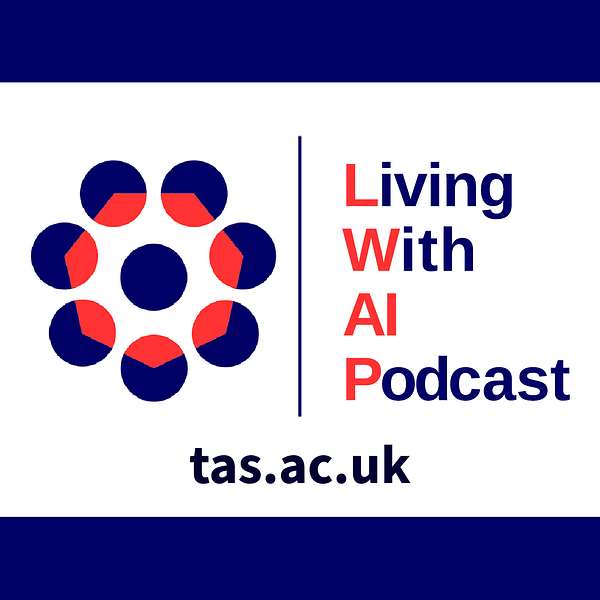
Living With AI Podcast: Challenges of Living with Artificial Intelligence
Living With AI Podcast: Challenges of Living with Artificial Intelligence
What is the Trustworthy Autonomous Systems Hub (TAS Hub) anyway?
Sean, Paurav, Christine & Joel debate Social Media Filter Bubbles before Gopal Ramchurn explains what the Trustworthy Autonomous Systems Hub is.
Paurav Shukla
Joel Fischer
Christine Evers
Sean Riley
Gopal Ramchurn
Podcast Host: Sean Riley
The UKRI Trustworthy Autonomous Systems (TAS) Hub Website
Living With AI Podcast: Challenges of Living with Artificial Intelligence
This podcast digs into key issues that arise when building, operating, and using machines and apps that are powered by artificial intelligence. We look at industry, homes and cities. AI is increasingly being used to help optimise our lives, making software and machines faster, more precise, and generally easier to use. However, they also raise concerns when they fail, misuse our data, or are too complex for the users to understand their implications. Set up by the UKRI Trustworthy Autonomous Systems Hub this podcast brings in experts in the field from Industry & Academia to discuss Robots in Space, Driverless Cars, Autonomous Ships, Drones, Covid-19 Track & Trace and much more.
Season: 1, Episode: 4
What is the Trustworthy Autonomous Systems Hub (TAS Hub) anyway?
Sean, Paurav, Christine & Joel debate Social Media Filter Bubbles before Gopal Ramchurn explains what the Trustworthy Autonomous Systems Hub is.
Paurav Shukla
Joel Fischer
Christine Evers
Gopal Ramchurn
Podcast Host: Sean Riley
Producer: Louise Male
Podcast production by boardie.com
If you want to get in touch with us here at the Living with AI Podcast, you can visit the TAS Hub website at www.tas.ac.uk where you can also find out more about the Trustworthy Autonomous Systems Hub Living With AI Podcast.
Episode Transcript:
Sean: Welcome to Living With AI, a podcast where we get together to look at how artificial intelligence is changing all of our lives, how it alters society, how it's changing personal freedom and what impact does it have on our general well-being. Today we're going to feature the TAS Hub, which is actually why the Living With AI podcast exists, so thank you TAS Hub. This is the newly set up UKRI Trustworthy Autonomous Systems Hub, the UK's flagship research programme on trustworthy artificial intelligence, easy for some to say. We'll hear from Gopal Ramchurn, who is the director of the TAS Hub. Have I said TAS Hub enough times yet?
Before that though, time to introduce our panel. Today, joining me via the magic of cyberspace are Paurav Shukla, Joel Fischer and Christine Evers. Paurav is Professor of Marketing and Head of Digital and Data Driven Marketing at Southampton Business School. He's recently become an avid runner and cyclist, but that may be on pause because looking at the shot I can see on your webcam you appear to have a broken arm.
Paurav: That’s true. It's, you know, Sean, avid cyclist probably became a little too engaged with it and possibly did not see the gutter, which was below the leaves on the road. And so my X-ray vision didn't work.
Sean: We can't blame AI for this then.
Paurav: We could, in a way I could say that, hey, why did my cycle did not have that X-ray vision that told me don't go there.
Sean: Absolutely. Joel is Associate Professor in Human Computer Interaction at the University of Nottingham. He likes rock climbing and also running, but I think you're wisely avoiding cycling by the looks of it, Joel?
Joel: Hi, Sean. Yeah, I've just been out for a run. So I seem to have developed a little cold. So apologies for that.
Sean: No problem. Christine is a computer science lecturer at the University of Southampton. She focuses on machine listening and also a road cyclist. I was going to ask if Paurav was catching you up on Strava yet, Christine, but I'm afraid we'll have to have that conversation another time, won't we?
Christine: Yeah, I think it would be unfair to check his Strava account right now.
Sean: And I'm Sean Riley. Normally I'm hiding behind the viewfinder with a wobbly, out of focus camera pointing at a clever computer scientist. But today I'm once again hiding, but behind a microphone this time and at least it doesn't have to be in focus. We're recording this on the 12th of November, 2020. So if you're from the future, what's your hover car like? Has it got some good stuff? Anyway, what's been happening this week? I mean, I researched AI today and the one thing I found was that a Cape Town AI summit was cancelled. I wish I was in Cape Town at the moment, but it's been cancelled due to Covid. I expect lots of things are cancelled due to Covid.
But we're not going to talk about that today. There've been a couple more interesting things happening in the world of AI, one of which is a new car from Honda. But also we've just had the presidential election and there's a lot of things floating around. Joel, what have you gleaned from what's going around on the internet at the moment?
Joel: Yeah, I've just read an article talking about some of the groups on the usual social media platforms that have been banned. It's interesting that we're still very much, I seem to find we’re still very much in this predicament where misinformation is driving a lot of the interactions on social media. And unfortunately, a lot of that goes back to the good intentions of the makers of social media platforms to bring the right content at the right time. I think the flip side of it, we've all seen now with a vast amount of people believing that there's been a lot of fraud to do with the American elections.
Sean: There's this constant problem with any social media where they are trying to refine it to give you what you want to see and that just reinforces this problem with the echo chamber. I know it's known as the social media bubble as well?
Joel: Absolutely.
Sean: Have other people had experience of this social media bubble?
Paurav: I would certainly say so. We all live in, in some sense, social media by itself creates that bubble around us. And earlier, we were talking about Joel and Christine and I, and we were talking about this in terms of some news that Joel sees, which we don't and what we see he doesn't and so on and so forth. So those bubbles affect us and our decision making in every which way.
Sean: Do you think there's a responsibility for those social media platforms to try and show us more? Or is the onus on us to understand that's happening? And to, this is an unfair question for anybody, but I just wonder if they can do more to show us both sides of a kind of debate? Christine, what's your experience with these social media bubbles?
Christine: It's the same as the other two. I think it's by nature, by us being able to create a circle or a network of people we would like to listen to, to also reinforce that isolation in a way. But I do agree with your statement, Sean, that is there perhaps a mechanism that social media services could provide where you as a user could even select how much diversity or how much diversity in news you would like to receive on your feed? And it doesn't have to be that they enforce that, but at least if you had the option, then people who would like to be informed about other topics could have the choice to do so.
Sean: And is this AI driven? We talk about algorithms and algorithms kind of deciding what's in our feeds. I mean, if you've got, I don't know, pick a number, 300 friends on Facebook, then you can't look at 300 statuses at one time. So there has to be some selection as to what you do see. Is that AI doing that though?
Christine: It certainly could be. If you think about Spotify, if you're a Spotify user, music recommendation is hugely AI driven in the sense that the system learns what your preferences are and then basically hones in on that and tries to play music that the system believes will fit within your taste. But then there's also a diversifying part to that, in that it would like to expose you to similar music, but that is still within the same genre, but also pieces that you haven't listened to in order to actually retain user engagement and avoid boredom. So this certainly could be extended to news or social media items.
Sean: But it did used to just be chronological, didn't it in these feeds? Joel, what do you think about that?
Joel: Yeah, I was going to say exactly that, Sean. It used to be simply that you would see things in chronological order in which they were posted and that was fine, I think. But then, you know, I think nowadays, the algorithms very much take into account the things that you might like or that you have liked, that you've given a thumbs up to, the general kind of popularity of certain vote, of certain stories and how many comments they have received. What you might have commented on in the past, what you have declared as your interest in terms of your hobbies or cultural interests. And these things all get taken into consideration in terms of what's being presented in your newsfeed and it's no longer the timeline, is it? It's called a newsfeed now for a reason. And I think that's, I mean, I think that's problematic.
Sean: Yeah, so basically, by trying to improve things for us, maybe for a lot of people, they've actually broken it for us. There's definitely an agenda going on here, though, isn't there? But I know, say, for instance, Twitter has the option to choose chronological or what they call home, which is curated, can I say, for want of a better word?
Paurav: I would agree with both what Christine and Joel say. But we have to understand that when employed at such a large scale of social media, what other options do we have? Because if we go into that joy of randomisation, we still remember that when Apple iPod was launched and they had that random shuffle button, and actually users complained that the same song was being played three times at a time. But that is what random is. So random is really confusing to human minds. So we will have to employ some smart mechanisms to actually make sure that people receive what they may have liked.
But in doing so, we are also creating a Machiavellian approach to news, because it would continue to decide what we would like more because it wants us to like more and engage. The point for many of the social media is the longer we stay with them, the longer they can monetise. And so the way they can keep us there longer, if they can produce some sort of interesting or likeable material. And that is where this buck stops. So it is a big debate between profitability versus what is good.
Sean: There's an interesting documentary called The Social Dilemma you can view on Netflix at the minute. And they liken it to being looking at your phone as being like pulling the handle on a fruit machine, you know, hey, what am I going to get? Am I going to win? Is there going to be something cool there? And then that the social networks want to basically have your eyeballs, I think for what's the better word. Have you seen this, Joel?
[00:09:53]
Joel: Yeah, I've seen it and I recommend it to my students. It's, yeah, it's, I mean, it is a dramatization to an extent as well. But it's also very informative. And it does show, it lays bare the business model of a lot of the internet companies, which, you know, it's not free. The currency that we are paying in is our attention and it's been talked about as the attention economy. And I think it's, you can't underestimate the implications of that, I think. A lot of that, a lot of what we were talking about to do around the kind of filter bubbles are a direct product of the business models of the internet.
Sean: Yeah, if you're not paying for something, then you've got to be wary that you're the product, right, Paurav?
Paurav: Absolutely. There is no free lunch in this digital world either. And funnily enough, if you saw, as we are talking about today being 12th of November, yesterday only, Google announced a very interesting thing about Google Photos. So many Android users have been using that high quality Google Photos saying, you know, you have it free unlimited photo upload on Google Photos. But now, it's no more from next June or so. And from next June, June 21, I think 21st or June 2021 is when whatever you upload will be counted towards your 15 gigabytes worth of data. And so that means, and Google has already created a kind of algorithm which tells you how quickly you're going to get that over with.
So I was today morning looking at it and it said two years and I don't think it'll take me two years to actually fulfil that 15 gigabytes. But what it is also interesting is that in some sense by providing that service free, Google taught us what free databases could look like. But in some sense, it was actually using that photo database to make sure that machine learning algorithm has learned. And now they are saying that something like 28 trillion data bytes worth of something is available. It is unbelievable, those numbers.
Sean: Well, I think this is a thing, you know, these smartphones have allowed us to take more and more photos and why would you ever delete anything when somebody is offering you free storage? It's only going to go up. We get addicted to the idea of keeping everything. And then, yes, as you say, they train algorithms and detection, computer vision algorithms on this. But also they can then very quickly potentially monetise this because once you've, once you've got somebody addicted to something, then you turn the taps, right? They do have a habit of starting something up which people buy into and then deciding to close the programme. What's a good example? Picasa was one. Google, what was their social network?
Paurav: Wave.
Sean: So there's so many options. I've forgotten half of them.
Christine: Yeah, but often the problem for Google specifically, I think there's often also the situation that users simply don't use it. If you think back to what was it called? Google Plus, I think it was, which was supposed to be their social media platform, it just simply didn't receive the interest that it wanted. And the option of basically providing a free service where users get hooked and then you make it a paid for service. That's sheer marketing. I mean, if you think back to Dropbox, who originally provided vast amounts of cloud storage, which was absolutely brilliant. And suddenly they said, ah ha, so now you pay for a pro account, which was not an insignificant sum. But since your data is on the cloud and it's so convenient not to have to remove it, people paid for it and stayed with the programme. So it's actually quite a clever marketing system if you think about it.
Sean: I think it's what drug dealers do, isn't it? Turning to something completely different and self-driving cars are kind of coming back into the fray. You spotted something, Paurav, an article about a new Honda car. Can you tell us about that?
Paurav: Yeah, Sean, it's very interesting. In a way, Honda is the first automaker who is now claiming that it will be able to mass produce autonomous capability vehicles, which meet a standard that was created by SAE International Systems and they are now saying it's a Level 3 standard car that they are thinking they would be able to launch by March 2021. And that is quite an achievement because Level 3 is the first categorisation wherein most experts would call it actually autonomous, wherein the driver can fully allow their vehicle to take over control. And only in certain conditions, the vehicle would ask the driver to take back control. Neither, it'll just continue to ride. And so that is some achievement in itself, if it actually becomes reality.
Sean: Where will these sorts of cars be able to be used, though? Because the big problem here we've got is basically the legislation that surrounds this kind of an autonomous system. Christine, what do you think about that?
Christine: So for me, the most worrying part is about mixing systems. So I believe if you dedicated a motorway, say, to lorries that are autonomously driven, and every vehicle on that motorway is steered and driven by, or controlled by the actual machine, I think the system could work. What worries me is the unpredictable nature of human drivers. Being a driver myself, I see things on the road that are just absolutely flabbergasting. And there's no way that a machine can basically predict the sometimes very erratic behaviours that humans may exhibit on the road. So for me, the problem really is that mixing of a human system with an AI system.
And equally, a human is not aware that a car may be driven by an AI, unless they actually pass that car and see whether or not there's a person in the seat. It might be that there's a driver in the seat, but the car is still controlling the vehicle. So I think the explainability between a human-controlled system and an AI-controlled system, that is a part that is, for me, a very worrying or a very, very big concern.
Sean: I've read something on Twitter, probably a year or so ago, where somebody put that the reason that they don't trust an autonomous vehicle is an unexplained item in the bagging area. You know, the fact that we still can't even get autonomous tills to work completely reliably in a supermarket, means that how can you make a vehicle work autonomously in the myriad of possible situations, as you've just said, erratic drivers, etc.?
Joel: Well, I entirely agree with Christine. I think it's really important to think through the implications when these vehicles are in the actual world, where there is lots of other vehicles driven by human beings who might not be paying attention. So I'm driven by different kinds of autonomous systems to an extent as well. I think, I mean, often when we talk about autonomous systems, and the press and the media doesn't really help there, it's sort of muddling the terms and there's very much very different kinds of- I mean, quite often the most advanced autonomous systems we have now are sort of autopilot type autonomous systems, autonomous driving autopilots.
So they're not fully autonomous, and they only work under certain conditions. And some of those have also been shown to, it's been shown to be important for these features to have the ability for humans to not, to not sort of sit back and completely take the hands of the steering wheel. But instead, what Tesla did with their autopilot is it will only work if you put your hands on the steering wheel now.
So bringing some of that human control back into the loop and I think this goes to the heart of what we're trying to do in the Trustworthy Autonomous Systems Hub. So I think that, is to get this balance right between autonomous systems and human beings.
Sean: Our featured topic this week is the Trustworthy Autonomous Systems Hub or TAS Hub. If you haven't made it to the end of our podcast yet, for full disclosure, this podcast was set up by the TAS Hub and the person we have to thank is Gopal Ramchand. Hello, Gopal.
Gopal: Hi, Sean, how are you?
Sean: All right, thank you. Gopal is Professor of Artificial Intelligence at the University of Southampton. He has won multiple Best Paper awards at AI conferences and works closely with industry. His specialisms include game theory, data science and machine learning or ML. If we're going to go to abbreviations, which we may need to do just to fit all his accomplishments in, there's so much to talk about. Instead of me telling you, let's hear it from Gopal himself. Welcome, Gopal.
Gopal: Thanks, Sean. Thanks for having me on the podcast.
Sean: No, no problem. What AI work are you most proud of that you've been involved in?
Gopal: That's a good question. I mean, the one thing that I talk about that catches most people's attention tends to be my work on fantasy football. So we wrote up this paper about eight years ago on an AI bot that would play fantasy football and beat 99.9% of all human players at fantasy football. So that's my most famous piece of work, despite all the other work I've done on AI, multi-agent systems, machine learning, etc. Yeah, that's got lots more traction and people know me for that in some circles.
[00:20:09]
But no, in the research community, my work's been mainly about multi-agent systems and coordinating robots, coordinating flying, flying robots, etc.
Sean: So not to get down the sidetrack with this, but we're talking about trustworthy things and the first thing you did was cheating at fantasy football, right?
Gopal: Yeah, that was a big, big, big issue we had to address. I mean, people said, why would we ever play the game if AI is able to manage all this data faster than any human and therefore, there's no point in playing the game anymore, if machines are going to win. And we said, no, it's not all about just the AI winning. It's all about humans also understanding the subjective nature of the game, the uncertainty in the game that machines can't really capture. So we had some arguments against that.
Sean: I mean, talking about cheating, I've been cheating and reading your website. So I know that you're interested in the development of autonomous agents and multi-agent systems. Agents are a word that gets thrown around a lot in academia. What does it mean in the case of autonomous systems?
Gopal: Okay, so agents, when we talk about agents, we talk about software agents, so algorithms, programmes that run in apps or run on a robot or run in a car or on the web, that have some intelligence built in so they can react to things that they sense in the environment, they can communicate with each other and share data and information with humans and other software agents. But agents can also mean robots, right? So any intelligent machine that is able to do things on its own, act autonomously. Autonomous systems is a term that we typically use to describe machines that are autonomous, have some intelligence to act on their own. And that means typically robots, but autonomous systems is a really broad term and can encompass humans as well.
So you have systems of humans and machines working together in an autonomous system. For example, a plane might be an autonomous system with some human input. A robot, a ground robot, an autonomous vehicle on our roads is an autonomous system, but does have some humans in the loop to help adjust its settings and guide it to its destination. But typically, it's the machine that has some intelligence built in.
Sean: What is it that you're working on at the moment then?
Gopal: So right now we're looking at how we make large teams of autonomous systems work alongside large teams of humans. How do we make them trustworthy? How do we ensure that we can predict their behaviours? How do we monitor their state when there's so many of them maybe flying in the air or on our roads producing vast amounts of data? That's really difficult for one human or one system to capture and make sense of. So my work is really looking at how do we build systems to simplify or extract the key insights that humans may be able to cope with without being overloaded?
How do we make sure that human teams work with that data and work with these autonomous systems in a seamless way? So how do they coordinate? How do they collaborate to make sure that they all work well together? And you can find good examples of that in the real world as new applications are emerging. For example, looking at large teams of drones that may be going out delivering parcels or surveying an area, or looking at emergency response where you're deploying drones and robots on the ground to find or extract casualties from under rubble in collaboration with humans, other human emergency responders that need their help or need to supplement with extra information or move things around for these robots to do well.
Sean: When we're talking about the trustworthiness of these autonomous systems, well, when you talk about autonomous systems, people jump to all sorts of ideas like drones, autopilot, self-driving cars like you mentioned, but we all have automation in our houses right now. We have washing machines, it's come up on the podcast before. We have central heating timers, even Spotify saves you scanning the CD rack for what to listen to. What's so different about some of these newer systems?
Gopal: So exactly. So the point is we're already surrounded by lots of AI, lots of autonomous systems. We have Netflix, for example, suggesting movies for us to watch. We have the Nest thermostat adjusting the temperature in our homes. We have Google Maps navigation systems guiding us on a daily basis. The key issues start arising when they really impact on our well-being, on our individual mental and physical well-being, when they start impacting on our financial, on our finances, start impacting on the efficiency of work, for example, and really cause us real trouble.
A good example that people may be able to relate to is the Nest thermostat. So there was an interesting study done on the Nest thermostat a while back. So the Nest thermostat is one of these intelligent thermostats that try to learn what is the temperature really what your home at, right? So should it be 22 degrees? Should it be 25 degrees? Should it be 19 degrees? And we know that men and women, for example, do need different sorts of, do find different temperatures comfortable, right? So in a family, you'd expect different members of the family to have different preferences.
So if you have one thermostat deciding that for everyone, you can expect that some people will be unhappy and therefore they turn off this automated, so autonomous feature. That's what the study revealed, that people were not happy with the intelligent feature. So, as soon as it starts impacting on your comfort, your well-being, people get a bit nervous about that. If your satellite navigation system, for example, doesn't react fast enough when you decide to change another route or you missed a turn, you may not trust it next time you go out. So you might want to try something else or just rely on the science that you see.
So we are starting to now being pervaded by many more of these sorts of autonomous machines in various contexts, they are more present at work. They guide us as to what should the next task be at the office, on our email systems. They filter our emails on our behalf without us deciding, sort of having a say into that. They may be organising the delivery of goods and services. For example, Ocado uses lots of robots to optimise its warehouses.
And we worry about what will happen if things go wrong, if one of these machines fails. When you have lots of them working together, connected with each other, what will happen? It's very hard to verify and trust these systems. So yeah, lots of worries arising as more of these machines become dominant in many, many environments.
Sean: Sometimes it comes down to mere implementation as well. I mean, we have a smart thermostat in this house, but it's quite an old house. It has one or two drafts. And it depends where you put the smart thermostat as to what reading it's going to generate, right? So it sits in the corner of the lounge most of the time. If somebody picks it up and moves it, then the entire system gets thrown. And we end up in this kind of hybrid world of trusting the automation sometimes and not for other things and overriding it. I think that feels like society as a whole at the minute. I have, you know, automation systems in a car which help me, but I don't trust on them 100% of the time.
Gopal: Yeah. And I think what you've just hinted at is the fact that, you know, these autonomous systems are not deployed in isolation. They're deployed in a context where you do have humans maybe interacting with them, with certain understandings of what the autonomous system is trying to do for them, but also with some controls of what it can do for them. And maybe not just one human, maybe multiple humans and at the back of that autonomous systems, you don't know, it's not clearly visible. What is the data that it's using? What other systems is it talking to to deliver you a service or an experience? So, yeah, it is really tricky to work out what exactly will engender trust in a certain context if you try and design a product for everything, right? So, it's not easy to do that. And that's why, you know, we're doing lots of research in that space.
Sean: I think the other thing that sort of springs to mind, and I'm reluctant to say the words, I'm going to say them just this once, Alexa, Siri, Google, all these different home, you know, smart speakers or assistants on phones and iPads and things. It's not clear how the decision making is happening, is it? You know, you ask for, I don't know, it might be the answer to a question, or it might be for the thermostat to be turned up, or any one of a number of things and then this robotic voice comes back and gives you a response. And we don't know what's actually happened in between. So, there are sorts of, you know, even more automation systems pervading our day-to-day lives now.
Gopal: Yeah, and the challenge we find is that these systems are so opaque, it's very unclear what is, how they're making decisions for us or advising us, that people tend to have different sorts of maybe mental models. So, they have a model, they create a model of what it's actually doing in the background. And what we find most of the time is that people expect it to be more intelligent than it is. They expect it to know their preferences, they expect it to take data from different sorts of sensors in the environment, etc, etc. So, there's an over-expectation of these capabilities that sometimes is unmet. And when it is unmet, that's when things go wrong and people start distrusting the system.
[00:30:08]
Sean: So, I think that brings us nicely around to the fact that we're here to talk about the TAS Hub. Is this why we need a TAS Hub? I mean, what sorts of things do you do or will you be doing with the Trustworthy Autonomous Systems Hub?
Gopal: Right. So, TAS Hub, the UKRI Trustworthy Autonomous Systems Hub, as it should be called, is a programme funded by UKRI. So, the UK Research and Innovation. UK Research and Innovation is the main body that allocates funding for large research projects and small research projects as well. And it decided to fund this programme, and it's called the TAS programme, the Trustworthy Autonomous Systems program, as one of its strategic priorities fund projects.
So, it's one of these programmes that's deemed to be of national importance, really important for the country to get right, with really serious impacts on a global level. The TAS Hub sits within that TAS programme, and is meant to coordinate the programme with a number of other research projects.
So, the TAS Hub organises a number of events, a number of activities, coordination activities that helps us bring together the whole community, not just those specific research projects, but the whole UK community, research community, around Trustworthy Autonomous Systems research. So, we are also going to carry out a bunch of research projects within the Hub, and we're going to try and address issues that arise when you design these Trustworthy Autonomous Systems from different angles.
So, for example, we might want to look at the governance element, we might want to look at the verifiability of these systems, we might want to look at the functionality of these systems, or the security, the cyber security elements that arise when you build this system. So, these nodes are going to help us dive deep into those research questions. We call them research nodes, not research projects, but these research nodes will work with the Hub, and the Hub will also have its own research projects that will help us sort of address the gaps in that programme, but also bring in other partners from outside that programme, because we do realise Autonomous Systems research, or Trustworthy Autonomous Systems research, is not just being carried out in this programme, there's lots of research projects across the UK and internationally that need to be brought together, and that's what the Hub aims to do.
So, bring them together, making sure that the research is developed in collaboration with industry, with a large number of industrial partners, government, key stakeholders, the public. And we've done our best, at least in the initial phase, to bring together over 60 partners, which is quite a lot for a research project, and we look to double that number of partners. So, I'm talking here about industrial partners who have an interest in building Autonomous Systems, supplying services that will drive these Autonomous Systems, or service those Autonomous Systems, or ensure those Autonomous Systems. So, lots of different sorts of people involved, even policymakers in that set of partners.
Sean: Is there an aim to analyse systems, or is there an aim perhaps to get towards a position where maybe there's like a certificate, or kind of like a qualification, you know, this has been certified as Trustworthy? Is that the aim?
Gopal: That's a really good question. I mean, I know a lot of people who would want something lined up to have a way of, you know, a kite mark for Autonomous Systems, saying this system is verified and is trustworthy. Now, you can, and one of the goals of this hub is to define those best practices that will help us achieve this level of trustworthiness, to say, these systems are trustworthy by design. Now, there's the aspect of technology itself is trustworthy by design, but as I said earlier, you know, these Autonomous Systems tend to be deployed in certain contexts with a certain set of users, and in a regulatory environment, or social context that determines whether you trust it or not, in a particular circumstances that you're going to use it.
So, the technology may be trustworthy, may be verifiable, may be fully functional, and it has been tested to death. But does it actually work when you have lots of humans making decisions around it, or based on what it says? Can they work with it? Does the system actually comply with regulation in the UK and abroad? So, we need to look at the international context as well. Can we export these products? What does it take to export these products to other environments where they may be deployed alongside humans and other systems? So, it's a really tricky one to get right.
Sean: But it's the complication here and the potential different contexts that perhaps mark this as being something special, because if we take the simplistic automation we have in our houses, the washing machine is a really good example. I'm quite happy to turn a washing machine on and go out to work and know that when I come back at the end of my work day, there won't be water all over the house. It won't have electrocuted the postman by applying electricity to water that's flooding out the house. It does what it does in a very specific way and a very specific context. But it works pretty well, right? You know, a washing machine does what you want it to do. But then, what's the difference now with these newer systems?
Gopal: So, a washing machine is a good example of an automated system. So, it has a very specific set of rules and it's very predictable and it's a very controlled environment, which is why you trust it. You know, you can put your clothes in there, close the door and you know what it's going to do and you can control it perfectly, right? Things go wrong when maybe something really, really strange happens, right? There's a power surge or something that's beyond the control of that system.
But an autonomous system is quite different. An autonomous system, you would expect to do certain things autonomously without you having any input in it and it's not clear what it's going to base its decision on at the time when it has to make decisions without you in the loop. So, you tell it, you know, I would like to go on a holiday next week, right? And trusting it to decide on where you should go, how you should get there, at what time you should leave your house, at what time you should come back. You know, it's very difficult to trust a system that would do that for you, right? Because it doesn't really know your preferences in this case.
You would have to tell it all your preferences, which is what you tend to do on those websites where you say, I want to go after 8am, I want to come back by this time on that day, I don't want to travel more than 12 hours, etc, etc. But sort of modelling all these preferences, so extracting all these preferences from humans, understanding what their constraints are, is very difficult for machines and therefore we need to work on ways to make this easier for the humans to understand what, well, for the humans to translate what they want to the machines and for the machines to explain what they've understood from the humans in order to act on it in the right way, in a predictable way.
Sean: So what sorts of plans do you have for the TAS Hub going forward and who's involved in it?
Gopal: Right, so the TAS Hub is led by the University of Southampton, so I direct the TAS Hub with my colleagues from the University of Nottingham and King's College London. So Derek Macaulay at Nottingham and Luke Mora at King's College London lead their groups. So we have come together as a group of really diverse, you know, a diverse set of academics. And my intention when we started building the team was to really have a diversity of views, diversity in many, many ways. Because I truly think that if you have to build a lot of systems that is going to be acceptable, that are going to be acceptable by different sorts of users, by different parts of society, you need to have that diversity of thinking, a diversity of backgrounds, diversity of disciplines, disciplinary perspectives on the questions.
So we started from that point and said, let's build a team that will represent that and does have an interest in that area. And then looked at who else do we bring on board to help us achieve this and also help us engage with the public and government? So we went out and looked at broad range of stakeholders, you know, from healthcare to government to policymakers, regulators, the learned societies, the Royal Academy of Arts, Royal Academy of Engineering, et cetera, et cetera. So and industry, obviously. All right. So the big players in the autonomous systems sector.
We brought them together. We got their feedback on how we should construct the research programme, how much flexibility there should be in the programme, in terms of both research and advocacy and engagement. So we have three key programmes within the hub. We have a research programme itself, which looks at addressing the fundamental research questions and doing that in a responsive way. So as the needs arises, we have a mechanism now to generate a new research project based on what the research community is seeing as a major issue to address. Also, as the other research projects in the task programme arise, we will be reacting to that.
We have an advocacy and engagement programme, which looks at taking all these nice research results and translating that and publicising that to a really wide audience, but also bringing in those partners, new partners and engaging with the public to get their views on how or what sorts of questions we should be addressing in the programme.
[00:40:06]
And one last sort of the key, I think for me in the programme was the skills programme, which I thought, which is actually not typical of these sorts of research programmes. But the skills programme for me is how we ensure the sustainability of these sorts of research projects, is making sure that we translate what we learn into people that will actually go out and build those trustworthy systems. Because what I find is in the communities that we are, if we have to build those trustworthy autonomous systems, we need to make sure that we have a trained workforce, that does understand the sort of disciplinary perspectives you need to take to address the notion of trust.
You can't just look at it from a pure engineering side or from a pure legal side or from a pure social sciences perspective, you need to bring all these views together and training the next generation of researchers to think in that way is what I'd like to achieve as part of this programme. And that will help us then ensure we have a sustainable pipeline of those, in terms of a workforce, in terms of upskilling people in industry as well, having this workforce that's ready to build the next generation of autonomous systems that will be trustworthy and being able to adapt as we go along. So ensuring that we are more responsive to the demands from society and the economy.
Sean: Those are great goals to have. What would concern me slightly is that I think some people don't take these things as seriously as perhaps they should. And just to put what I'm saying there in context, over on Computerphile, I've done a number of videos about AI safety, and this raging debate about some people saying, well, the robots will uprise and blah, blah, and the opposite side of it, well, no way, that's years away, don't worry about it. What difference do you think this will make?
Gopal: The big questions arise, I mean, those that the public tend to latch on very quickly are those to do with killer robots, for example. Will we allow killer robots in our defence systems? Or how do we then work against adversaries that might be using them? How do we lead the way in doing this? Do we let this be led by researchers? Do we let this be led by policymakers? Who will decide on this? And I think that's the kind of question that we want to address in the programme, to bring to the fore those difficult questions that matter to people in their daily lives and matter to their ethics, but also understanding the balance you need to strike between what needs to be achieved in terms of running services, running a defence system, for example, or running a viable economy, and the trade-offs you're making in terms of other sort of delegation of control that results from you letting autonomous systems do things for you.
So where is the trade-off? Maybe there's an efficiency trade-off against control over these systems. I think surfacing those questions is what we hope to do in the programme, making sure people are aware of it, researchers are aware of public perception of their research, and then that helps us guide, or have an informed debate at least as to what should be the research questions we address and how we go about addressing them.
Sean: Because, yeah, exactly as you say, the public would worry about killer robots and Skynet and all these sort of science fiction things, but it's not a million miles away to say, okay, there are drone strikes being carried out by remote control, and yet in other stories we read about cars driving themselves, swarm robots managing their own. It doesn't take much to put those two together, so it'd be great to think that particularly on this podcast we'll be asking the experts about those things.
Gopal: Yeah, and as you rightly say, I mean, the public perception tends to be largely shaped by what the media puts out there, and they tend to focus on those very high-profile negative events typically. And that's what tends to harm research and innovation. It's public perception that may be wrongly guided sometimes. For example, autonomous vehicles are really, really safe in many cases, or in many controlled environments at least, but it is in different levels of autonomy, for example, do allow us to drive or fly more safely than otherwise.
For example, landing a plane on a ship is really, really hard without an autonomous system helping you do that. But we don't dwell too much on that part. We tend to look at, oh, there was a car crash, someone got killed. It's really sad, but that's maybe one of those chance events that should not have happened either because maybe a sensor failed, maybe a human didn't pay attention, etc. but it was one out of so many millions of accidents that humans tend to be involved with without any autonomous system.
So there are systems that are really helpful, and we should be focusing on those, but I'm trying to understand where the failures might be, what would happen when failures happen, who will be accountable for this? Who will be liable for this? And that sort of starts becoming really tricky when you have multiple of these systems involved or you have multiple dependencies between different systems.
Sean: And multiple assumptions, right?
Gopal: Yeah, multiple assumptions, but also interconnected systems. So you have, say you have a transportation system, you have a set of traffic lights and a set of autonomous vehicles on the roads, and sort of conventions as to, in terms of driving on the left, driving on the right from different countries, and you have the sorts of conventions that allow you to orchestrate the system. And suddenly something happens, right? So suddenly there's a-
Sean: A tree falls on the road.
Gopal: A tree falls on the road or a major storm that reduces visibility. Now, in a way that was unpredictable, right? And then humans might react in a certain way, start driving on the wrong side of the road to avoid an obstacle, which is completely unpredictable for maybe an autonomous vehicle, and the autonomous vehicle doesn't know what to do and crashes in the tree, right? You don't know. So things like that might happen. And this sort of connections between those different systems, you have maybe a traffic light system that doesn't recognise what's going on and maybe less cars pass through, and then there's a major congestion and more accidents. So there's interconnected, there are interconnections that result in major, major events, that could happen. I could see that potentially happening just because we could not foresee all the different circumstances in which we could deploy those systems. But that, I think that's the kind of problem we would like to address, right? One of the problems we'd like to address.
Sean: Without being cheeky, do you think anyone will notice? Will government take notice of this? Will there be legislation related to this? What do you think?
Gopa: I surely hope so. I mean, we are doing this podcast. We are trying to push out those questions that we think everyone should be worried about. We're trying to bring on board people from different backgrounds, different industries, sectors, to tell us what problems we should address and find commonality across all these problems. What we really don't want to do is to find a solution for just autonomous vehicles or just healthcare, right? We want to address problems that are cut across lots of sectors, which is why we are bringing in this diversity of views, diversity of different perspectives.
And by doing that, I think we will sort of make sure that we have those connections and do influence what the government is thinking about in terms of regulating or in terms of guiding the development of autonomous systems and exporting autonomous systems. So we do have good links with the Office for AI. We do have ways of engaging with the other major research centres in the UK. And we are looking and we do have partners who are regulators or do advise on government on various aspects of autonomous vehicles.
Sean: And we kind of really cover this probably, but can you think of any other challenges that you'll have? I mean, dissemination should be fairly straightforward because we're talking about it here and hopefully people will listen. Will there be challenges within the research itself or will it be, what do you think they'll be?
Gopal: I think as with any large programme of this sort, you expect to have, as you push for diversity in views and perspectives, you do expect diverging views on certain matters. We do expect that to happen at a research level. So people thinking, this is the research direction we should take, not the one that the hub is taking, et cetera. But I think what we would like to do is to make sure that these differing perspectives, both on the research and on the applications or the policy implications, should be made very clear and open for everyone to evaluate and understand, this is what the research community is saying and there is divergence in the research community. We should try and address maybe at another level, engaging regulators and policy makers.
[00:50:12]
And trying to come up with one answer to every question is going to be hard, I do accept that. And my role is to try and help sort of have an informed debate, drive the research in a direction that hopefully brings everyone on board from different perspectives, working with all these large research projects that have just been funded, they've just been announced last week. So we're looking forward to working with them and with all these other major research centres in the UK, to really work together to drive or influence policy, influence the regulators, influence industry and really give a boost to industry, lead the way, help them lead the way in this space, build some of the first most trustworthy autonomous systems that could be built in the UK or abroad.
Sean: And how, this is a horrible question, I'm sorry to ask it, how will you measure success in that respect?
Gopal: A typical question we get asked for any programme of this sort, but success for me is when the programme is due to last four years. Ideally, I think we would have solved all the problems in four years' time, but I don't think that will happen.
Sean: Never say never.
Gopal: But we do have some measures of success we'd like to achieve, like influencing government policy with respect to how these systems are regulated or the kind of measures that can be put in place to ensure that these sorts of trustworthy systems or autonomous systems are trusted by everyone, at least the majority, if not also those that are marginalised in society, ensuring that these systems are beneficial to everyone and specifically to industry, so ensuring the adoption by industry.
So I'd like to see some early examples at the end of this programme of how we've supported the development of frameworks, of autonomous systems that industry finds safe to deploy at scale. We'd like to see these systems maybe being certified, having some way of certifying them. And one example, maybe having, finally having some of these systems, these drones being allowed to fly in civilian airspace. So, seeing that happen in the next four years would be great, if we can influence that in one way or another.
But also looking at the other end in terms of systems that are deployed in our daily lives that rely on our data to make suggestions to us on the web, that take our data to optimise our healthcare plans, that incentivise us to exercise more, etc. How can we make sure that these systems are doing the right thing for us? Seeing some good examples of that at the end of this project would be great. But also creating this community, creating a community around this whole topic of trustworthy autonomous systems, and a collaborative community that does acknowledge the differences in perspectives that people might have and go beyond their comfort zone to address the challenges.
Sean: Gopal, thank you very much for joining us today and we look forward to hearing more from you in future episodes.
Gopal: Thank you, Sean. Definitely try to join you again to tell you about the outcomes and the successes we've achieved.
Sean: Okay, so back to our panel now and some really interesting points there and of course, you guys are all part of this Trustworthy Autonomous Systems Hub. So it's not news to you what Gopal's been talking about. But say, for instance, he mentioned the Nest thermostat there. So AI trying to become individualised and people turning it off sometimes. Is there an invasion of privacy here? Paurav, what do you think about that?
Paurav: It is quite interesting, you know, from when you look at it as in the AI is trying to predict our behaviours, but we don't like that. So is it an issue of kind of losing sense of that control? Or is it that we are so random in our approach and our behaviours that whatever that AI is going to do is not going to fit in? The reason is, is that because as individuals, we want to, in a way, I don't sit every time in the same sofa the same way. I don't use, I don't watch, I don't put the remote in the same place every time. That is what machines do. I do it every time differently. And then there is this joy of finding the remote control in the house, where it is and those debates. And that is what makes us human.
And in some sense, when you think about these automation examples that are making our lives easier, initially, they may sound very exciting. But at the same time, they also put a challenge to us that we are not automatons. And so there has to be this balance we will have to find somewhere.
Joel: The thing that to me, why the Nest thermostat is a failure is because it is completely unaware of the social ways in which we do negotiate something mundane, even as heating and heating our home. And if you are thinking about it yourselves, and for those of you, I think the Nest kind of works, it sort of works for one person, you know, but we don't generally tend to live just on our own. I mean, and even then it has its problems. But the point is that, you know, even something as mundane as heating is socially negotiated. And, you know, the people we live with, they have different needs when it comes to, you know, feeling comfortable and having a comfortable temperature.
And so it's actually quite normal in my household that my partner, she will turn the thermostat up and you know, whereas I'm probably quite comfortable already. And then, you know, it's just, and it's things that we take for granted, but it's actually something that the AI cannot seem to cope with very well at all.
Sean: There's even more things. I mean, this is a complex system, isn't it? And it might just be a fashion choice that day, you decide to put a jumper on and suddenly later on that day, you're too warm, right? So that can be as something as simplistic as that. Christine, I can see you're waving your hand. You're desperate to say something about it. Go on?
Christine: So I think coming back to what both Paurav and Joel said, I think there's the concept of uncertainty here. And we as humans are ultimately extremely good at handling predictions under uncertainty and there's a vast amount of research that is actually going into that. How can machines incorporate uncertainty and still make reliable decisions? And this does incorporate, for example, that multiple humans live in a dynamic system and that due to these dynamics between people, between their preferences and the changes in preferences, that the AI needs to be able to predict that. So there is work going into this to exactly tackle that concept and that problem of uncertainty.
Sean: And I think you could argue the case that it's just more complicated than perhaps the implementers of that specific device realise. Maybe we all need our own thermostats and we all need our own, you know, fingerprint access to the thermostat so that it can work out who needs what temperature and where and when. But leaving that to one side for a moment, because everybody has their feelings about how warm a room should be, go in to any office with air conditioning to see that battle playing out. What is it to mean to be trustworthy by design? What does that mean? Can we ever really trust something, something new anyway?
Paurav: Yeah, it is, Sean, it is so difficult for us to trust something new. Else then there is trustworthiness into it. Because trust by nature gets built over a period of time. It is not an instantaneous phenomenon. And so, how do we create trustworthiness by design, is one of the questions which companies corporations have been asking.
So for example, Apple launches iPhone, if you remember in 2007, at that point in time, the way how Steve Jobs makes that presentation, but he carries a bundle of such innovations with him since 2001, since the advent of iTunes and iPods and all those things that allowed them to make that happen. But if you remember in 2001, seven years before Nokia came up with this whole idea of smartphone, it had it the whole thing ready. But there was no trust around that, that it was an engineering company, it was not a consumer company.
And so while you may build a better designed product, it is not necessary that the better designed product would win. What is better trusted, that is what would win. And I think that is something what companies need to think about when they are building these algorithms. If they keep thinking the mathematics of it, then it is not going to work. They will have to think about the behaviour that is going to attach itself to that mathematics.
[01:00:04]
And I think if that doesn't work, then however great a product is, and we have seen so many failures of those kinds.
Sean: I would also argue that it's also down to the emotion and the kind of the feeling invoked by something, you know, if you desire something, trusting it is sort of second nature, if it's something you want?
Christine: Well, it's not just that systems or people have to earn trust in this particular case. I think specifically with regards to AI, we have been subliminally primed by the entertainment industry for decades, that AI is not to be trusted. There have been so many movies and books that were drawing out this singularity phenomenon, that humans ultimately believe that robotics and AI are not trustworthy. So we don't just need to gain the trust, we need to overcome the distrust that has been ingrained into us by society. So I think that is an extremely difficult problem to tackle. And part of our responsibilities as researchers, academics and practitioners in the area, to actually find mechanisms to engage the public and explain to the public what we actually do and what the benefits are to society and to industry.
Sean: I think you're right, from films like The Terminator, the killer robots kind of idea, you know, be it iRobot or whatever, it has been ingrained. However, just before the iPhone was released, there was a film that came out, which most people may well have seen called Iron Man. Iron Man has a virtual assistant called Jarvis. Jarvis is not a million miles away from kind of what they hope Siri or Alexa or any of those might want to be. And yet, he's seen as sort of he, I’m anthropomorphising, if I can say the word properly, is seen as kind of a benevolent, you know, something everyone thinks is cool, we'd want to have that. So there is definitely some kind of separation there between the killer robot idea and the, hey, this AI is going to do this work for me.
Paurav: One of the examples I would give of a positive phenomenon in terms of how media has made us buy into new technology would be the foldable phone. If you remember the foldable phone, it was, you know, a lot of people bought it because they wanted to feel like that Star Trek's Captain Kirk. The whole point was, you know, I'm opening that phone and now I'm talking and I can put it in my pocket and all those things. So there is this media driven nostalgia that also is built around some of these technologies, which allows us to, you know, buy that trust, in some sense, or predate that trust.
But Christine is absolutely right that as a programme also, as a UKRI funded programme, I think our job as researchers and scientists is to build, you know, a better picture, a more informed picture, rather than just one sided picture.
Sean: I mean, we mentioned in the conversation with Gopal about the idea of a washing machine, just doing what it's supposed to do. But there have been cases where that's not the case. I mean, there was a famous case of the Whirlpool dryer where, I mean, I think that was years ago, but there are still ramifications of that, where it's a faulty device that can cause fire to happen. So, we still continue to trust our washing machines and our dryers and our white goods, though. Is that just habit?
Joel: I think perhaps a lot of it has to do with experience. And of course, we take it, you might say habit, we take it for granted, the washing machine and the dryer, and we don't think of it as a high tech product. And, and also, it's a simple machine. You know, although you might not be able to explain it fully, you get the concept of what it is, is simple, and is intuitive for people. And AI is not. AI is the opposite of that. AI is complicated, and complex. And hence why, with digital as well, I mean, there are so many different layers.
And there are examples of AI intruding into our privacy. And hence, why trust is just so important, and trustworthiness with regards to AI is so important. And what is trustworthy by design is, of course, sort of one of the key research questions for the TAS Hub. And the answer is, there isn't a single answer to that. But there is a context dependent answer. And it depends on the application, the domain and the technologies. So yeah, these are really important questions.
Sean: I think you've really hit upon something there. I mean, the whole point of this is that as a more simplistic piece of automation, you can fathom it, you can see, you know, I put the washing powder in here, a motor turns this, water comes in, hopefully at the end of it, your clothes are slightly cleaner. And you can sort of see where it starts and where it ends. With a lot of these AI devices, you don't know what's gone into it. You don't know what was in the training data that that got the AI to the space to the point it's at now. You don't know what the perhaps Google or Amazon or whoever is controlling it are doing with your data that you know, what's going to happen from that point onwards. There's all sorts of things that are a little bit for want of a better word opaque.
But hopefully with the test hub, we will, and with these podcasts, we'll be able to try and make some sense of it for people and get to a point where people start to understand what's going on and understand how things are trustworthy by design or whether they are trustworthy by design more to the point. So I'd like to say thank you for joining us again today, Christine and Joel and Paurav. And yeah, we'll hope to hear from you again very soon.
Paurav: Thanks for having us, Sean.
Joel: Thank you.
Christine: Thank you very much.
Sean: If you want to get in touch with us here at the Living with AI podcast, you can visit the TAS website at www.tas.ac.uk, where you can also find out more about the Trustworthy Autonomous Systems Hub. The Living with AI podcast is a production of the Trustworthy Autonomous Systems Hub. Audio engineering was by Boardie Limited and it was presented by me, Sean Riley. Subscribe to us wherever you get your podcasts from and we hope to see you again soon.
[01:06:53]