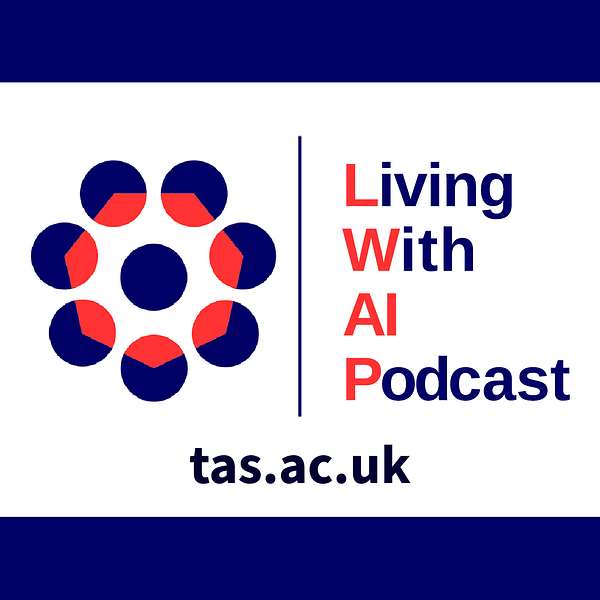
Living With AI Podcast: Challenges of Living with Artificial Intelligence
Living With AI Podcast: Challenges of Living with Artificial Intelligence
AI & Energy
Discussing what AI could bring to the energy grid. Can we trust artificial intelligence systems to improve the supply, either to improve reliability, efficiency or ecology?
We learn about the four "D"s of energy:
Democratisation
Digitisation
Decarbonised.
Decentralised
Podcast Guest: Professor Zhong Fan Net Zero Energy Systems , Exeter University.
Podcast production by boardie.com
Podcast Host: Sean Riley
Producer: Stacha Hicks
If you want to get in touch with us here at the Living with AI Podcast, you can visit the TAS Hub website at www.tas.ac.uk where you can also find out more about the Trustworthy Autonomous Systems Hub Living With AI Podcast.
Podcast Host: Sean Riley
The UKRI Trustworthy Autonomous Systems (TAS) Hub Website
Living With AI Podcast: Challenges of Living with Artificial Intelligence
This podcast digs into key issues that arise when building, operating, and using machines and apps that are powered by artificial intelligence. We look at industry, homes and cities. AI is increasingly being used to help optimise our lives, making software and machines faster, more precise, and generally easier to use. However, they also raise concerns when they fail, misuse our data, or are too complex for the users to understand their implications. Set up by the UKRI Trustworthy Autonomous Systems Hub this podcast brings in experts in the field from Industry & Academia to discuss Robots in Space, Driverless Cars, Autonomous Ships, Drones, Covid-19 Track & Trace and much more.
Season: 4, Episode: 10
AI & Energy
Discussing what AI could bring to the energy grid. Can we trust artificial intelligence systems to improve the supply, either to improve reliability, efficiency or ecology?
We learn about the four "D"s of energy:
Democratisation
Digitisation
Decarbonised.
Decentralised
Podcast Guest: Professor Zhong Fan Net Zero Energy Systems , Exeter University.
Podcast production by boardie.com
Podcast Host: Sean Riley
Producer: Stacha Hicks
If you want to get in touch with us here at the Living with AI Podcast, you can visit the TAS Hub website at www.tas.ac.uk where you can also find out more about the Trustworthy Autonomous Systems Hub Living With AI Podcast.
Episode Transcript:
Sean: Welcome to Living with AI, the Trustworthy Autonomous System Hub’s own podcast. The topic for this episode is energy. I’m Sean Riley your host and we’re recording this on the 22nd of April 2024 and our guest for today is Zhong Fan.
Welcome to the podcast, Zhong, can you just give us a sense of who you are, what you do and just introduce yourself?
Zhong: Hello, my name is Zhong. I’m a professor of Net Zero Energy Systems at the University of Exeter. So, I’m leading a group of researchers working on mainly Net Zero Energy Systems but my own focus is mainly on the application of AI and digital technologies to this area. Thank you for inviting me.
Sean: You’re absolutely welcome it’s really great to have you here. Where do we start with this then, how does AI intersect? You know, what’s the relationship there with energy. I mean is it managing it or is there more to it than that?
Zhong: Well probably I can come about it from my own sort of journey and how I got in to this. I’m from an ICT background, I was trained as a communications engineer and then about maybe 10/12 years ago I moved in to this area applying ICT technology to smart grid and to energy in general. And, obviously, over the past few years we’ve witnessed this tremendous advance and success of AI, to start with, maybe, you know, computer vision, image processing and languages, you know, speeches.
But now because it’s so successful people have applied it to different sectors or as we say verticals, you know, different industries. There are also a lot of applications to the energy area that we’ve seen, although I would say so far the majority of it are kind of still in the academic domain. There is limited success in the actual practical applicated industry and adoption of this industry.
Sean: Is that because the energy industry is quite conservative. It’s kind of- I don’t want to say scared of moving forward but, you know, it’s a cautious industry?
Zhong: Yeah, in a way it is. And also, I think there are two things. One thing is that obviously, it’s a critical infrastructure, you know, you want to keep the lights on, you know, 100% or 99.9% of the time, so, you need to be very reliable. And on the other hand, the infrastructure has served us well for the past, maybe, you know, 100 years most of the time. So, I think that actually is one of the reasons that when you apply a new technology such as AI, we need to make sure it is, you know, trustworthy or explainable, you know which have these desirable features.
Sean: And I know that in the UK we have the National Grid which is much celebrated by people who live here, I don’t know, if it’s as good as we all think it is, but it’s a very reliable system. How can you improve that with AI or is that the idea, to think about moving it or is this more about the environmental impacts than the reliability side?
Zhong: Well, for example, traditionally- I think it’s a long story, let me put it this way. So now in the transition from a traditional electricity grid to a more kind of Net Zero or sustainable grid of the future, we are often talking about four “D”s, which means democratisation which means, you know, you want to involve citizens, right. So not just consumer users but rather some of these consumers will want to become more involved or even producers, so, there’s a terminology called prosumers.
So democratisation that’s the first D. The second D is digitisation, so we know, you know, you want to apply ICT technologies including data, AI to enable the digitisation. So that is one of the reasons within AI. And third is decarbonisation, obviously that is quite obvious. And the fourth is decentralised. So decentralised meaning in the past we have, most of the time, we have a one way system. You have the generation, you know, transmission, distribution, all the way down to everybody’s homes and offices, so it’s one way. And now because we have so much, you know, renewables and distributed generations so that adds complexity. So, these distributed type of systems.
And also that means we will have maybe thousands of millions sort of powered electronics devices connected to the network. And that, again, adds uncertainty and complexity and we think AI may be able to help for us to make some predictions to enable sort of informed decision making.
Sean: Fantastic. I lost you for a moment there, but I think I got the gist of it. I think our internet just gave us a little blip. But, obviously not decentralised enough our internet- Just thinking about what you’re saying there and that idea of decentralised, it’s going to take AI or something similar to manage all of that isn’t it. I mean we’re already getting- Well certainly I know in our house even we have a smart meter, we have an electric car charger which is smart. And all these different devices they, you know, work to do simple things like send the meter readings back to the utility company. But they also do things like make sure that the car charges at a time that’s good for the whole grid. I mean is that what we’re looking at, just more devices being cleverer? I know that’s maybe an over-simplification.
Zhong: Yeah, in a way. I mean from the homes perspective you have more and more devices and also from the grid side, you know, you want to connect all these sort of generations, like renewables, then you need some clever sort of electronics devices to enable that. You know, DC/AC, conversions that sort of thing and all these add complexity.
And on the other hand we talk about not just electricity but also other [s/l convectors 00:06:29] like gas networks, heating. You know, heating is one of the big problems for decarbonisation in this country. The Government’s pushed by the plan of, you know, everybody has got a heat [s/l Plan B 00:06:41] instead of boilers, you know, things like that.
And also hydrogen, for example, that’s another sort of hot topic at the moment. You know, and you’re at your destination, they’re EV. So, all these things, you know, sort of are exciting but in the meantime add complexity to our system.
Sean: And you touched on something there which I think is important which is that I started talking initially purely about electricity but, of course, there are all these different things that we use, like you said, the gas, some people use oil to heat their homes. There’s a lot to be said for this kind of energy. And do you think AI is going to be able to help kind of bring all that together?
Zhong: I think probably AI- Personally I think it’s an enabling technology. So, obviously, you know it can help in some ways but not everything. You know, we need some kind of- Again, we need, for example, chemical engineering, we need some physics, you know, material sciences, all these advancements. But, from the digital space, AI could help for us to make some, maybe quicker decision makings or some, you know, more accurate predictions.
As an example, so a lot of these energy companies, they have a lot of assets they have networks, cables and so on, you know. And in the past if something goes wrong more often you need to send people over to have a look. And now if we’ve got more sensors or even if we can fly drones to these places and then bring back, you know, some of these images and then we use clever AI to predict, you know, maybe there’s likely some fault in the future, you know, we can sort of proactively take some actions. So, that is beginning to happen in the industry.
Sean: So, basically, yeah, I mean it’s easy to think, oh, AI it will be running in real time, fixing stuff and moving around the distribution but actually it could be used in planning. It could be used in, you know, fault detection as you’ve just said. There’s all sorts of different potential applications, aren’t there?
Zhong: Yeah, that’s right, yes.
Sean: How do we sort of think of responsible AI, particularly, in something so complicated as the energy network. How do we think of responsible AI in relation to that?
Zhong: I think probably- Because this is about, you know, trustworthy AI and I’m sure, you know, you have a lot of experts talking about, you know, the deep learning, you know, stuff like that. And there’s some advancement of explaining, you know, why deep learning works and so on. Largely, it’s a black box, let’s say, start with the black box because it’s so complicated, millions of parameters.
[00:09:42]
So, if, let’s say you are a grid operator, you know, and sort of responsible for infrastructure like the UK grid and then you want to deploy this sort of a black box in your system and, in the meantime, you have to make sure, oh, if there’s an outage, you know, can the black box tell us what’s going on. Or, the black box says this, can I trust it, so that’s a question. So, that’s why I think at the beginning I said, you know, there’s some applications of AI in this area but there’s still limited, you know, adoption. So, that’s why, you know, we need to actually, from a university’s perspective, we need to put in more research in to this area. How can we make AI- Basically how can we convince a power network operator this is something you can trust.
Sean: Well, I suppose- Could it make the argument that you don’t know that a team of humans is always going to be able to give you the right answer either in certain circumstances. So, yeah, and it needs to be maybe run in parallel for a little while with a conventional method and check that way perhaps.
Are there any examples of where things are working well? Has there been any adoption already and can you give us any examples of that?
Zhong: One of the things I think currently, at least I know has gained some popularity, is combining AI or data driven models with physics models. For a power energy network, we got, you know, years on years of theoretical work and models, we know it works well, you know, based on physical laws. And now we’ve got a huge amount of data.
So, one of the interesting, I think, research reference is to combine these- How can you combine these physical models in to your integrate, in to your, you know, machine learning or AI models to make a more trustworthy or more safe [unclear 00:11:59], because you don’t want the AI out with certain sort of actions which is not safe or beyond the constraints of the physical system. So, that’s something, you know, currently there is a lot of interest of doing that.
Sean: So when you say a physical system there do you mean, actually, you know, trying to get it to almost take over the reins of something that at the moment is managed by humans but then maybe we’re thinking AI could manage it instead, is that what you’re saying?
Zhong: Well, it’s kind of- Combine some of the- We’ve got a traditional, theoretical or physics models, obviously that involves human knowledge, you know, that’s a lot of expert knowledge. And with the pure data driven, got a lot of data, more often we use AI to manipulate this data, to interpret this data. So, it’s kind of combine the strengths of both worlds.
I think one of the interesting applications would be- One of the buzz words is digital training, right. You want to be able to digital train. And I think that’s a very powerful tool to build like physics informed machine learning and then use that to build digital training. It is hard, digital training, although it is very popular now, everybody’s talking about it, but I think for a complex system like an energy system, it’s very hard. You may be able to build kind of a sub-component, the digital training of sub-component, maybe, you know, a transformer or even part of a transformer but when you talk about sort of a real network, a distributing network, for example, that’s still quite challenging.
Sean: You may not have these numbers too hand, but how big is an energy network like the UK’s? You know, how many transformers? I mean are we talking hundreds, thousands, tens of thousands?
Zhong: Typically if you look at National Grid, we’ve got the [s/l popology 00:14:01], you know, the graphs, yes.
Sean: So, I suppose when thinking of that, because, you know, the digital training perhaps just has to be simplified for that sort of situation, it’s just too complicated, isn’t it?
Zhong: My understanding is that- First of all people have different definitions of digital training, so there’s no sort of universal definition. But I think it depends on the level of instruction. You may have a system level to do training but obviously down to the devices, it’s just [unclear 00:14:38], right.
Sean: Yeah, yeah.
Zhong: So, that depends on the granularity.
Sean: Yes. You try and make a digital twin of the National Grid you’re probably not looking at the stresses on one washer in one transformer-
Zhong: Of course, yeah.
Sean: Well, look, l mean in some of the very kind of critical areas like health and things like this, people are looking at using the AI as a tool that, you know, still has a human in the loop, do you think that’s something that energy needs as well or are there certain areas that can just be automated and we can trust it and that’s fine?
Zhong: I think energy is typically- Because people are actually using it, I think we definitely need a human in the loop. One of the things I think AI may help but I don’t know how yet, is how can AI be used to help people, for example, to accept new technologies to change their behaviour. So, a great example is smart meters, for example, I think when 10 or 15 years ago the smart meter, you know, the government tried to roll out the smart meters, you know. The hope was, okay, with these smart meters and this display in our homes, people will change their behaviour, will be more, you know, conscious about their energy usage and, you know, basically will save energy and save money. But a lot of studies, including our own, have shown that is not the case. You know, maybe on the first few weeks, you know, you tend to look at the display and then say, oh, how much are you. And then after maybe you just put it in the drawer.
Sean: Yeah, yeah, that’s definitely my experience. And having said that, there are a couple of things I would point out, one is I don’t think there was any incentive to, you know, alter your behaviour. Yes, you could see how much it was costing but there wasn’t any incentive, certainly in the tariffs that we’re on here, because I also think that smart meters, and this is just my own personal opinion, were a bit of a- Not the smart meters weren’t a mistake but the way they were rolled out was a mistake because they weren’t compatible with changing suppliers now, I think this has been remedied.
But certainly in my experience, we got a smart meter then slightly further down the track decided to change supplier and then the smart meter didn’t work anymore. And we were told you need a different smart meter. And so I think, unfortunately, this kind of implementation has caused people to almost step back a few paces and go I don’t want this.
Zhong: Yeah, so in that respect I was wondering- Because if we got a lot of consumption data and whether AI can help us to design a better scheme- How to better engage with customers. And how to design incentives to enable customers to make informed decisions about, you know, for example, different tariffs or different energy companies.
The other interesting thing is, in my previous university we had this trial of hydrogen injection in to the gas pipe with 20%, so, one of the first in the country. And obviously at the beginning, you know, some people are worried about the safety of hydrogen. And then I think my colleagues did some interesting studies actually in the social sciences domain to see how people’s perception, you know, changes or no change during this trial. So, a lot of things like that, I think AI may be able to help. So, we need to work with [unclear 00:18:21]. I think we need to work with social scientists for example.
Sean: Yeah, it’s an implementation that needs to be done correctly, as you say, social engineering needs to come in to play.
I mean I was thinking about the smart meters as well and the smart charge and things, how smart are they? Is this purely a system that is able to communicate or is there any AI going on there?
Zhong: Okay, definitely it has connectivity, yeah, so that’s one thing. How smart, I think that in theory it can be smart but probably- I didn’t look at it in detail, but my impression is probably it’s very limited at the moment. In theory, for example, the smart meter can be working together or even connected to your home, sort of a gateway, you know, Alexa, stuff like that.
Sean: So, I guess, potentially smart as part of a larger system but-
Zhong: Yeah, it could be in, let’s say, your home, smart home, sort of a management system, one of the components.
Sean: Yes. Okay, yeah, yeah, it makes sense, it makes sense. So, we talked a little bit there about potential negative connotations of AI and I’m just wondering how do we kind of get past that, do we think it’s down to social engineering as much as anything?
[00:19:40]
Zhong: Well, obviously, there’s an ethical question, you know, even, I think the philosophical question- I’m not an expert but one of the things- I can give you an example, maybe, how this works. So, again, when the smart meter came out, not just in the UK but mainly in the mainland Europe, there were people concerned about their privacy, right. So with the smart meter data third parties can get to know your, you know, consumption, your behaviour. When do you sleep, when you wake up, you know, the pattern, living pattern. So, then I think this quite interesting problem, at least for the academic, but also for the utility companies, especially from a GDPR, you know, privacy concern.
So, I think currently we have a different sort of a method to so called privacy preserving data analytics. And that is actually applied equally to AI and machine learning. So, how can you sort of protect people’s privacy while in the meantime do something interesting.
Sean: So, that’s an open question I suppose.
Zhong: Yeah, in this area in terms of how do you do machine learning, to suit identity, you know, rather than doing it in the Cloud, so that you have to send your data there.
Sean: And plus, of course, to train systems you need data to train them. So, you need to have the data to be able to train them to work properly.
What do you think we’re going to see going forwards a few years, what’s in the pipeline?
Zhong: Again, I think, especially- I think people realised, okay, AI is very powerful and if used properly it can do good things for the humanity and the society. And, in the meantime, how can we make it- If we deployed it in the mission critical system, like energy, like infrastructure or even health and medicine, how can we make it more sort of trustworthy and, you know, explainable, you know, reliable, all these sort of good words. How can we make it happen. Obviously, that’s a lot of- In the computer science area, there is a lot of work in this direction. And, as an engineer, as we apply advancement of AI to different sectors, I think that’s something we keep an eye on.
Sean: If I just ask one last question, how have things like the TAS Hub helped with your research and, you know, finding out more, and what’s going on there?
Zhong: Well, I think the Hub would be a good opportunity to bring people from different disciplines together, because essentially AI starts with, you know, obviously, computer science, you know, researchers. But now since that’s been applied to all sorts of areas and sectors, I think it would be good if we can bring in, you know, like a Hub, bring in sort of people from all sorts of different disciplines, engineering, computer science, you know, social sciences, business people, law, all these together. Because, in fact, the challenging problem like energy does involve all these people.
Sean: Yeah, absolutely, it definitely does. And, yeah, it’s great to hear that, you know, things like TAS help with that mixing those different disciplines together because academia doesn’t do it often enough in my humble opinion.
It’s been absolutely fantastic to have you on the podcast, thank you very much for sparing your time.
Zhong: Thank you very much.
Sean: If you want to get in touch with us here at the Living with AI Podcast, you can visit the TAS Hub website at tas.ac.uk where you can also find out more about the Trustworthy Autonomous Systems Hub. Living with AI Podcast is a production of the Trustworthy Autonomous Systems Hub, audio engineering was by Boardie Limited and it was presented by me, Sean Riley.