done last time. And, you know, when you get a conflict, where the machine might give you a different prediction to what the team wants to do, I think it’s a moment to pause and to reflect and to work out whether what you’re doing is just right. It’s a sense check. So I think you need to be really careful to make sure that we- That this is the ballpark that we’re putting this tool in. We’re not trying to replace the doctor or the team.
Tim: If we go back to one of the reasons we talked about doing this, which is streamlining the process of the MDT meeting, one of the things that this could be really powerful for is to say we have a group of patients in the MDT who it’s clear what the decision’s going to be. The AI machine, the AI says this. We agree with it. They’re straightforward, they can take two minutes of your time. Where we can then focus our energies is on the patients where it’s not clear. Where the AI tool isn’t clear. Where we’re not clear. Where there’s conflict amongst the clinicians. And then we can spend five minutes, ten minutes, 15 minutes, the appropriate amount of time on that particular complicated case. That would be really powerful. Streamline the MDT so that we focus our energies on the right people.
Sean: That makes a lot of sense. I mean one thing that we found in other areas, other spheres, is that when people think of an AI system, let’s take autonomous driving, people expect it’s going to be eons better than the human equivalent, when actually, perhaps all we need is to be as good as the humans. Is that something that you’ve found in the health area?
[00:09:28]
Ganesh: Absolutely, I mean I think the benchmark for an AI system is much higher than humans essentially. We need it to be better, we need it to be more accurate, we need it to be making no mistake because we always feel that, as humans, we will always make the right decision even though that might not be the case. And I think it’s just about delegating that responsibility, isn’t it, to another system? When you can take ownership and responsibility as a human, then you say well my bad, I got it wrong. But we can’t let the computer do that.
Tim: So I find this whole thing fascinating. We talk about- Take the car example, right? I know nothing about autonomous cars, right, but I’m going to come at it from a personal perspective, right? We get really obsessed about the autonomous car can’t make a mistake, yet human beings crash their cars all the time. In fact, we’re atrocious at driving in general. We’re just atrocious at it. We’re abysmal. And yet we won’t trust a car because one of them might have crashed once in ten billion road journeys. I mean, it’s just- Somehow human beings have got to say have we gone mad? You know?
Sean: It’s a control thing though isn’t it?
Tim: Absolutely. Yeah it is.
Sean: People want to think they’re in control and when a black box is doing something for them, that’s a worry for them.
Tim: So the other day I was on an aeroplane and it was coming in to land and it was foggy and the pilot said we’re going to use the Autoland system to land this plane because I can’t see the runway and I thought to myself thank the lord that they’re using the Autoland. Because if he can’t see the runway, and it was a he, then I don’t want him to land the aeroplane. I want the aeroplane to say there’s the runway, I can see it. I’m going to land on it.
Sean: Yeah absolutely. Well, we’ve had on the podcast before Professor Des Gupta who’s a leading light as a surgeon himself and he’s said that he’s found that patients, when they’re doing that pre-surgery chat in his field, which is urology, he’s found that people would prefer to have the robot assisted surgery. So that’s obviously something that’s happening and has been happening for years. So AI, I mean, just going into a wider sphere here, AI’s helping clinicians across the board isn’t it? Just stepping outside your project at the minute. Have you had any other experiences with AI in your field, in the field of health?
Ganesh: So I can speak for radiology. So at Southampton, when any patient is coming in with a stroke they have a CT head and they have a CT angio which shows the blood vessels of the brain and see if there’s any blockages and we automatically get those images analysed and it kind of highlights areas where there’s a blockage and interestingly I’ve been on a night shift or two where you’re really, really tired, it’s 2 am, you’ve picked up the CT head and you’ve looked through and thought oh, it looks normal and then you wait and you say oh, here’s the PDF just popping through on PACS which is our imaging system and it shows you and it says blockage, left MCA, distal branch and it’s put a little circle around it and you like open the images and scroll through, oh yeah, it looks like there is a blockage there. So I think these technologies do have a role to play, you know? Whenever we have these, you know, whenever you examine these publications, it’s always the best case scenario, it’s always the best radiologist who’s got 15 years experience and that versus the AI. But what about the trainee? What about the night shift? What about when you’re hungry and you’re tired? Those are scenarios where the AI system is going to have benefit and I don’t think we’re realising that yet.
Tim: I’ve got an example from our research work where we’re actually- What Ganesh is alluding to there is that the AI computer vision stuff is where AI has a real advantage. I mean, we don’t see the patterns in the images as well as machines can do because we can’t analyse the images that quickly and assimilate the information. So in the research world, where there’s now good examples of AI being used to look at pathology slides for example and define neighbourhoods of cells within those pathology slides to say well this tissue looks like this and it might behave like this because these neighbourhoods are next to each other. And you can do that at a gross level, you can do it in single level, and that’s giving us tremendous insight into cancer, yes, but also other disease types where cell cell interactions are really important. And that’s a small step away from being applied to routine, digitally applied slides from diagnosis and then being used in a patient.
Sean: There’s a data management issue here though isn’t there? I mean, how much data is there going to be with- Everything is- Or has it already been acquired anyway? Is this what we’re saying? You know Ganesh, so you mentioned you’re getting these scans anyway, happen to put them through the AI system or you don’t, you know, it’s become process I’m guessing, and then actually that’s just become another tool that you use?
Ganesh: Yeah I think it’s really important that the AI based solutions that we’re going to implement first are based on data that we would routinely acquire. Firstly, that would be making best use of our existing resources and we’ll see a good- You know, there’ll be a good cost benefit analysis for that, cost effectiveness for deploying these technologies. Let’s get the low hanging fruit first and then we can start talking about more sophisticated things.
Tim: And we’ve deliberately done that in our project. We’ve said that we don’t want to overburden the MDT with collecting even more data. What we need to do is have a system that works on the data that we know is readily available for every patient in every meeting and if that’s available, can we build a model on it and we can.
Sean: Excellent, so it sounds like it’s going well, the project. Any particular highlights of the project that, you know, because as we said it’s ongoing, what sorts of things have you found?
Ganesh: Yeah I think- So we did a national survey from multiple bodies, surgeons, gastroenterologists, oncologists, and there is a desire to have a machine learning tool for MDT. I think that clinicians on the whole are kind of getting a bit run down by MDTs. I don’t think I’ve seen anyone who’s been particularly enthusiastic when you mention the word MDT and I think we fouind that there’s a great appetite for it and it’s been incredibly easy to grow this team, and this team has grown. I mean, it started such as- As such as small team, small idea, and now we’ve got I think over 15, 20 people working on this project and I haven’t really had to ask them very much to- Or twist their arm very much.
Tim: We’ve done most of this work initially with internal data from Southampton. We have robust data. We know how the team works. We know what the data points are. That’s fine. But that means it’ll work brilliantly in Southampton. So one of the highlights for me has been we’ve also now got some data from Oxford from our collaborators and we’ve run the same system on the Oxford data and actually to our surprise and amazement, it translates amazingly well, in another centre’s data which gives us real confidence that we’re building something robust and we’re building something that might have utility in more than one place in the country. And then if you think about that it might have- You can spin that out further and further and further. That’s a recent highlight that we saw the data for yesterday actually that looks really, really solid.
Sean: Yes that’s superb, because of course lots of trusts work in different ways and have different teams and have, you know, different people invited to those teams I’m sure. What sorts of challenges have you seen with this project? Have you found challenges- You mentioned the data. I mean, collecting the data or the breadth of the data or people reluctant to engage? Have you had any of those sorts of challenges Tim?
Tim: Data. Data and the NHS is a- I know people work on this but data in the NHS is a mess. It’s still- Realistically, it still comes down to people collecting stuff on an Excel spreadsheet like it’s 1993. Genuinely, that’s what’s going on. We’re trying, and Southampton is one of the exemplars of digitalisation of the NHS, but still we can’t routinely drag information across as quickly as we’d like. We can’t collect it straight from the record. It’s cumbersome, it’s difficult, it relies on human beings inputting data and then the machine learning being applied to that. There’s an inherent problem there. It’s obvious. And we need to do better.
Sean: Is there a patient privacy issue there? Is that part of it? Is that why? Or is it just we’re not moving with the times fast enough?
Tim: It’s a massive resource issue. We all know the problems with the NHS at the moment and if you have a choice between putting people on the front line in the emergency department or paying for a data controller specifically to work in the MDT, I know where I’d put the money.
Sean: Where does this go from here? What comes next? I mean when does the project conclude? I mean obviously we said we’re recording this in April but I’m not sure exactly when the podcast is being listened to. Somebody might be listening to this years in the future, so give us a bit of an idea of the timeline on the current project.
Ganesh: So I think the project’s due to finish in July. It’s going really well but the problem is that just every step of the way there are new doors and we’re going down them and the team is growing. Things that I didn’t- Things that I would have to implement have now come to the forefront. For example, recently we’ve been collaborating with [unclear 00:19:38] Chapman’s Group looking at data drift because, you know, we were set out to make one model that works and we’ve done that. But now we’re thinking well, how are we going to get this into the NHS? What are the regulatory requirements that we need to fulfil? And it’s not really clear. So we’re going to have to adapt to the regulatory approval process. But also to the fact that, you know, it’s not just going to be a model that’s going to be deployed on day one and it’s not going to be the same on day 365, it’s going to be changing. We’re going to have to be able to adapt to new trials, new data. We’re going to have to be able to detect if something has changed in the system and whether we need to retrain our models so that leads to the data drift thing. So I think I don’t know when this project’s going to end. I think probably when I retire.
[00:20:27]
Sean: Fabulous, well I hope you’re able to have some closure on it before then. One thing I was thinking and you kind of mentioned there with the data management is, from my experience, and I’ve made one or two computer science videos, so that’s where my thinking’s coming from, you have things like missing data which you have to, you know, make decisions on what to do with the model in those cases. Is that also a big problem? I’m imaginging these Excel spreadsheets Tim just mentioned and lots of them having fields that are either not filled in or have slipped or somebody’s put it in the wrong place or- I mean, does tht cause big problems for a system like this or is that something that you’ve kind of built in to manage?
Ganesh: So in terms of missing data that is a very, very important point and it’s rife within health data I think, full stop. And so it makes me try to look for solutions that rely on the least number of data points. And this is an evolving process. We haven’t got there yet. Our current models are relying on multiple data points in the region of about 19, 29, but a lot of that prediction is made from very few data points. Maybe five or six. So I think that’s something in the future that we can concentrate on. How do we reduce the number of data points needed to make an accurate decision? At the moment, we’re using complete cases and we’re using data points that are routinely collected so, in a way, we don’t have too many missing fields, but I can see what you’re saying in terms of- I can imagine being in an MDTM, we don’t have one or two of the variables so what do we do?
Sean: Yeah, and I’m imagining- I mean, for those listeners, I’m guessing you’re in a healthcare scenario and somebody doesn’t take the temperature at 10 o’clock because the patient’s been taken somewhere else for some procedure or something, so that’s a simple example of a missing bit of data, right? Tim, is this something that you’ve had to deal with somewhat
Tim: Yeah throughout my career actually. Every time you write a paper and you’re trying to publish something around clinical data, missing data has to be dealt with. You can either ignore those cases and then you miss that case. It could be an informative case. Or you use a putation or some other way of making up the missing data points. But it’s an issue we need to deal with. I’ll stress what Ganesh said about this MDT process is that we’ve deliberately chosen points that are routinely available on every patient at every meeting. So it’s yes we do have a few missing, but not many, because we know the patient’s age. We know their tumour stage. We know this data. It is defined and it is recorded. So hopefully, in this particular model, it’s less of a problem.
Ganesh: Exactly that. I echo that. So if the data is missing there then probably we would have deferred that case anyway if we didn’t know the tumour stage for example. So I think in our particular project we’re okay, but you allude to the fact that on a wider healthcare AI problem, I think that is going to be an issue.
Sean: Not necessarily in the system we’re discussing specifically now, but you can imagine a point down the line where- Let’s blame the accountants, because most people do, somebody decides that the AI system doesn’t need any help with this and then you’re potentially into a computer says no situation. Now hopefully in very minor decision making, but you know, these are the sorts of things I’m kind of, you know, maybe I’m doom saying, I don’t know.
Ganesh: I don’t think the human will be out of the loop in these kind of scenarios. I just can’t see that happening. I might be wrong.
Sean: Yeah I suppose, I suppose thinking of the wider picture, the bigger picture of health and perhaps even just simple, you know, who gets to stay in the bed and who doesn’t in a ward for instance might come down to some system that decides okay, you’re okay now. But maybe not. I mean, as I say, I might be just doom and gloom. I don’t know. There’s something that we do come back to time and time again on the podcast and that is that AI has to be trained. If it’s trained on terrible information then how can it be expected to anything other than a terrible outcome. Now that’s often known as garbage in, garbage out. Is that something that we face here a little bit in health Tim?
Tim: Yes it’s a really important issue. I’ll use an example. I was asked to comment a few years ago on, I think it’s Google, I may be disparaging of them inappropriately, but one of the major companies made an AI model that they released to look at skin lesions and you could upload a picture of the skin. It was published in Age of Medicine. Upload a picture of the skin and it would tell you what your skin lesion was. And if you looked at the dataset that was built on, it was 90 something percent white skin. So if you were black or Asian, had black or Asian skin, then you weren’t represented in the data it was built on. So you might upload your image but you might not get an accurate result because the dataset wasn’t built on skin that represented you.
Sean: It just didn’t work.
Tim: And why would it work? Because it wasn’t trained on a skin type like yours. So- But that’s an inherent kind of engraining, persistent bias in systems that is inappropriate. Now if I bring it back to our particular disease, oesophageal cancer, adenocarcinoma, the commonest type in the UK, is a disease of middle aged, slightly overweight white men. But we occasionally see a lady with it. A female with it. We occasionally see a person who is black with it. So our model is based on 85% middle aged overweight white men. How accurate is it going to be for the female or the person with non-white skin? I don’t know the answer to that.
Sean: Yeah it’s a problem, and this comes round to sort of the idea of bias in kind of training and bias in data. In AI and bias is going to be a problem all over, but then you have bias in humans as well I suppose. I mean, you know, is there presumably a chance that you’re going to make that mistake as a human? Or is it just exacerbated?
Tim: I’m going to be really- We have to be really, really, powerfully clear here that we have an obligation and an opportunity and the time to get rid of that bias because we need to build models that are not biased and get over the perpetual human bias that exists. I’m really strong on this. We have to get this right. It’s perhaps a once in a lifetime, once in a generation opportunity to get rid of some of the horribleness that’s gone before.
Sean: If you want to get in touch with us here at the Living With AI podcast, you can visit the TAS hub website at tas.ac.uk, where you can also find out more about the Trust for the Autonomous Systems Hub. The Living With AI podcast is a production of the Trustworthy Autonomous Systems Hub, audio engineering was by Bordie Ltd and it was presented by me, Sean Riley.
[00:27:44]